Validity & Outcome Measurements
Designing a study is a complicated process and choosing the measurements is one of the essential milestones in research. From creating appropriate items to computing statistical analyses, ensuring validity is vital.
Although the definition of validity has undergone many modifications, there are two types of validity: external and internal; both being crucial factors to consider.
External Validity: Aim for Generalizability
External validity is known as generalizability or the extent to which scientific findings can be applied to other settings rather than the ones tested. In other words, external validity reveals if research outcomes apply to everyday life and the general population.
Note that external validity is a complex concept that can’t be measured in a single statistical analysis. Therefore, external validity must be agreed upon by experts. One of the good methods is to implement strict inclusion and exclusion criteria, especially in medical settings. For instance, in clinical trials, a study with good external validity would involve hospitalized patients and would reveal results that can be applied to the general population near the same hospital. On the other hand, in population research, random sampling and high response are needed to guarantee external validity (Peat, 2011).
Here we should mention a couple of curious examples, which tackle the problem of external validity. In psychology, for instance, conformity and diffusion of responsibility are common phenomena. Several studies replicated some alarming findings of people’s nature. For example, a study conducted by Latan and Darley (1968) tested if participants would help a sick person while waiting at a laboratory. The findings showed that people would only act if they thought they were the only person waiting in the laboratory. If there were more people around them, the chances to help someone sick decreased. This study has good external validity. The fatal case of Kitty Genovese who was stabbed near her apartment while her neighbors watched passively through their windows proved the existence of the bystander effect – or the mentioned phenomenon about observers being less likely to help in the presence of other people.
Internal Validity: Improve Your Measurements
Internal validity can be defined as the degree to which a measurement is valid in what it claims to assess. To be more precise, this type of validity refers to the design of the study – or if it measures what it is supposed to assess (Peat, 2011). In other words, internal validity explains how well a study avoids confounders and other nuisances, such as within-subject and between-between observer errors.
To guarantee good internal validity, measurements need to be accurate and precise. Note that for objective measurements, such as spirometers, internal validity is not a warning concern (Peat, 2011). However, for measures, such as subjective outcomes, surrogate end-points, and predictions – internal validity is crucial. Therefore, when developing a new measurement or an instrument that can be prone to bias, such as health-related quality of life tools, a lot of testing is needed to reach good internal validity.
Consequently, to show how robust each measurement is, there are four types of internal validities that researchers need to assess:
Face Validity
Face validity is an essential aspect to consider. Face validity or measurement validity shows if a measurement is at face value and if it assesses what it appears to test. As explained above, often experts need to decide upon the concept of validity as statistical analyses cannot identify good validity. This is extremely vital for subjective outcome measurements. Researchers need to measure if a tool identifies all changes and symptoms if it’s acceptable and precise and if it fulfills its purposes (Peat, 2011).
When it comes to the development of new questionnaires, for instance, face validity can be increased by the inclusion of questions that are relevant, reveal proper wording, and have a good response rate. Although the research panel decides on these issues, both researchers and subjects need to agree about the acceptability of any new survey.
Content Validity
Content validity, known as logical or rational validity, shows if a measurement manages to assess every facet of a theoretical construct. In other words, content validity shows if a questionnaire covers the domain of interest in an adequate way and if it represents the illness of interest precisely (Peat, 2011). This is an important indicator for both subjective and objective measurements. Content validity also needs to be discussed by experts to reach acceptability. Note that in every survey, each item may have different content validity.
Some of the techniques to increase content validity is to cover all aspects of the disease and to measure all confounders and nuisance. In questionnaires with many items, statistical procedures can help researchers include or eliminate items. For instance, factor analysis to find all items that belong to an independent domain and all items that cluster together can be performed. Also, Cronbach’s alpha is a vital indicator of internal consistency – if questions reveal close replies, then they address the same dimension. Note that by eliminating questions that correlate with each other, internal consistency may increase; however, that would limit the domains and the applicability of a tool. Thus, it’s better to include various questions to obtain a comprehensive picture of the disease or the treatment of interest.
