Study Designs: Basics of Research
Study designs are paramount in research. From generating a scientific question and testing a hypothesis to publishing a scientific paper, research teams need to plan and develop a relevant study design, which can suit their experimental goals and financial strategies.
Designing a study is an exciting process. Since experiments in both physical and life sciences aim to establish a causal relationship between variables, each study design should be adapted to the basics of research accordingly. Of course, the first step for every team is to formulate a clear research problem, based on in-depth literature research. Then, research hypotheses, roles, and experimental methods, such as data collection and analysis, should be established clearly. Most of all, when designing and conducting a study, researchers need to follow safety and ethical regulations – always aiming for patients’ well-being.
Designing a study, however, is also a challenging task. Financial demands, time delays, and ethical issues seem to sabotage medical research. What’s more, when it comes to medicine, safety and efficacy become crucial.
Therefore, when choosing a study design, experts need to be familiar with all basics, specifications, limitations, and benefits of all types of study designs.
Study Designs: Choose the Right One
Study designs are paramount in research. From generating a scientific question and testing a hypothesis to publishing a scientific paper, research teams need to plan and develop a relevant study design, which can suit their experimental goals and financial strategies.
Designing a study is an exciting process. Since experiments in both physical and life sciences aim to establish a causal relationship between variables, each study design should be adapted to the basics of research accordingly. Of course, the first step for every team is to formulate a clear research problem, based on in-depth literature research. Then, research hypotheses, roles, and experimental methods, such as data collection and analysis, should be established clearly. Most of all, when designing and conducting a study, researchers need to follow safety and ethical regulations – always aiming for patients’ well-being.
Designing a study, however, is also a challenging task. Financial demands, time delays, and ethical issues seem to sabotage medical research. What’s more, when it comes to medicine, safety and efficacy become crucial.
Therefore, when choosing a study design, experts need to be familiar with all basics, specifications, limitations, and benefits of all types of study designs.
Before experts proceed with choosing a design and creating a study within medical settings, there are a few research steps and terms that need to be clarified (Peat, 2011)
Descriptive Vs. Experimental Studies:
While each study design can be described as the procedure of employing research methods to recruit participants, administer interventions, and collect data, descriptive and experimental studies should be differentiated.
Descriptive studies refer to situations in which vital research factors, such as gender or age, cannot be modified. In other words, descriptive studies identify behaviors but can’t make predictions or reveal causality. Thus, when employing descriptive studies, researchers become observers and analysts. Descriptive studies simply provide a snapshot of the current situation (Stangor, 2011).
Another powerful method is a correlation. A correlational study can describe, discover or predict the way research variables are connected – without manipulating them.
On the other hand, experimental studies give researchers the unique opportunity to modify and control variables to test research hypotheses. For instance, when implementing experimental studies, experts can modify various aspects of research – let’s say via administering a novice drug treatment across various groups. As a result, experimental studies are perceived as more powerful when compared to descriptive studies, and consequently, they are widely used in medical research.
Qualitative Vs. Quantitate Studies:
Another important difference between studies is their qualitative or quantitative nature (Moffatt, 2006). While quantitative methods rely on conventional data collection and statistical procedures, qualitative studies involve open questions and other in-depth methods, such as interviews with patients.
As mentioned above, quantitative studies help researchers collect data and transform it into statistics. Such studies are well structured and can include large samples. As a result, they are widely used in research and medical practice.
On the other hand, qualitative studies can help researchers gain insights and generate testable hypotheses. They can be used parallel to quantitative studies to explore patients’ feelings, attitudes towards a new treatment, and personal tactics to cope with a disease.
Phases of Clinical Trials
Also, when it comes to medical trials, there are four different phases according to which studies can be divided (Peat, 2011). As a matter of fact, some rare studies may include Phase 0. Note that this differentiation comes from the fact that patients’ safety always comes first.
Phase I studies aim to test the safety aspect of all new medical interventions. Usually, new interventions and drugs are tested on animals and a small group of volunteers. Still, ethics and safety are the main objectives. Note that since animals are used to develop and test new medical treatments, the ethics of animal research may challenge conventional methods and medicine (Festing & Wilkinson, 2007).
Phase II studies include a larger sample of participants and aim to test the efficacy of a treatment. Note that efficacy can be defined as the effect of a treatment in ideal conditions – whether an intervention does more good than harm under ideal options. Here placebo can also be employed. This can be the case only if the employment of a placebo won’t breach ethical and safety issues. In other words, researchers cannot deny a life-saving treatment just to test a placebo control group.
Phases III studies can be conducted after safety and efficacy have been established. Such studies include randomized trials and multicenter studies. In fact, they aim to test the effectiveness and equivalence of the treatment. In other words, Phase III studies aim to test the effect of the treatment in less ideal circumstances and routine practices, which is the so-called effectiveness, and the extent to which the new treatment relates to an existing treatment, which is the so-called equivalence.
Last but not the least, Phase IV studies can also be implemented. They are crucial in medical research as they aim to test any possible rare side effects of a new treatment or intervention in the long-term.
Study Designs: Types
Now, when it’s clear that each study design can only benefit practice, let’s explore some of the most common types of study designs used in medical research. There’s no right and wrong as there’s no such a thing as the one-size-fits-all approach in medicine.
Meta-analysis
Meta-analysis is a study design, which is a powerful research method. It’s based on data collected from different studies. Meta-analysis can also be described as quantitative and epidemiological study design. In fact, a rigorous meta-analysis is a great approach to evidence-based medicine.
Since this design involves the profound analysis of previous studies, a meta-analysis may have the potential to reveal hidden insights and relationships, such as possible health risks related to a new treatment and medical interventions. Not surprisingly, this particular aspect is one of the main advantages of meta-analyses (Peat, 2011).
However, as meta-analysis requires the use of complex data and quantitative review of the literature, failing to identify all existing studies in literature may lead to wrong conclusions and sabotage research.
Systematic Review
The systematic review is another common type of research used in the assessment of literature and studies, which addresses a particular health-related issue (Cochrane Handbook for Systematic Reviews of Interventions).
Systematic reviews can be used to summarize the results of all available medical studies and controlled trials. In fact, they can provide vital information about the effectiveness of an intervention. Note that systematic reviews can include meta-analyses.
