Reporting the Results
Reporting the results is one of the fundamental aspects of clinical research. Accurate results benefit diagnosis, patient outcomes, and drug manufacturing. Nevertheless, measurements and findings are often prone to errors and bias (Bartlett & Frost, 2008).
Various research methods and statistical procedures exist to help experts erase discrepancies and reach “true” values. In the end, the main aim of any clinical trial is accuracy.
Repeatability and Medical Data
Repeatability is a paramount factor that reveals the consistency of any clinical method. In other words, repeatability unveils if the same instrument used in the same subject more than once will lead to the same results (Peat, 2011). Note that the term repeatability was introduced by Bland and Altman, after which various terminology was created in a similar way.
Although terms, such as repeatability, reproducibility, reliability, consistency, and test-retest variability, can be used interchangeably – there are some slight differences. Repeatability, for instance, requires the same location, the same tool, the same observer, and the same subject. Consequently, the repeatability coefficient reveals the precision of a test and the difference between the two repeated test findings over a short period of time. To test repeatability in continuous data – statistics, such as the intraclass correlation coefficient and Levine’s test of equal variance, can be utilized. For categorical data – kappa and proportion in the agreement can support research. Reproducibility, on the other side, refers to the ability to replicate medical studies. In other words, it reveals the agreement between results – obtained from different subjects, via different tools, and at different locations (“What is the difference between repeatability and reproducibility?” 2014).
Data types also matter. As explained above, in the case of continuously distributed measures, measurement error (or standard error of the measurement (SEM)) and intraclass correlation coefficient (ICC) are the two most effective indicators of repeatability (regarding the reliability of a measure). The measurement error reveals any within-subject test-retest variation. Note the measurement error is an absolute estimate of the absolute range in which the true value can be found. On the other hand, the ICC is defined as a relative estimate of repeatability. To be more precise, it reveals any between-subject variance to the total variance for continuous measures. When it comes to interpretation, a high ICC means that only a small proportion of the variance is due to within-subject variance. In fact, ICC close to one means there’s no within-subject variance.
For categorical data, there are also various methods – with kappa being one of the sufficient statistics. Basically, kappa is similar to ICC but applicable to categorical data. Thus, kappa values close to one indicate total agreement (Peat, 2011). Note that repeatability in categorical measurements is also called misclassification error.
Continuous Data and True Values
Medical research is a curious niche full of unexpected outcomes. Variations and errors are quite common. Variations may occur even when the same subject is tested twice via the same tool. Such discrepancies might be a result of various factors: within-observer variation (intra-observer error), between-observer variation (inter-observer error), and within-subject variation (test-retest error), and actual changes in the subject after a certain intervention (responsiveness). To be more precise, variations may occur due to changes: in the observer, the subject, or the equipment.
Consequently, it’s hard to analyze true values. To guarantee accuracy, any good study design should ensure that more than one measurement will be taken from each subject to assess estimate repeatability (Peat, 2011).
Selection Bias and Sample Size
Selection bias affects the repeatability scores. Therefore, studies that have different subject selection criteria cannot be compared. At the same time, estimates of studies with three or four repeated measures cannot be compared to studies with two repeated measures.
Note that estimates of ICC may be higher and estimates of measurement error lower if the inclusion criteria lead to variations. To set an example, usually, ICC will be higher when subjects are selected randomly. Researchers should recruit a sample of a minimum of 30 subjects to guarantee adequate measurements of variance.
Within-subject Variance and Two Paired Measurements
Paired data is vital in research. Paired data should be used to measure within-subject variances. The mean values and the standard deviation (SD) of the differences also must be computed. The measurement error can be later transformed into a 95% range. In fact, these is the so-called limits of agreement – or the 95% certainty that the true value for a subject lies within the calculated range (Peat, 2011).
- Paired t-tests are beneficial in assessing systematic bias between observers.
- A test of equal variance (e.g., Levene’s test of equal variance) can be helpful to assess repeatability in two different groups.
- A plot of the mean value is also crucial in order to assess the difference between various measures for each subject. This is an effective method as usually, the mean-vs-difference plot (or Bland-&-Altman plot) is clearer than any scatter plots.
- Note that Kendall’s correlation can add more valuable insights to the study. Kendall’s tau-b correlation coefficient indicates the strength of association that exists between two variables (“Kendall’s Tau-b using SPSS Statistics”).
Measurement Error and Various Measurements per Subject
Nothing is only black and white in medical research. Often, more than two measures are required per subject. In case there are more than two measurements taken per subject, experts should calculate the variance for each subject and after that, any within-subject variances. Note that such values can be calculated via ANOVA. A mean-vs-standard deviation plot can visualize the results. In addition, Kendall’s coefficient can indicate if there’s any systematic error.
Note that deciding on a reliable measure out of a selection of different measures is a difficult task. In clinical settings, this is a vital process as it may affect patients’ outcomes. Assessing measurement errors is also fundamental. The measurement error indicates the range of normal and abnormal values from the baseline. In fact, these values can reveal either a positive or a negative effect of a treatment (Peat, 2011). Let’s say that previously abnormal values have come close to normal values. One interpretation of this phenomenon can be that a disease has been affected by a treatment in a positive direction.
ICC and Methods to Calculate ICC
The ICC is an essential indicator in order to show the extent to which multiple measures taken from the same subjects are related to each other. This form of correlation is also known as a reliability coefficient. As explained above, a high ICC value means that variances are due to true differences between subjects, while the rest due to measurement errors (within-subject variance).
Unlike other correlation coefficients (Pearson’s correlation, for example), ICC is relatively easy to calculate. There are a few methods that can help experts calculate ICC, along with other sufficient computer programs. The first method employs a one-way analysis of variance – it is used when the difference between observers is fixed. The second method that can be beneficial refers to cases when there are many observers – it is based on a two-way analysis of variance. There is also a third method which is simplified – based on only two measures per subject.