Criterion Validity
Criterion validity reveals how well a measurement agrees with an established standard, and consequently, how it correlates with other research measures. Criterion validity is fundamental, especially when it comes to new measures. If new tools prove to be more beneficial than the established standard (e.g., time-effective, cost-effective, and repeatable), then the old gold standard should be replaced. To assess criterion validity – or which tool is better or if two measures can be used interchangeably – the conditions of measurement should be identical, the order of the tests should be randomized, the interval between assessments should be short, and most of all, researchers and subjects should be blinded to the results (Peat, 2011).
What’s more, criterion validity can be used to predict outcomes: in other words, it can be utilized to predict the gold standard results. This property is known as the predictive utility. For example, the severity of back pain in predicting future back problems can be assessed. In this case, aspects, such as the history of pain, current therapy, and objective outcomes (such as X-rays), can be included in the analysis.
Construct Validity
Measuring validity is an important task, and as explained above, there are various techniques that can be implemented in research. Here we should mention a fundamental requirement regarding the study sample. Usually, a wide range of studies (especially when measuring construct and criterion validity), focuses on two defined groups: subjects with a well-defined disease and healthy individuals. This extremity of choosing well-defined groups brings clarity to the analysis, but it has some disadvantages. One of the cons is that it limits the applications of a tool. Thus, it’s recommended to apply outcome measurements to individuals who are not diagnosed clearly or who have less severe symptoms. In addition, it’s beneficial if validity is measured in random samples.
Note that when it comes to the measurement of validity, the relationship between validity and repeatability also matters. Basically, repeatability or test-retest reliability refers to the precision of an instrument. It measures the variation in tools over a short period – with measures being administered to the same subjects, under identical conditions. Usually, criterion and construct validity improve when repeatability is high. Still, do not forget that good repeatability does not guarantee good validity.
References