Nevertheless, one of the main disadvantages is that, as mentioned above, failing to collect and research complicated data may lead to erroneous conclusions and crash research.
Randomized Controlled Trials
Randomized controlled trials are among the most rigorous study designs implemented in clinical research. These trials are defined as controlled experiments that give researchers the chance to test various interventions in random order. Randomized trials can also be classified as quantitative and comparative studies because research outcomes can be measured and compared easily.
To be more precise – as the name suggests- randomized controlled trials are studies in which subjects are allocated to conditions and interventions at random (Peat, 2011). The method of allocating by chance alone helps experts exclude interfering factors, such as selection bias. Note that double binding can also be used to avoid bias and other prognostics factors.
Since the subjects are allocated to receive different interventions (new treatment, existing treatment, placebo, or no intervention) at random, randomized controlled trials reveal an outstanding advantage when compared to other study designs. By implementing randomized controlled trials in research, experts have the unique opportunity to check efficacy, effectiveness, equivalence, and causation. On top of that, vital cofounders and factors, such as environmental exposure, can also be compared and assessed. What’s more, each subject has an equal chance to be in a control group or treatment, which can help generalizability. Yet, consent is mandatory.
However, one of the main limitations consists in the fact that this study design requires large samples. The size of the sample matters to measure adverse effects and other long-term outcomes that may occur with time. For this purpose, though, Phase IV surveys can be used. In fact, the size of the sample is also crucial in determining statistical significance in medical conditions that can have only minimal improvement (for instance, chronic conditions like asthma).
An example of a double-blinded randomized trial is a study conducted by Idris and colleagues (1993). The team aimed to test the equivalence of treatment with a bronchodilator via a nebulizer and the metered-dose inhaler for acute asthma. Patients (N=35) who were attending two emergence asthma departments were included. 20 patients received treatment by a nebulizer and 15 – by an inhaler and placebo via a nebulizer. The study didn’t find any significant difference between groups but noted quicker effects of treatment delivered via inhalers. However, there are various limitations in this study. One of the biggest disadvantages is the small sample and the lack of measurement of outcomes, such as the number of discharges.
Placebo-Controlled Trials
Placebo-controlled trials are an important aspect of randomized controlled trials. Placebo groups are often beneficial in medicine. Still, a placebo can only be used when researchers are uncertain about which treatment is the best for patients (Peat, 2011). Often Phase II studies implement placebo groups, and only with small samples in the short-term.
Nevertheless, the use of a placebo control group can raise some ethical issues; for instance, in cases when subjects are denied the best treatment. Therefore, as explained above, the use of a placebo can only be justified when experts are uncertain which treatment is the best. Unfortunately, there were cases of patients who had been withheld from receiving a beneficial treatment and left in a placebo group for long periods.
An example of a placebo trial, for instance, was a study on asthma that aimed to test the use of leukotriene receptor agonists against placebo (Ferreira, 2001). Note that the next logical step, if effective and efficacy were revealed, would be to conduct Phase III and Phase IV studies to compare effectiveness.
Pragmatic Trials
Since clinical trials can be divided into two categories – pragmatic and explanatory trials, we should focus on pragmatic trials (Tosh et al., 2011). Pragmatic trials are used to assess the effectiveness of medical interventions in real-life practices. On the other hand, explanatory trials test the effectiveness of an intervention under optimal conditions. To be more precise, pragmatic trials are a variation of the randomized controlled trial designs which means that participants are allocated at random.
A major advantage of this design is the fact that pragmatic designs measure effectiveness, not efficacy, and aim to test if a new treatment is better than current treatment. Complex methods, survival rates, and other factors that are patient-oriented are also a focus of research. These studies employ ‘intention-to-treat’ methods to improve patients’ physical and emotional well-being. In order words, pragmatic trials help experts choose the best treatment available to help patients.
However, let’s not forget that blinding is not always possible in practice, which can be one of the biggest limitations of all pragmatic studies. Often, to avoid drop-out rates, researchers can organize a run-in phase before randomization and give time for the subjects to decide if they want to participate or not. Identifying non-complaint subjects may affect the generalizability of results, but smaller samples may prevent delays and dropouts.
An example of a pragmatic study is the randomized trial designed by Laidlaw and colleagues (1998) to test the effectiveness of second eye cataract surgery, following the first eye. Out of 807 subjects, 208 consented to participate. Questions regarding one’s visual difficulties along with visual tests were given to participants. The team proved that second surgery might lead to some improvements. Still, subjective information should be interpreted carefully.
Cross-over Trials
Cross-over trials are also a powerful study design. Just like with randomized trials, they involve the procedure of researchers allocating subjects randomly. However, the difference here is that subjects receive two or more treatments one after another; so, the randomization refers to the order patients receive each treatment (Peat, 2011). In other words, this design is a repeated measurements design, and subjects cross over treatments during the study. In comparison, in parallel studies subjects stay on the same treatment during the whole trial.
Having one subject going through multiple treatments is, in fact, one of the biggest pluses of this study design. Thus, cross-over trials are powerful methods with each subject serving as their own control group. This also means that big samples are not a must and even fewer subjects can be used to obtain meaningful results. Note that cross-over trials work the best for chronic diseases where there’s no absolute treatment for patients but some slight improvements in life.
Nevertheless, there are some disadvantages to this design. If a subject drops out after the second treatment, their results need to be excluded from all analyses. Also, there might be a ‘carry-over’ effect; this means that subjects whose health has improved after the first treatment carry this improvement in the second phase. To resolve the ‘carry-over’ effect, experts can organize a ‘wash-out’ period, something that should be considered during the design stage of the study. Of course, lack of treatment during the wash-out period should not have a negative effect on patients’ health.
Ellaway and colleagues (1999) organized a cross-over trial to measure the L-carnitine effectiveness in improving functional limitations in girls with Rett syndrome. 35 girls were randomized and received either: 1) eight weeks of L-carnitine, washout of four weeks, and eight weeks of placebo; or 2) eight weeks of placebo, washout of four weeks, and eight weeks of L-carnitine. Note that -carnitine proved to be effective in patients with classical Rett syndrome.