- In fact, P values can be computed from ICC, which eliminates the need for a test of significance. However, note that the Pearson’s correlation coefficient (R) is often used to describe repeatability or agreement, which could lead to false interpretations. To set an example, in cases when there’s a systematic difference (e.g., the second set of measures which is larger than the first), the correlation can be perfect but yet, the repeatability poor.
- A coefficient of variation, which is the within-subject SD divided by the mean of the measures, may be employed. Still, ICC is a more accurate indicator.
- F tests to show if ICC differs from zero can be performed. Generally speaking, an F test is computed after methods, such as ANOVA and regression, to assess if the mean values of two populations differ (“F Statistic / F Value: Simple Definition and Interpretation”).
- Confidence intervals should be calculated as well to support the data analysis.
Measurement Error and ICC
To sum up, measurement error and ICC are two paramount indicators in medical research. Since both give different statistics, they should be reported together.
Note that while the ICC is related to the ratio of the measurement error to the total SD, the measurement error is an absolute value related to the total SD (Peat, 2011).
Repeatability
Repeatability of Categorical Data
The repeatability of categorical data (e.g., the presence of illnesses) collected via surveys and questionnaires is also vital. As explained above, when it comes to categorical data, measurement error is called misclassification error. Note that there are a few requirements that are mandatory: 1) the questions, the mode of administration, and the settings must be identical on each occasion, 2) subjects and observers must be blinded to the results, and 3) the time between the test-retest processes must be appropriate. When it comes to the community, the repeatability values should be established in similar community settings (not extreme subsamples). On top of that, patients who are tested quite often should be excluded as they might have all answers well-rehearsed.
Kappa is the most popular statistic, which can reveal the observed proportion in agreement and the estimated correct classification value. Usually, kappa is beneficial for measuring test-retest repeatability of self-administered surveys and between-observer agreement in interviews. Note that a kappa value of zero reveals the chance agreement, while a value of one is the perfect agreement. In addition, 0.5 shows moderate agreement, above 0.7 – good agreement, above 0.8 – very good agreement. We should mention that the average correct classification rate is an alternative to kappa: it’s higher than the observed proportion in agreement, and it represents the probability of a consistent reply.
Repeatability and Validity
In the end, repeatability and validity go hand in hand. Basically, poor repeatability leads to the poor validity of an instrument and limits the accuracy of results. Thus, many indicators and statistics can be employed. Since causes and outcomes are all interconnected, it’s not recommended to use ICC in isolation – simply because ICC is not a very responsive factor and it is not powerful enough to describe the consistency of an instrument.
We should mention that even a valid instrument may reveal some measurement error. In fact, measurement error has a simple clinical interpretation, which makes it a good statistic to use.
Agreement and Measures
Apart from repeatability, the agreement is another paramount aspect of medical research. The agreement is defined as the extent to which two different methods used to measure a particular variable can be compared or substituted with one another. To set an example, experts should know when measurements taken from the same subject via different tools can be used interchangeably (Peat, 2011). Note that agreement, or comparability of the tests, mainly assesses the criterion and the construct validity of a test. Nevertheless, results can never be identical.
There are numerous statistics that can be explored to measure agreement. There are tables that can guide experts on how to employ several effective methods in various situations. For example, just like with repeatability, measurement error, ICC, and paired tests are among the most powerful statistics for continuous data and units the same. Also, Kappa is the main indicator that can help researchers with the analysis of categorical data. On the other hand, in situations when one measure is continuous, and the other one categorical – The receiver Operating Curve (ROC) curve can be employed (“What is a ROC curve?”).
Continuous Data and Units the Same
As mentioned earlier, different measures rarely give identical results. Even if experts measure weight via two different scales, the figures won’t be exactly the same. Thus, when two measurements have to be used interchangeably or converted from one another, it must be clear how much error there will be after the conversion.
When figures are expressed in the same units, the agreement can be assessed via the measurement error or the mean value of the within-subject variance. Since these measures are calculated within the same group of subjects, methods are similar to the ones for repeatability.
Agreement and Mean-vs-differences Plot
Drawing a mean-vs-differences plot is also an effective method, which can be used along with calculating the 95% of agreement. Note that when two tools do not agree well, this effect might be because they measure different variables or because one of the instruments is unprecise.
Note that when it comes to a poor agreement, consistent bias can be assessed by computing the rank correlation coefficient of a plot. Kendall’s correlation coefficient, for instance, can indicate if the agreement and the size of the measurement are related. If the correlation is high, then there’s a systematic bias that varies with the size of the measurement. In case such a relationship occurs, a regression equation can help experts convert measurements. Usually, a regression equation can help researchers explore the connections between sets of data and predict future events. Note that in linear regression, there’s a perfectly straight line.
95%-of-Agreement and Clinical Differences
Calculating the 95%-of-agreement is also essential. As a matter of fact, Bland and Altman defined the limits of agreement as to the range in which 95% of the difference can be found (Peat, 2011). Note that a measure with poor repeatability will never agree well with another tool.
Variances are a common phenomenon in research. As described above, it’s normal for two instruments to express some differences. However, such instruments can be used interchangeably in practice only when this range of differences is not clinically important. In the end, patients’ well-being is the main goal of science.
Continuous and Categorical Measures
Continuously distributed information (such as blood tests) is often needed in practice as it can predict the presence of a disease. Also, in order to predict the presence or the absence of a condition, experts need cut-off values, which can indicate normal and abnormal results. The ROC curve is the most effective method to obtain such information.