Barlett, J. (2008). Reliability, repeatability, and reproducibility: analysis of measurement errors in continuous variables. Ultrasound in Obstetrics and Gynecology, 31(4).
Construct Validity. Retrieved from https://explorable.com/construct-validity
Latane, B., & Darley, J. (1966). Bystander “Apathy”. American Scientist, 57, p.244-268.
Peat, J. (2011). Choosing the Measurements. Health Science Research, SAGE Publications, Ltd.
What Is Validity (2013). Retrieved from https://www.simplypsychology.org/validity.html
See more of Our Posts
DIGITAL HEALTH CATEGORY
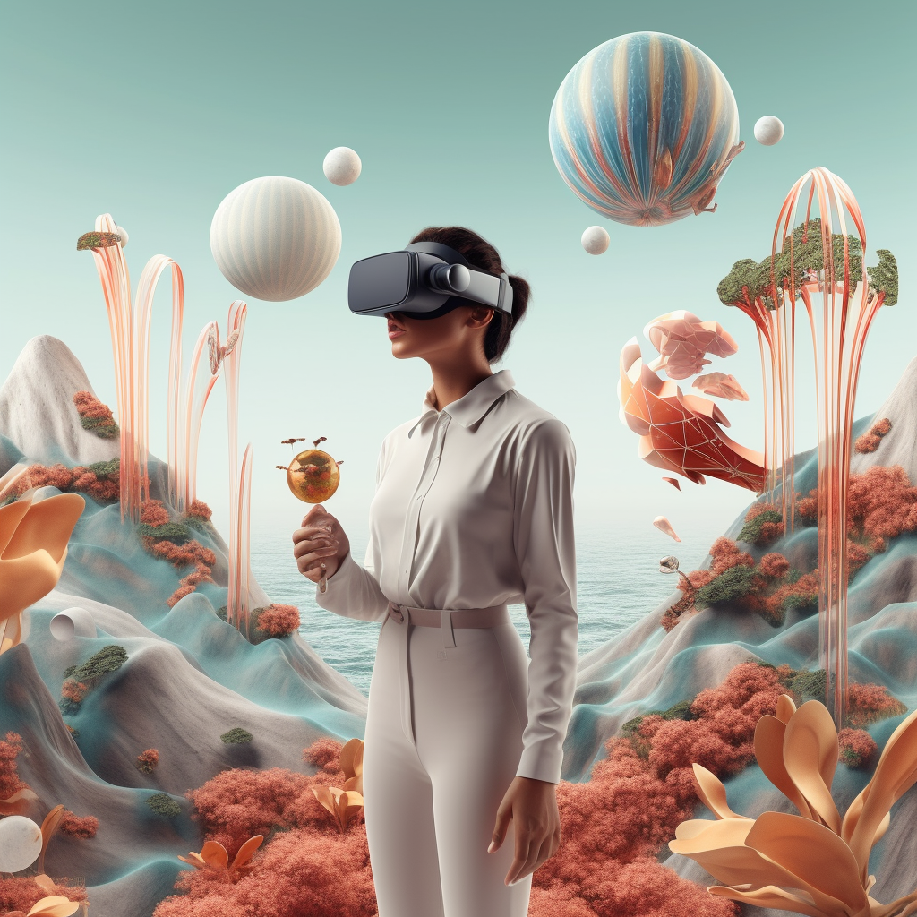
Virtual Reality Science: Exploring the Boundaries of Scientific Discovery
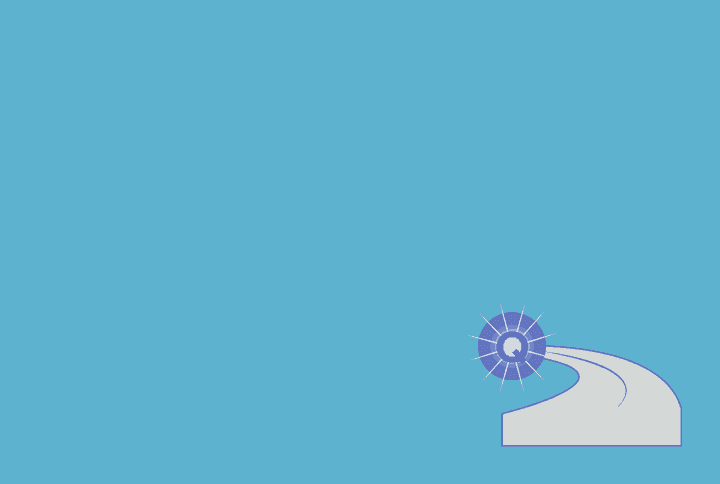
FHIR Claim Response
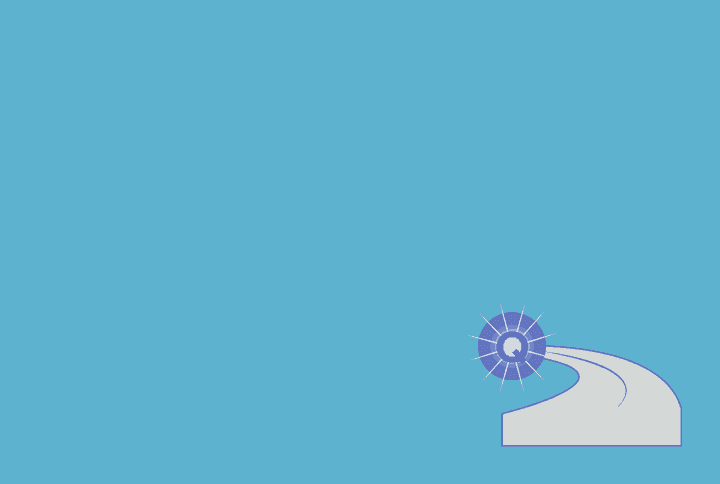
Corsi Block Test
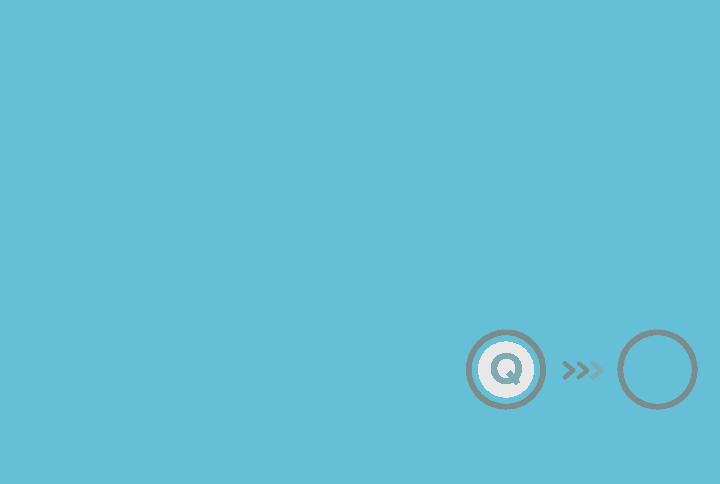
9-Hole Peg

What Adoption of Remote Patient Monitoring will mean for Healthcare Delivery

Digital Health Research: A Guide
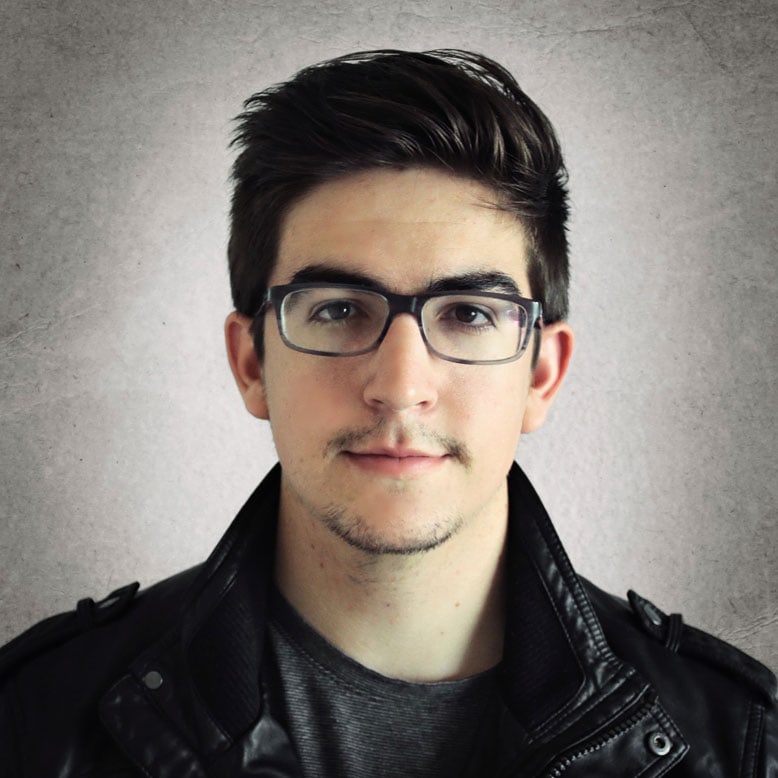
Alex Wormuth: Data Saves Lives
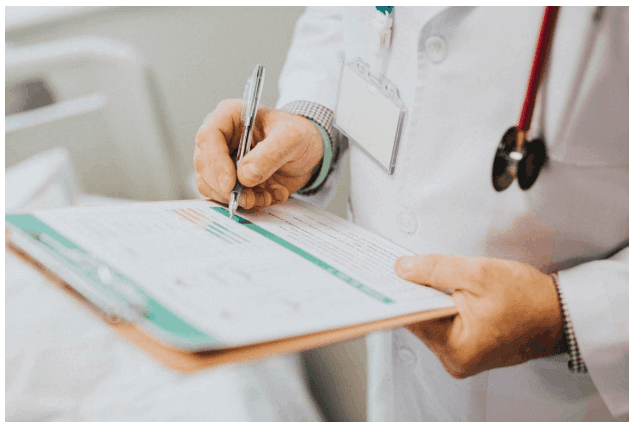
Digital Transformation in the Healthcare Industry: 5 Key Trends

mHealth App Development: Current Practices and Future Perspectives

Schizophrenia in mHealth
Never miss our posts!