Zelen’s Design
Zelen’s design or randomized consent design is also a randomized design, but randomization occurs before consent. In fact, consent is obtained only from the subjects allocated to an experimental treatment. When it comes to Zelen’s designs, treatment should be invasive and illnesses severe. Note that statistician Marvin Zelen was the first person to suggest this idea, so the design was named after him.
When randomization to a placebo or a standard treatment is not acceptable, Zelen’s designs can be employed. In fact, this helps experts deal with low rates of consent and low recruitment rates in invasive treatments. Subjects who don’t agree with taking part in an experimental treatment still receive the standard treatment, and their results are analyzed as if they were part of the experimental groups (Peat, 2011).
One of the disadvantages of this study design, though, is that due to its nature, experts cannot always control all confounders. On top of that, Zelen’s designs raise some questions: isn’t it unethical to randomize patients before consent? Yet, such designs are a great research method that can be employed in screening.
Comprehensive Cohort Studies
The comprehensive cohort study or prospective cohort study with a randomized sub-cohort is a study design that includes subjects who agree to be randomized and subjects who choose (and insist on) a treatment.
Comprehensive cohort studies are extremely useful in cases when subjects may refuse randomization; for instance, when it comes to radiotherapy or surgery for treating cancer. In contrast with Zelen’s studies, comprehensive cohort studies respect patients’ freedom and choice, which is highly beneficial.
Just like other trials with a preference group, comprehensive cohort studies do not provide definitive information about the effectiveness and the efficacy of a treatment, but supplemental results. Therefore, a further independent randomized controlled trial will be needed to provide clear evidence and to answer generalizability goals.
In fact, one of the biggest limitations of the comprehensive cohort study is related to generalizability: the randomized groups should be big enough to lead to meaningful results (Peat, 2011).
An example of a comprehensive cohort study with patient preference groups is the study conducted by Agertoft and Pedersen (1994). The research team aimed to measure the effects of long-term treatment with inhaled corticosteroids in kids with asthma. The parents of 216 children consented to take inhaled corticosteroids for 3-6 years, and the parents of 62 children preferred their kids to stay on cromoglicate. Results showed that experimental treatment resulted in reduced hospital admission rates.
Non-randomized Clinical Trials
In non-randomized clinical trials, the researcher or the participants themselves decide the group of allocation. Non-randomized clinical trials are used mainly to answer questions and provide additional evidence that randomized trials can’t.
Yet, the information can have greater generalizability, and selection bias is not an issue. In contrast, randomized trials have strict inclusion criteria, which may affect the generalizability of results. On top of that, while subjects in non-randomized trials are more willing to enroll, participants in the randomized trial who are receiving standard treatment after randomization may drop out due to dissatisfaction (Peat, 2011). As a result, selection bias may affect the generalizability of results in randomized trials.
Nevertheless, in non-randomized studies, allocation bias is still an issue. Cofounders may not be controlled and lead the team to wrong interpretations. What’s more, when subjects choose a treatment in which they believe, this positive attitude may affect the study results.
However, do not forget that the information obtained during a non-randomized trial is only supplemental. Therefore, one of the best options – in case the sample is big enough and allows it – is to include randomized and preference groups and compare them separately.
Open Trials
Open trials or open-label trials are study designs in which both researchers and subjects know which treatment the subject receives.
This study design is very useful in Phase I trials. It’s important for patients to be aware that the treatment is experimental, and the study may answer questions only about efficacy (Peat, 2011). Transparency is a must.
However, open trials have a major disadvantage due to their transparent nature: subjects’ optimistic attitudes may lead to bias in a positive direction.
Case-control Studies
Case-control studies are also very informative. In case-control studies, people suffering from a disease are compared to healthy people. Information about exposure factors is then collected and compared (Peat, 2011). Note that there are matched case-control studies in which vital personal characteristics between cases and controls match.
Case-control studies are not as costly as cohort studies, and on top of that, they provide answers quicker than cohort studies. Another advantage is that by implementing a case-control design, researchers have the unique chance to employ various ways to select cases and controls. In fact, the most appropriate way to choose controls is to recruit people who would have been selected as cases – if they had the particular health issue of interest. Also, a good approach is to select controls from the same study base or population of the cases. Note that each case can have more than one control, which will be compared.
As this study design often relies on retrospective data about exposure and other risks, recall of past events can lead to uncontrolled confounding and bias and type I error (false-positive result). Therefore, the results that case-control studies provide are more beneficial in generating hypotheses and ideas, instead of testing actual causation. In other words, case-control studies are great in the initial stages of research, and in fact, all generated ideas can be consequently developed in other studies.
For instance, results from one particular case-control study conducted by Parker and colleagues (1998) can be extremely beneficial in practice. The team tested if there was a connection between neonatal intra-muscular administration of vitamin K and childhood cancer, based on self-reported exposure. Consequently, based on results, another study was conducted using medical records and assessing the national cancer registry for more objective information.
Nested Case-control Studies
Nested case-control studies are studies that can be conducted within a cohort study. It is a powerful study design as cases and controls are chosen from one single cohort. In other words, this approach reduces costs and time when compared to the full cohort approach. Nested case-control studies are extremely beneficial for studying factors, such as biological precursors of an illness.
One of the main advantages of this design is that when a case appears in the cohort, controls who were at risk at the time can be selected. This tactic gives researchers control over any confounding effects that may be established. As mentioned above, nested case-control studies reduce the costs of following up a whole cohort.
Nevertheless, bias and other errors may occur. Therefore, a larger number of controls can be enrolled for each case to improve the statistical efficiency of the study.
An example is the following study. Badawi and colleagues (1998) designed a case-control study to measure risk factors for newborn encephalopathy. The sample base was selected from all births in the metropolitan area of Western Australia between June 1993 and September 1995. The team proved that many causes of encephalopathy start before birth.
Matched Case-control studies
Matched case-control studies are another powerful design. They are used when cases and controls are selected based on matching personal characteristics (like gender). This way vital confounders, such as gender and age, can be eliminated, and as a result, researchers can focus on other exposure factors related to a certain disease. This approach is highly important to determine prognostic factors, which may go unnoticed in the random selection and small studies.