Note that in order to draw a ROC curve, the first step is to calculate the sensitivity and the specificity of the measure – for different cut-off points of the variable. The bigger the area under the curve is, the more effective the test is. Also, if experts want to check if one test can differentiate between two conditions, they can plot two ROC curves on the same graph and compare them (Peat, 2011)
Agreement and Categorical Data
Continuous data is crucial, and so is categorical information. Categorical measurements and the level of agreement between them can reveal the utility of a test. To be more precise, the ability of a test to predict the presence or the absence of disease is paramount in medicine (Peat, 2011). In clinical settings, methods, such as self-reports, observation of clinical symptoms, and diagnostic tests (e.g., X-rays), can help experts classify patients according to a presence of a disease or an absence of a disease (e.g., tuberculosis).
In this case, sensitivity and specificity are two essential statistics as they can be applied to different populations. Sensitivity is defined as the proportion of ill subjects who are correctly diagnosed by a positive test result. Specificity, on the other hand, is the proportion of disease negative patients who are correctly diagnosed by a negative test result. What’s more, these indicators can be compared between different studies, which employ different selection criteria and different testing methods.
Yet, the probability that the measure will reveal the correct diagnosis is the most important aspect, which can be achieved by the positive predictive value (PPV) and the negative predictive value (NPV) of a test (Peat, 2011). Note that PPV and NPV depend on the prevalence of a disease, so they cannot be applied to studies with different levels of prevalence of illness. We should mention that in rare diseases, the PPV will be closer to zero. This effect is because experts cannot be certain if a positive result can actually reveal an existing illness.
Likelihood Ratio and Confidence Intervals
The likelihood ratio is the most effective statistic used to compare different populations and clinical settings. The likelihood ratio is defined as the likelihood that certain findings would be expected in subjects with the disorder of interest, compared to subjects without that disease. This statistic incorporates both sensitivity and specificity and reveals how good a test is (e.g., the higher the value, the more effective the test will be). The likelihood ratio can be used to calculate pre-test and post-test odds, which can provide valuable clinical information (“Likelihood ratios”).
Note that all statistics described above, including the likelihood ratio, reveal a certain degree of error (Peat, 2011). Therefore, their 95% confidence intervals should be calculated.
Continuous and Categorical Measures
Continuously distributed information (such as blood tests) is often needed in practice as it can predict the presence of a disease. Also, in order to predict the presence or the absence of a condition, experts need cut-off values, which can indicate normal and abnormal results. The ROC curve is the most effective method to obtain such information.
Note that in order to draw a ROC curve, the first step is to calculate the sensitivity and the specificity of the measure – for different cut-off points of the variable. The bigger the area under the curve is, the more effective the test is. Also, if experts want to check if one test can differentiate between two conditions, they can plot two ROC curves on the same graph and compare them (Peat, 2011)
Relative Risk, Odds Ratios and Number Needed to Treat
Measures of association are also vital in reporting the results. The relative risk (RR), odds ratio (OR), and number needed to treat (NNT) can reveal if there’s a risk of disease in patients exposed to a certain factor or a treatment (Peat, 2011).
Relative Risk and Associations
For prospective cohort and cross-sectional studies, relative risk is the most effective statistic to present associations between exposures and outcomes. Note that exposures can be related to personal choice (e.g., drinking) or occupational and environmental risks (e.g., pollutants).
Consequently, relative risk is calculated by comparing the prevalence of an illness in the exposed and non-exposed groups. Note that relative risk depends on the time needed for an illness to develop.
Odds Ratios and Case-control Studies
Odds ratios are another essential characteristic. It can be employed in case-control studies in which is impossible to calculate the relative risk due to the sampling method. The odds ratios represent the odds of exposure in both cases and controls. Note that in such studies, the prevalence of a disease does not represent the prevalence in the community. Nevertheless, statistical procedures like multiple regression allow experts to apply odds ratios to cohort and cross-sectional studies. When confounders occur, experts may employ adjusted odds ratios – or when confounders have been removed from the association between risks and outcomes.
Here we should mention that both statistics, relative risk, and odds ratios, are hard to interpret. Due to their complicity, when it comes to 95% confidence intervals, it is recommended to use a statistical program. Note that sometimes both statistics may differ when in practice, the absolute effect of the exposure is the same. On the other hand, they may be statistically the same when in reality, the absolute effect is actually different. Such differences may mislead experts and lead to type I or type II errors. Thus, odds ratios are recommended only for case-control studies and rare diseases (Peat, 2011).
Number Needed to Treat and Practice
Analysis of medical data might be tricky. While odds ratios are difficult to explore, the number needed to treat is a statistic, which is extremely beneficial in practice. The number needed to treat is defined as the estimate of patients who need to undergo treatment for one additional subject to benefit (Peat, 2011). In other words, this is the number of subjects that experts need to treat to prevent one bad outcome (“Number Needed to Treat”).
Note that the number needed to treat represents the clinical effect of a new treatment. It should balance the costs of treatment and possible negative effects for the controls. The number needed to treat can be calculated from meta-analyses and findings from different studies. There are also formulas to convert odds ratios to a number needed to treat. To set an example, if the number needed to treat for a new intervention equals four (NNT=4), that means that experts need to treat four people to prevent one bad outcome. It’s not surprising that it’s better to save one life for four patients, instead of one life for ten patients (Peat, 2011).
Matched and Paired Studies
There are different study designs, and in fact, case-control studies are a popular method. Basically, in case-control studies, the matching process of cases and controls is based on confounders. Note that removing any possible effects in the study design is a more effective technique than analyzing confounders at a later stage of the study. Also, we should mention that analyses may employ paired data and methods such as conditional logistic regression.