Author:
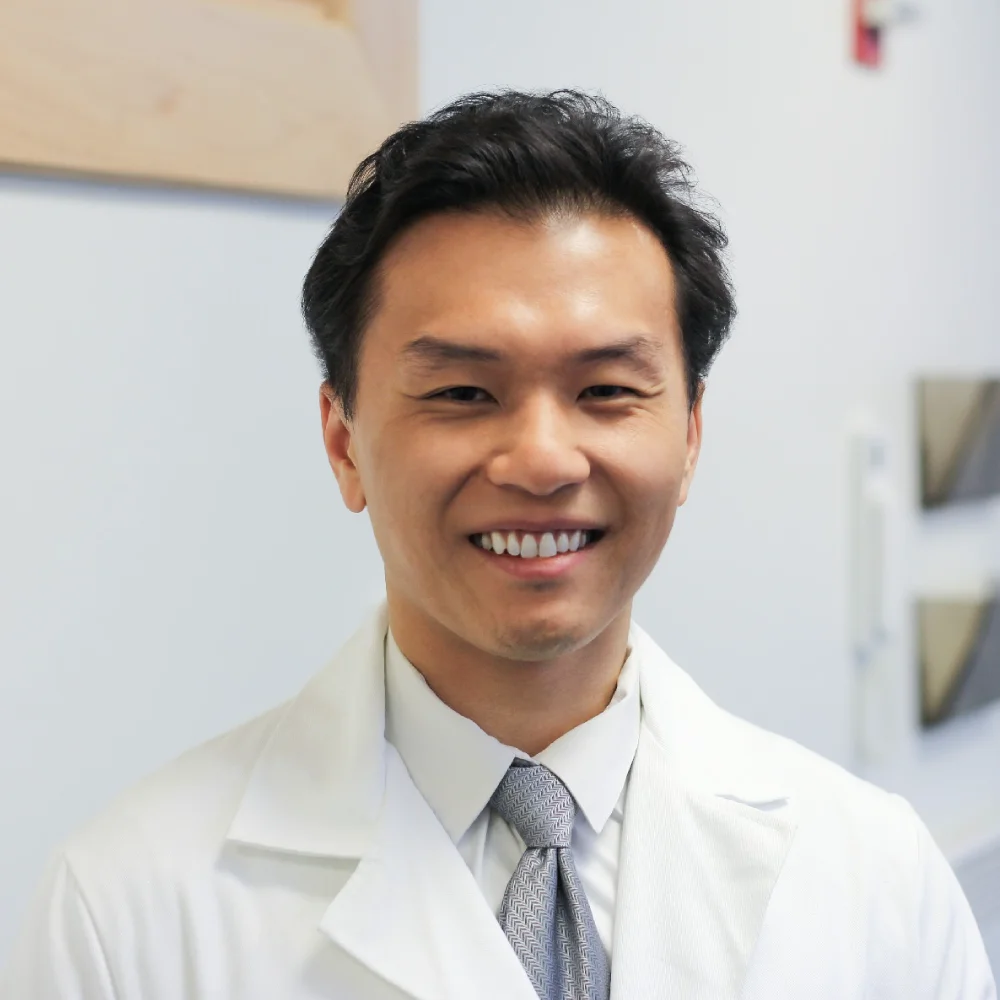
Shuhan He. MD
Shuhan He, MD is a dual-board certified physician with expertise in Emergency Medicine and Clinical Informatics. Dr. He works at the Laboratory of Computer Science, clinically in the Department of Emergency Medicine and Instructor of Medicine at Harvard Medical School. He serves as the Program Director of Healthcare Data Analytics at MGHIHP. Dr. He has interests at the intersection of acute care and computer science, utilizing algorithmic approaches to systems with a focus on large actionable data and Bayesian interpretation. Committed to making a positive impact in the field of healthcare through the use of cutting-edge technology and data analytics.