Some factors are strong cofounders in many diseases. However, when the matching variables occur within friends and family, selection bias may occur. Therefore, a better option is to choose matched controls based on other characteristics; for instance, the next person on an electoral registry. Note that an effective sample size counts the number of pairs, not the number of subjects. Also, another disadvantage of this study design is related to generalizability: generalizability may be reduced if controls match cases more than the general population. On top of that, the case has to be excluded from the analysis if control cannot be found. Last but not the least, remember that over-matching is also harmful: it can lead to bias and shift the results towards the null. Over-matching can occur when experts select cases and controls based on many cofounders: age, gender, social background, etc.
Salonen and colleagues (1998) conducted a matched case-control study to test the link between iron stores and non-insulin-dependent diabetes among men (N=1038). Matching characteristics were age, time of examination, smoking, exercising, weight, etc. The follow-up period was four years. The statistical power of the study was increased by having two controls for each case.
Studies with Historical Cohorts
Studies with historical cohorts compare people given the new treatment and people given the standard treatment in the past. These studies can be used for convenience, and they definitely benefit practice.
Nevertheless, studies with historical cohorts are often subject to bias. Lack of factors, such as control of cofounders, changes in inclusion criteria for other treatments, and outcome variables, may interfere with findings.
Halken and colleagues (2004) conducted an interesting study with historical cohorts. Their research aims were to investigate the effectiveness of allergenic avoidance in the prevention of allergic symptoms in infancy. The intervention consisted of avoidance of exposure to tobacco, pets, etc. The infants were followed up within 18 months. Outcomes like dermatitis and wheeze were measured.
Cross-sectional Studies
Cross-sectional studies are another wonderful way to obtain initial information about diseases within a community, including mortality rates and associations between exposure and risks. Data is collected at a single point in time, which provides a snapshot of a disease. A large random sample is obtained from the general population of interest.
One of the biggest advantages is that such studies provide vital information about the burden of disease within the community (Peat, 2011). On top of that, serial cross-sectional studies are a preferred method for measuring health status, health-related behavior, and chronic diseases in a population. Since information is collected via questionnaires, serial cross-sectional studies are cheaper and quicker than cohort studies, for example.
However, a big disadvantage is that, since exposures and outcomes are measured at the same time, researchers can’t determine which comes first. Note that a response over 80% is needed to avoid bias and improve generalizability. In general, smaller samples with high response rates are more useful than larger samples with low response rates. Note that the poor validity of the methods can also affect the results.
An example of a cross-sectional study is the study designed by Kauer and colleagues. The research team aimed to measure the variation and prevalence of asthma symptoms in children (12-14). Various schools in England, Wales, and Scotland were selected.
Ecological Studies
Ecological studies are also a popular study design. They provide statistics on population groups, such as schools and countries. They are extremely useful in describing populations and differences between different groups (such as schools). Research is often based on various factors, such as the prevalence of a disease or mortality rates.
However, ecological studies rely on data that is collected not directly from subjects, but a national census, for example. Therefore, these studies can’t show vital differences between individuals. On top of that, ecological studies have one big disadvantage: since there’s no control of confounders, ecological studies provide a weak study design to assess causation (Peat, 2011).
For instance, Douglas and colleagues (1998) conducted an ecological study investigating if sudden infant death syndrome (SIDS) has a seasonal pattern. They concluded that there’s a seasonal peak in winter, especially in kids under five months
Qualitative Studies
Qualitative studies, as explained earlier, can be described as descriptive studies that use in-depth interviews to collect vital information. Such studies give additional meaning to research, provide a better understanding, and may complete qualitative studies.
Qualitative studies provide information about the patients and carers, which is tailored and patient-oriented. It is extremely helpful in providing additional information and for revealing patients’ needs and feelings towards healthcare. Since patients can share their thoughts on treatments (in studies of effectiveness), qualitative studies can reveal the acceptability of treatments and interventions (Peat, 2011).
Nevertheless, there are some disadvantages. For instance, the generalization of results is not known.
An example of a qualitative study is the work of Butler and colleagues (1998). They conducted semi-structured interviews to assess the effectiveness of opportunistic anti-smoking campaigns, which is an overused practice employed by general practitioners.
Case Reports
Last but not the least, case reports and case series are also vital. They are descriptive designs and are very educational tools. They can be described as a record of interesting cases. As such, case reports provide information about one patient or a small group of patients, and case series – about larger groups of patients.
Unfortunately, hypotheses can’t be tested, and associations can’t be explored. What’s more, the number of cases is often limited.
A curious case report was provided by Ellaway and colleagues (1998). They examined the association of protein-losing enteropathy with cobalamin C-defect in a male infant.
Study Designs: Plan in Advance
Pilot studies
Pilot studies, as mentioned earlier, are vital in research. Before conducting any study, a pilot study or a preliminary investigation will be needed.
For instance, pilot studies, also called feasibility studies, are beneficial in testing recruiting methods, practicality, sample size, and other specifications of each study design (Peat, 2011). Such studies will prevent changes in protocol and eliminate errors.
Remember that it’s better to conduct a pilot study, instead of changing the design and failing to conduct the actual study in later stages of research.
Methodological Studies
Methodological studies, just like pilot studies, are also paramount in research. They are studies that aim to test if research methods are accurate and repeatable (Peat, 2011).
Methodological studies can help experts test if the employed research instrument can be interchanged with another one. Therefore, studies that assess repeatability and agreement of a research instrument are crucial. In the end, repeatability and avoiding bias are important aspects of research.
Study Designs: Conclusion
To sum up, designing a study is a challenging process. There are many factors that should be considered: from funding to recruiting subjects; research can be tricky. Unfortunately, without a good study design, even the most impressive research idea can fail.
Also, it’s important to understand that each type of study design has various benefits and limitations, and there’s no one-size-fits-all approach in medicine.
In the end, designing a study is worth it: all research studies pile on the existing scientific knowledge with the sole aim to improve patients’ well-being.
References
- Agertoft, L., & Pedersen, S. (1994). Effects of long-term treatment with an inhaled corticosteroid on growth and pulmonary function in asthmatic children. Respiratory Medicine, Volume 88, Issue 5, pages 373-381.
- Badawi, N., Kurinczuk, J., Keogh, J., Alessandri, L., O’Sullivan, F., Burton, P., Pemberton, P., Stanley, F. (1998). Antepartum risk factors for newborn encephalopathy: the Western Australian case-control study. BMJ, 317(7172), p.1549-1553.