Another crucial characteristic of matched and paired analyses is the fact that the number of units is the number of matches or pairs – not the total number of subjects. The effect of the pairing also has an effect on associations, confidence intervals, and odds ratios (Peat, 2011). Usually, matched analyses reduce bias and improve the precision of confidence intervals.
More than One Control and Precision
In some situations, more than one control can be used for each case. This technique improves precision. Note that the number of controls is considered for an effective sample size.
Let’s say we have 30 cases and 60 controls; the number of matched pairs will be 60.
Logistic Regression and t-tests
When there are non-matched data, experts can use logistic regression and calculate adjusted odds ratios. Note that logistic regression is used when more than one independent variable determines an outcome. The outcome, on the other hand, is a dichotomous variable (e.g., data can be coded as 1 (e.g., pregnant) and 0 (non-pregnant)).
In addition, the differences in outcomes between cases and controls can be calculated via a paired t-test. This type of testing assesses if the mean difference between two sets of observations (each subject is measured twice) is zero. Multiple regression is also beneficial.
Exact Methods
Data analysis should be based on accurate statistical methods. It’s unethical to violate information to obtain statistically significant results, which are not clinically important. In cases when the prevalence of a disease is not common, exact methods can be employed. Exact methods can also be used for small sample sizes and small groups in stratified analyses (Peat, 2011).
The differences between normal methods and exact methods are:
- Normal methods rely on big samples
- Normal methods utilize normally distributed data
- The variable of interest in normal methods is not rare
- Exact methods require more complex statistical packages
Rate of Occurrence and Prevalence Statistics
To explore rare diseases, experts may investigate the incidence or the rate of occurrence of a disease. The incidence reveals any new cases within a defined group and a defined period of time. Usually, since some diseases are rare, this number is expressed per 10,000 or 100,000 subjects (e.g., children less than five years old).
Prevalence, on the other hand, is estimated from the total number of cases – regarding a specific illness, a given population, and a clear time period (e.g., 10% of the population in 2017). Prevalence is affected by factors, such as the number of deaths (Peat, 2011).
Confidence Intervals and Chi-square