- Butler, C., Pill, R. & Stott, N. (1998). Qualitative study of patients’ perceptions of doctors’ advice to quit smoking: implications for opportunistic health promotion. BMJ, 316(7148), p.1878-1881.
- Cochrane Handbook for Systematic Reviews of Interventions. Retrieved from www.cochrane.org/resources/handbook/index.htm
- Douglas, A., Helms, P., & Jolliffe, I. (1998). Seasonality of sudden infant death syndrome (SIDS) by age at death. Acta Paediatrica, 87(10), p.1033-1038.
- Ellaway, C., Christpdoulou, J., Kamath, R., Carpenter, K., & Wilcken, B. (1998). The association of protein-losing enteropathy with cobalamin C defect. Journal of Inherited Metabolic Disease, 21(1), p.17-22.
- Ellaway, C., Williams, K., Leonard, H., Higgins, G., Wilcken, B., & Christodoulou, J. (1999). Rett syndrome: randomized controlled trial of L-carnitine. Journal of Child Neurology, 14(3), p.162-167.
- Ferreira, M., Santos, A., Pregal, A., Michelena, T., Alonson, E., de Sousa, A., Pereira, E., & Palma-Carlos, A. (2001). Leukotriene receptor antagonists (Montelukast) in the treatment of asthma crisis: preliminary results of a double-blind placebo controlled randomized study. Allergy and Immunology, 22(8), p. 315-318.
- Festing, S., & Wilkinson, R. (2007). The ethics of animal research. Talking Point on the use of animals in scientific research. EMBO, 8(6), p.526–530.
- Halken, S. (2004). Prevention of allergic disease in childhood: clinical and epidemiological aspects of primary and secondary allergy prevention. Pediatric Allergy and Immunology, 16:4-5, p.9-32.
- Idris, A., McDermott, M., Raucci, J., Morrabel, A., McGorray, S., & Hendeles, L., (1993). Emergency department treatment of severe asthma. Metered-dose inhaler plus holding chamber is equivalent in effectiveness to nebulizer. Chest, 103(3), p.665-672.
- Laidlaw, D. Harrad, R., Hopper, C., Whiteaker, A., Donovan, J., Brooker, S., Marsh, G., Peters, T., Sparrow J., & Frankle, S. (1998). Randomised trial of effectiveness of second eye cataract surgery. Lancet, 352(9132), p.925-929.
- Martinez, F., Wright, A., Taussig, L., Holberg, C., Halonen, M., & Morgan W. (1995). Asthma and wheezing in the first six years of life. The New England Journal of Medicine, 332(3), p.133-138.
- Moffatt, S., White, M., Mackintosh, J., & Howel, D. (2006). Using quantitative and qualitative data in health services research – what happens when mixed method findings conflict? BMC Health Services Research, 6:28.