Confidence intervals are also paramount inexact methods. As explained above, the 95% confidence intervals are defined as the range in which experts are 95% confident that the true value lies. Usually, the exact confidence intervals are based on the Poisson distribution. This type of distribution helps researchers investigate the probability of events in a certain period.
When we need to explore the association between a disease and other factors, such as age, we can employ a contingency table. This helps the cross-classification of data into a table in order to visualize the total number of participants in all subgroups. Note that chi-square is a statistic that is of great help. Usually, Pearson’s chi-square is used for large samples (more than 1000, with five subjects in each cell of the table). Continuity adjusted chi-square, on the other hand, can be adjusted for samples under 1000. Last but not the least, Fischer’s chi-square is applicable when there are less than five subjects in each case. Chi-square tests are also used to analyze subsets of information (Peat, 2011).
Reporting the results is a complicated process. Data types and statistical procedures may challenge scientific findings. Nevertheless, experts should always aim for accuracy – with the sole purpose to improve patients’ well-being.
References
Bartlett, J., & Frost, C. (2008). Reliability, repeatability and reproducibility: analysis of measurement errors in continuous variables. Ultrasound in Obstetrics and Gynecology, 4, p. 466-75.
Kendall’s Tau-b using SPSS Statistics. Retrieved from https://statistics.laerd.com/spss-tutorials/kendalls-tau-b-using-spss-statistics.php
Likelihood Ratios. Retrieved from https://www.cebm.net/2014/02/likelihood-ratios/
Number needed to treat. Retrieved from https://www.cebm.net/2014/03/number-needed-to-treat-nnt/
Peat, J. (2011). Reporting the Results. Health Science Research: SAGE Publications, Ltd.
Statistic / F Value: Simple Definition and Interpretation. Retrieved from http://www.statisticshowto.com/probability-and-statistics/f-statistic-value-test/
What is a ROC curve? Retrieved from https://www.theanalysisfactor.com/what-is-an-roc-curve/
What is the Difference Between Repeatability and Reproducibility? (2014, June 27). Retrieved from https://www.labmate-online.com/news/news-and-views/5/breaking-news/what-is-the-difference-between-repeatability-and-reproducibility/30638
See more of Our Posts
DIGITAL HEALTH CATEGORY
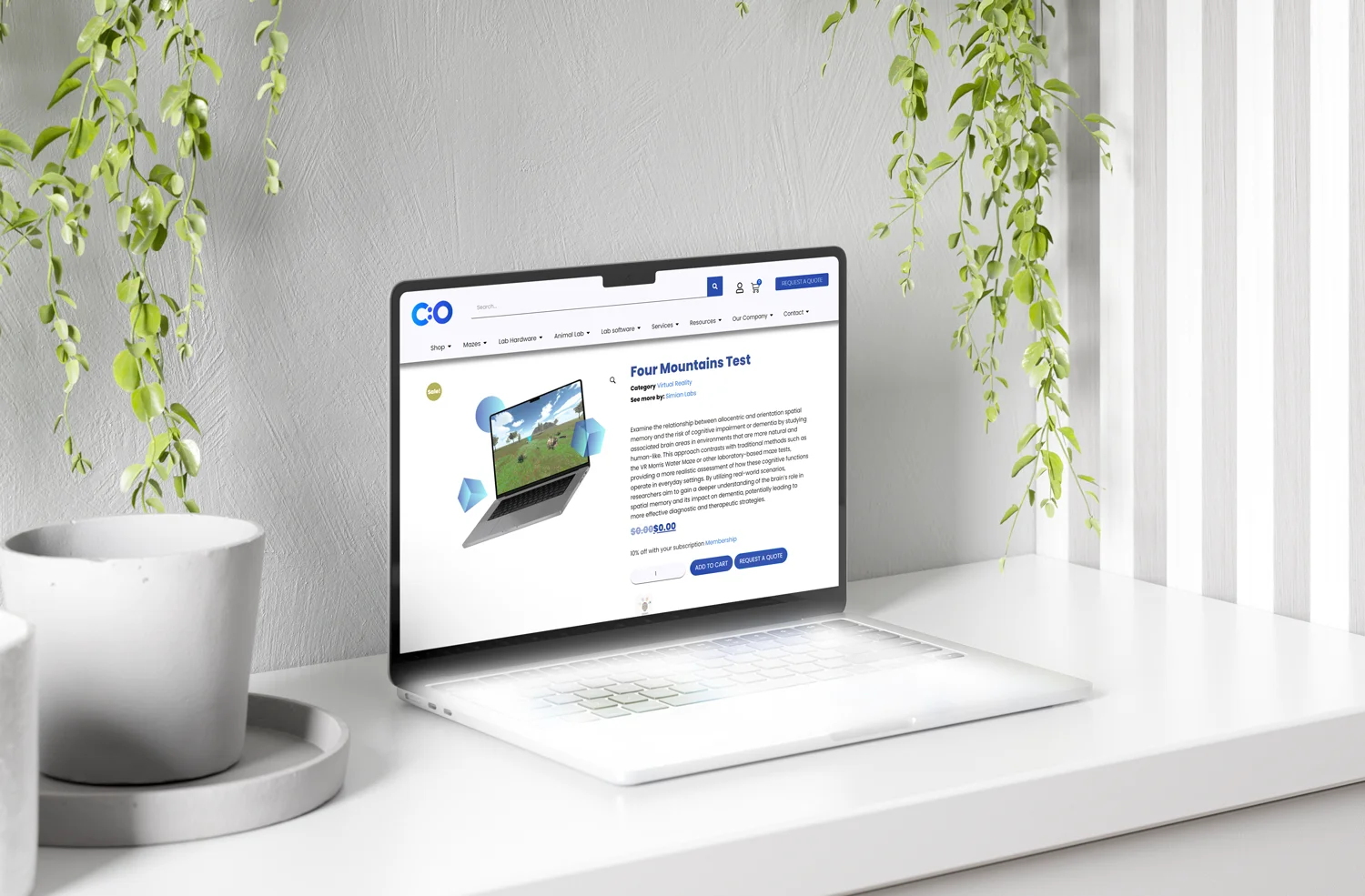
History and Early Tests Leading to the Four Mountains Test
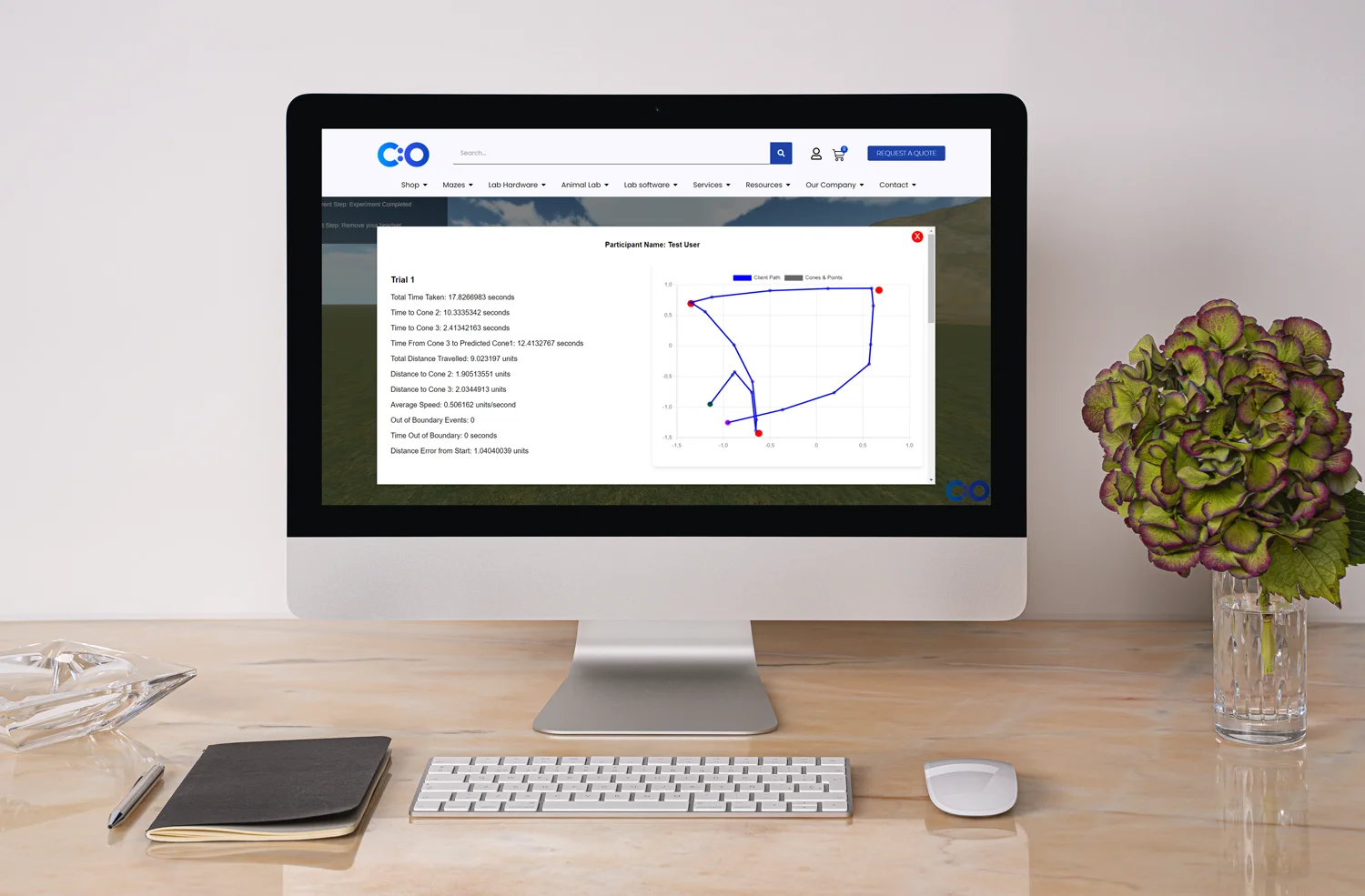
Analyzing Data from the Four Mountains Test