- Parker, L., Cole, M., Craft, A., & Hey, E. (1998). Neonatal vitamin K administration and childhood cancer in the north of England: retrospective case-control study. BMJ, 316(7126), p.189–193.
- Peat, J. (2011). Planning the study. Sage Research Methods.
- Salonen, J., Tuomainen, T., Nyyssönen, K., Lakka, H., & Punnonen, K. (1998). Relation between iron stores and non-insulin dependent diabetes in men: case-control study. MJ; 317(7160), p. 727–730.
- Stangor, C. (2011). Research methods for the behavioral sciences (4th ed.). Mountain View, CA: Cengage.
- Tosh, G., Soares-Weiser, K., & Adams, C. (2011). Pragmatic vs explanatory trials: the Pragmascope tool to help measure differences in protocols of mental health randomized controlled trials. Dialogues in Clinical Neuroscience, 13(2), p. 209–215.
See more of Our Posts
DIGITAL HEALTH CATEGORY
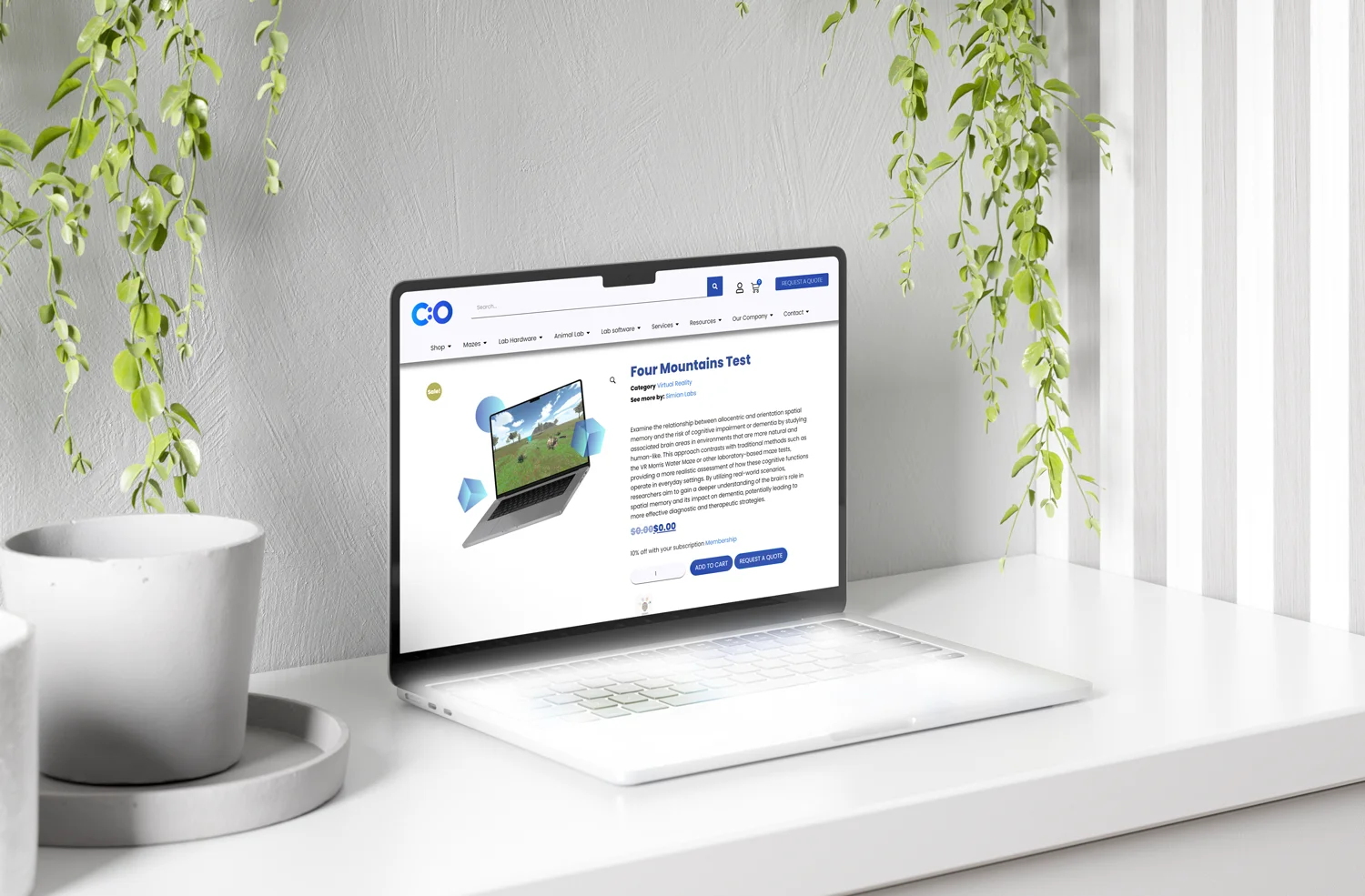
History and Early Tests Leading to the Four Mountains Test
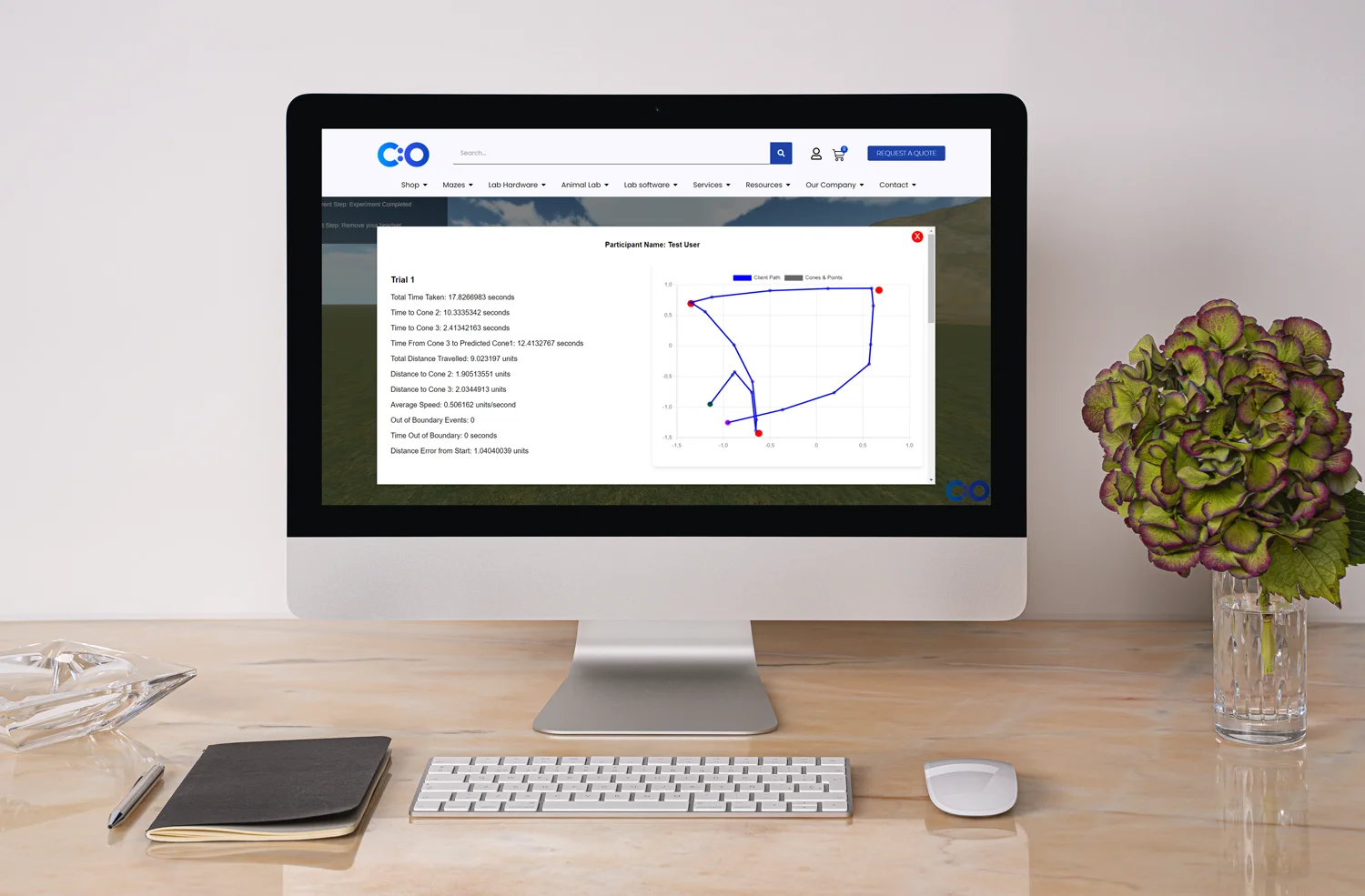
Analyzing Data from the Four Mountains Test
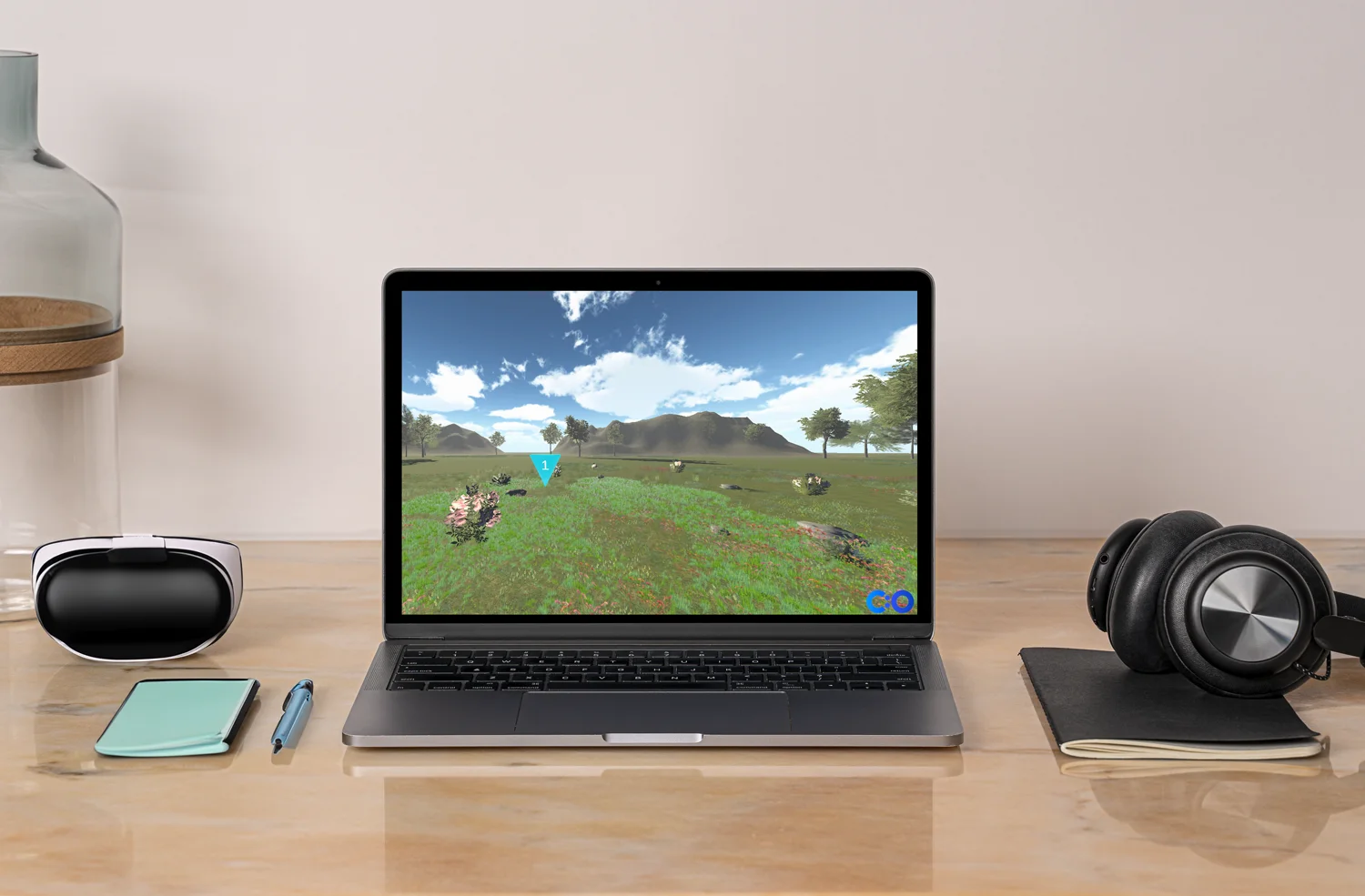
Understanding the Four Mountains Test: A Scientific Exploration