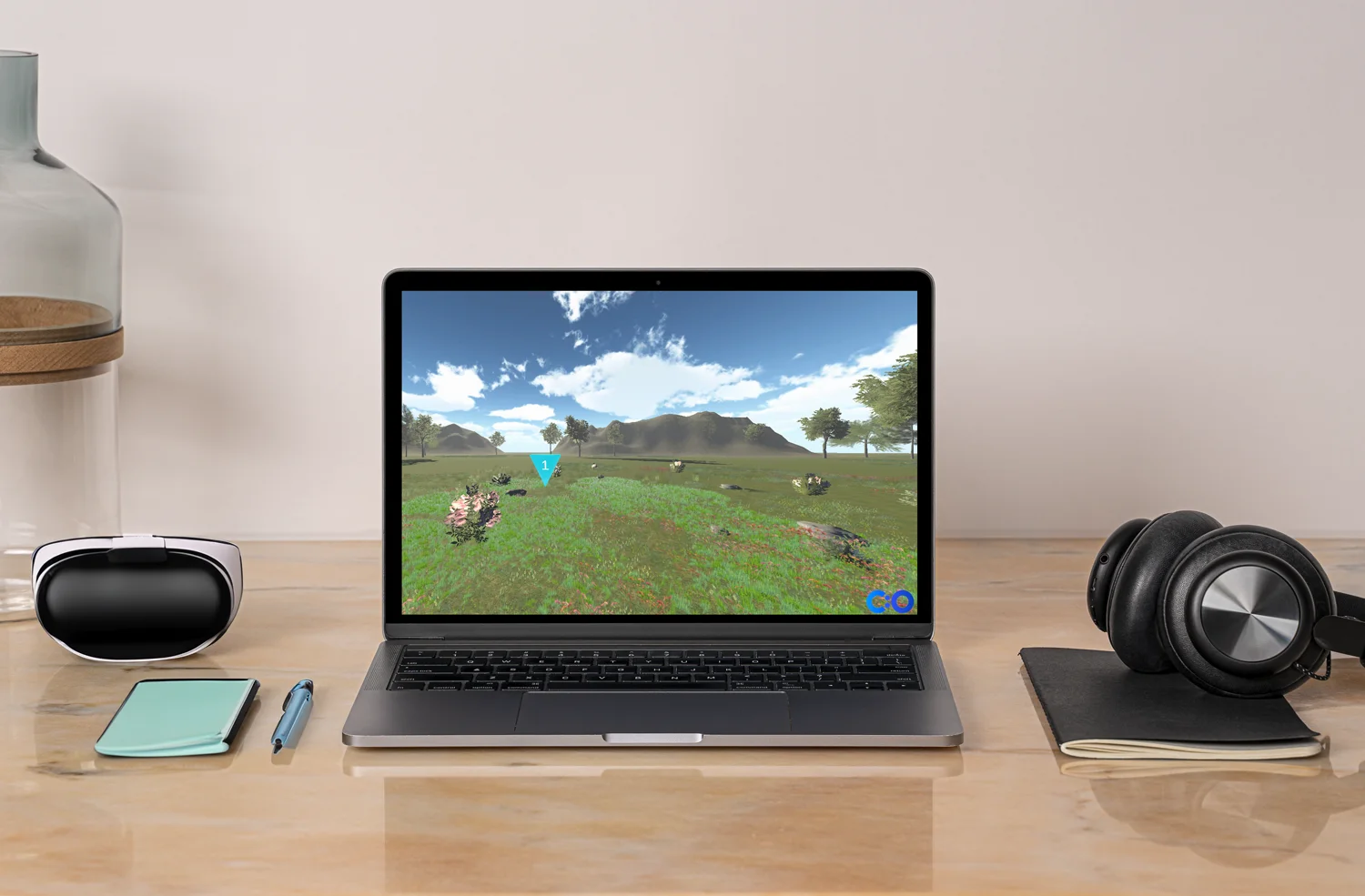
Understanding the Four Mountains Test: A Scientific Exploration
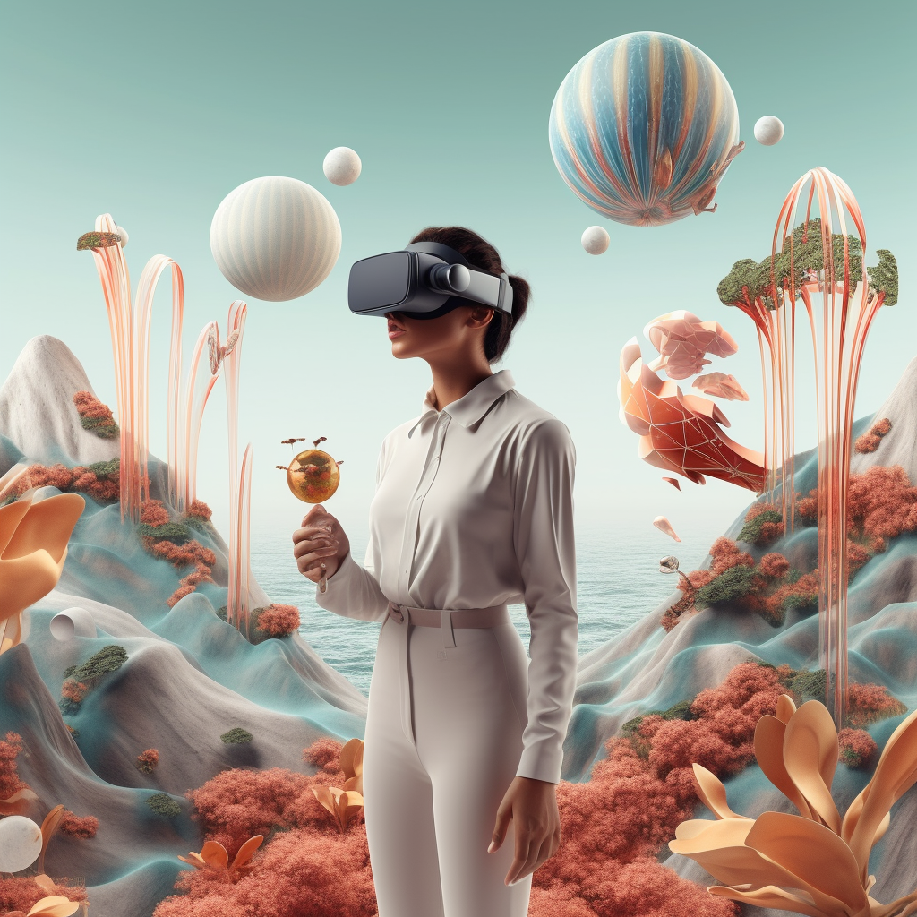
Virtual Reality Science: Exploring the Boundaries of Scientific Discovery
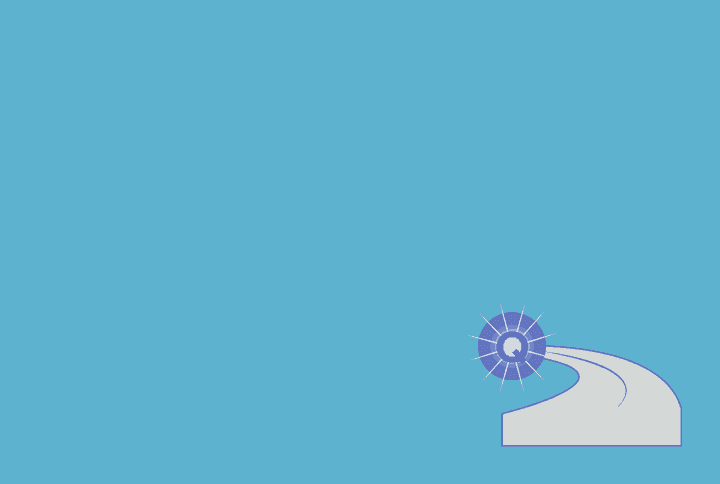
FHIR Claim Response
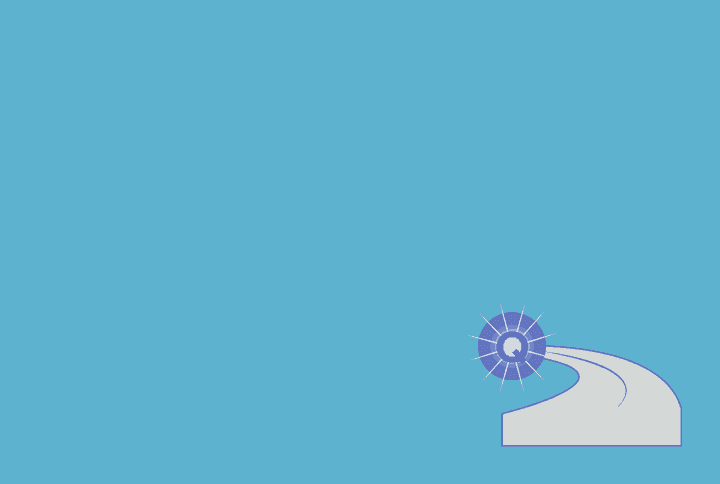
Corsi Block Test
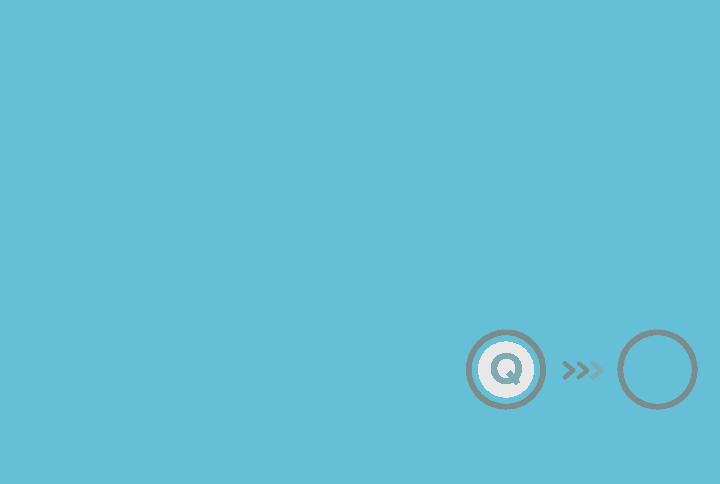
9-Hole Peg

What Adoption of Remote Patient Monitoring will mean for Healthcare Delivery

Digital Health Research: A Guide
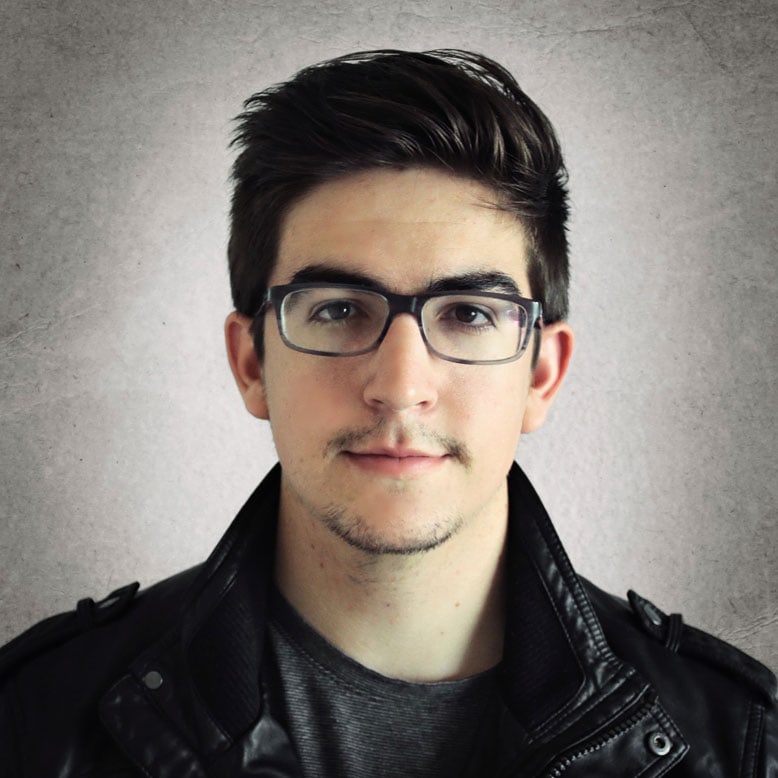
Alex Wormuth: Data Saves Lives
Never miss our posts!
Author:
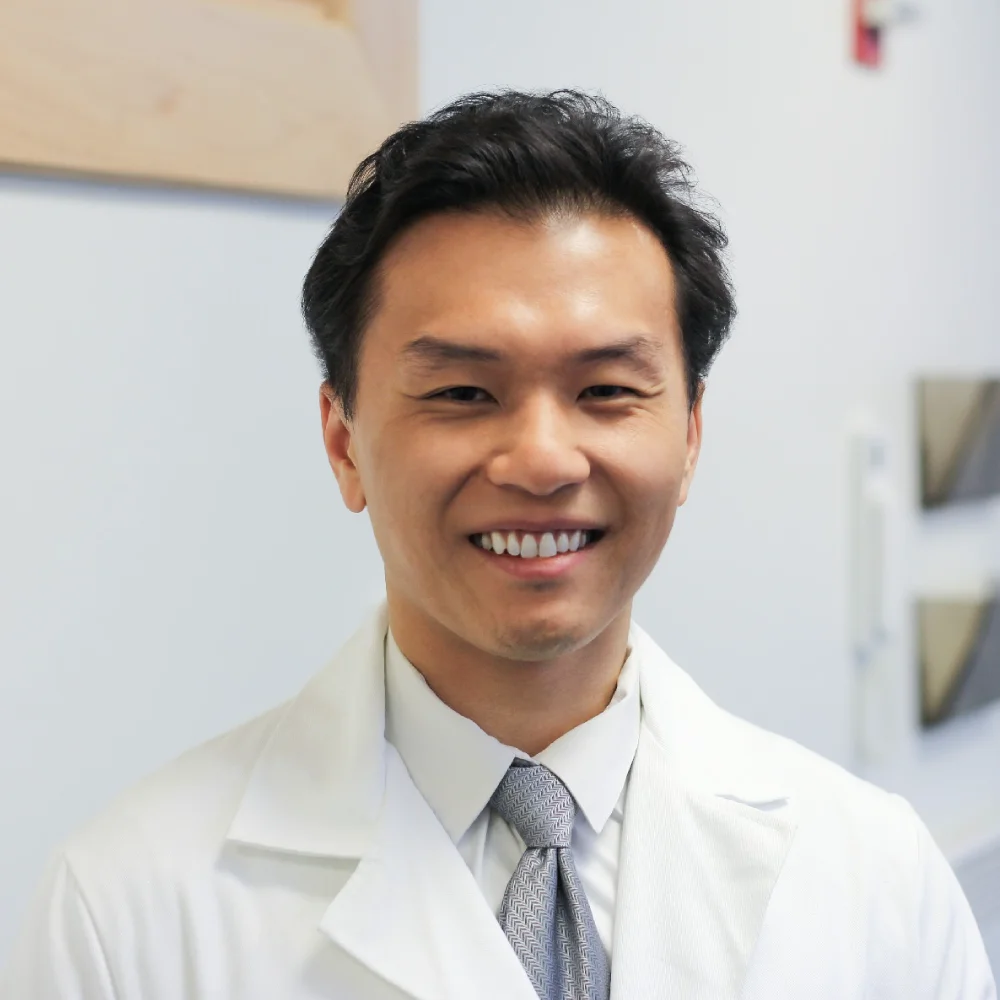
Shuhan He. MD
Shuhan He, MD is a dual-board certified physician with expertise in Emergency Medicine and Clinical Informatics. Dr. He works at the Laboratory of Computer Science, clinically in the Department of Emergency Medicine and Instructor of Medicine at Harvard Medical School. He serves as the Program Director of Healthcare Data Analytics at MGHIHP. Dr. He has interests at the intersection of acute care and computer science, utilizing algorithmic approaches to systems with a focus on large actionable data and Bayesian interpretation. Committed to making a positive impact in the field of healthcare through the use of cutting-edge technology and data analytics.