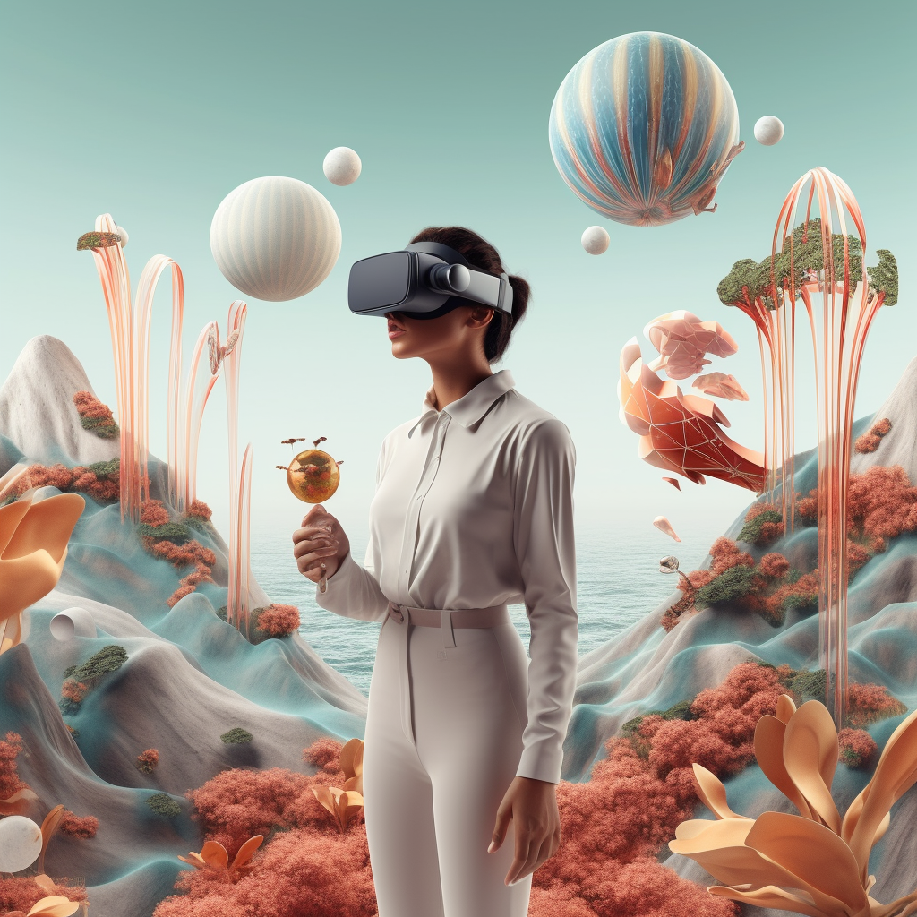
Virtual Reality Science: Exploring the Boundaries of Scientific Discovery
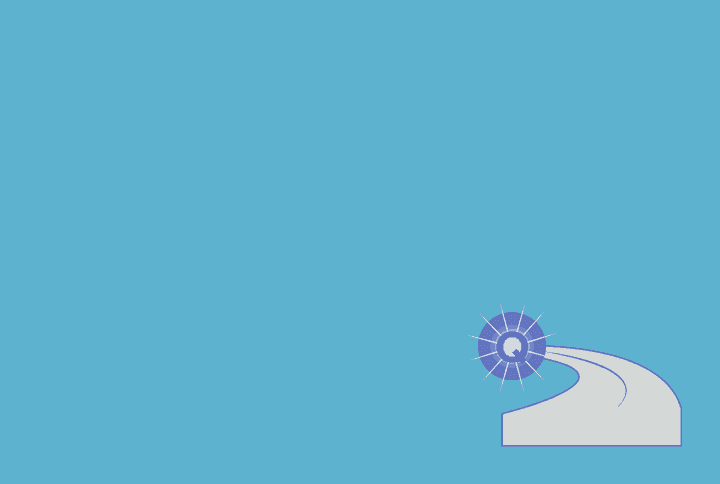
FHIR Claim Response
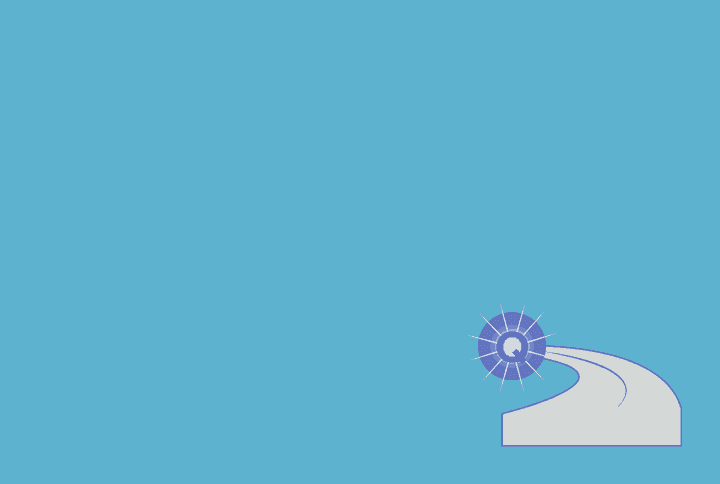
Corsi Block Test
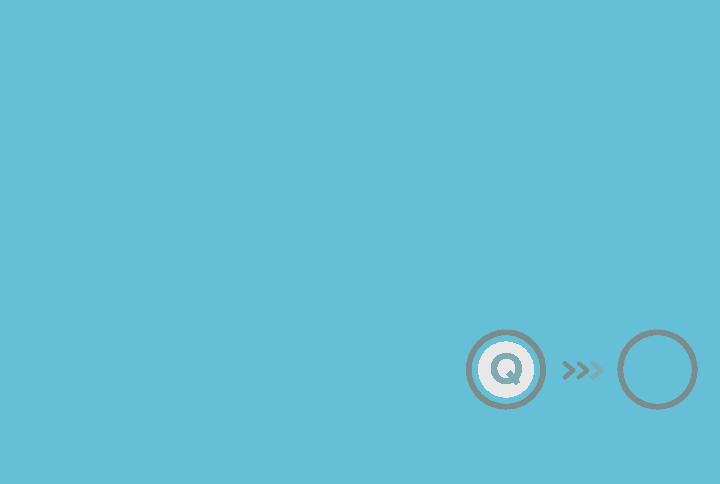
9-Hole Peg

What Adoption of Remote Patient Monitoring will mean for Healthcare Delivery

Digital Health Research: A Guide
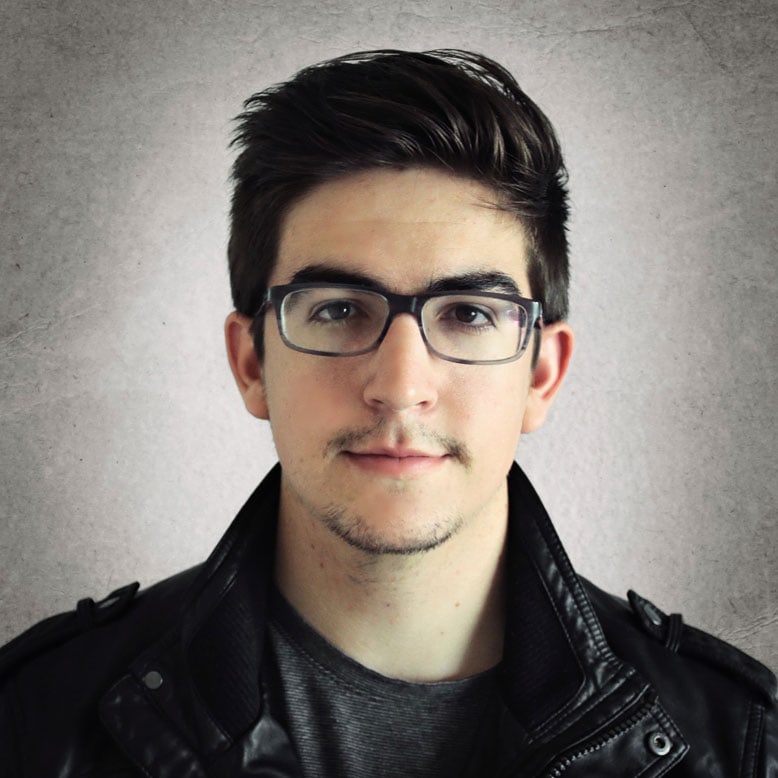
Alex Wormuth: Data Saves Lives
Never miss our posts!
Author:
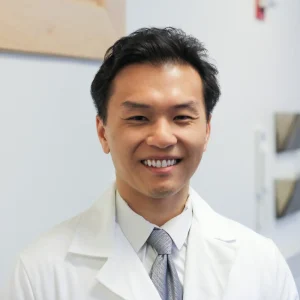
Shuhan He, MD
Shuhan He, MD is a dual-board certified physician with expertise in Emergency Medicine and Clinical Informatics. Dr. He works at the Laboratory of Computer Science, clinically in the Department of Emergency Medicine and Instructor of Medicine at Harvard Medical School. He serves as the Program Director of Healthcare Data Analytics at MGHIHP. Dr. He has interests at the intersection of acute care and computer science, utilizing algorithmic approaches to systems with a focus on large actionable data and Bayesian interpretation. Committed to making a positive impact in the field of healthcare through the use of cutting-edge technology and data analytics.