For years scientists have struggled how to find a way to model and study the brain, something that we do very much rely on but is also such a big mystery to us. One person who is making and has made significant advances in this field is Doctor Jeffrey Krichmar of the University of California Irvine. As in many other areas, one of the most effective ways in which to study how something works is to reverse engineer it, this is obviously a hard thing to do with the brain. But Dr. Krichmar has found a way by mixing together neuroscience, artificial intelligence, and robotics.
Dr. Krichmar graduated with a BS in computer science, and an MS in computational sciences and informatics, and also spent 15 years as a software engineer on massive projects such as the PATRIOT missile system.
He turned his attention to trying to decipher how to brain works and thought this could be achieved by combining the three aforementioned areas due for a simple reason: “The brain is embodied, and the body is embedded in the environment” thus the brain needs a body, and that body needs to be in the environment to relay information to the brain. As such you need to build a ‘body’, or in this case, a robotic body to measure the response of the modeled brain to determine the real and observable results of the experiments. Dr. Krichmar has worked on a few notable experiments such as the Darwin X robot which contained nearly 1.5 million digital synapses, 100,000 neurons, and developed hippocampus place cells as seen in rodents and humans.
This monumental discovery led to back-tracing the origin of place cells, which has led to the now accepted model of hippocampal function.
Dr. Krichmar’s work and other projects have very important and unintentional real-world consequences. While their artificial intelligence (AI) is still being experimented with, as they make more and more progress their research paves the way for new technology. We will have and start to see smarter and smarter AI around us, from Amazon Echo’s Alexa to self-driving cars.
We had the amazing opportunity to interview Dr. Krichmar.
You can listen to Under the Microscope by using the player above, searching for “The Conduct Science Podcast” on any place you listen to your podcasts, using any of the links below or you can download it HERE. You can also read the transcription down below.
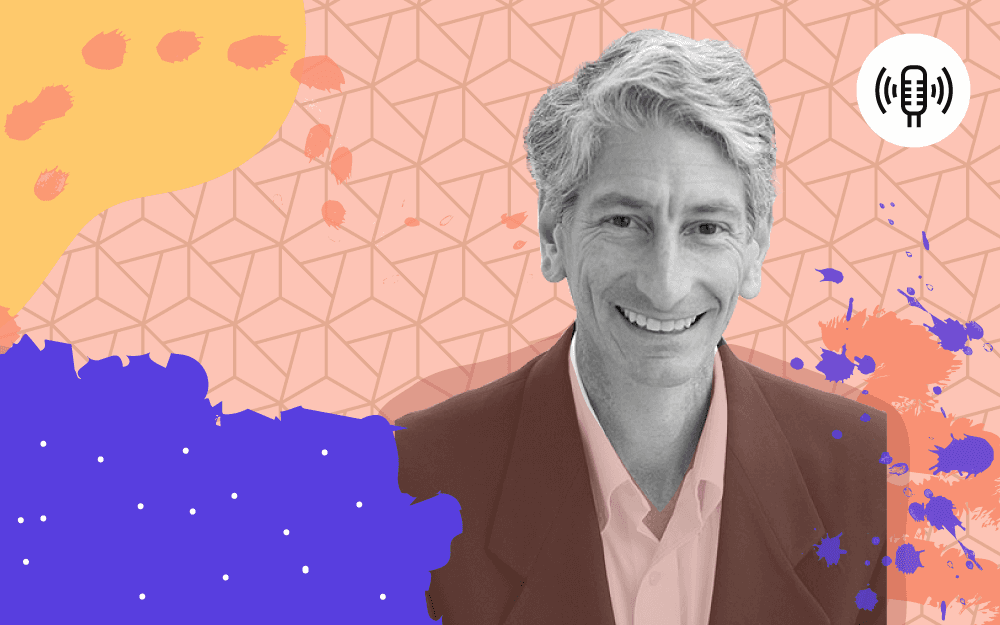
Today’s Guest:
Episode Description
In this inaugural episode of Under the Microscope Tom is speaking with Dr. Jeffrey Krichmar, a professor at the University of California Irvine. His revolutionary work has led to the now accepted model of the way the hippocampus works in our brains, we discuss this, why using robots is critical to the research of how the brain works, how technology affects and is affected by his research and we even take a look into the future af artificial intelligence. Music by: Joakim Karud – https://soundcloud.com/joakimkarud.
Thanks for Listening!
Feel free to share your thoughts on our Twitter here: @Conduct_Science
Use #ConductScience on twitter to:
- Suggest a guest
- Suggest a topic
- Ask a question we can answer on the show
- Or to just get in touch!
Learn about our products:
- Visit our Lab
- Find Resources for your lab including protocols, methodologies, and more.
- Are you a scientist with a new device that you would like tech transferred? Join our Creators Program
Transcript
Tom: Hello guys and welcome to the first ever conduct science audio interview. I’m incredibly excited to be sharing this with you today and I can’t wait to get it underway. So today I’ll be speaking to doctor Jeffery Krichmar who is a neuroscientist from the University of California Irvine. And I really hope you guys enjoy this as much as I did conducting it. So without further ado…
Tom: Hello everyone. Today joining me is Dr Jeffrey Krichmar from the University of California Irvine. So to start, why didn’t you just tell us a little bit about yourself? What’s your background? What do you do? What do you research?
Jeffrey: Well thank you Tom for setting up this interview. Uh, yeah, I was trained originally as a computer scientist and uh, I was, did my undergraduate work at UMASS (University of Massachusetts) Amherst and then, uh, I did a number of years working in industry on work that was embedded in real time systems. Uh, at some point I went back to graduate school and was getting advanced degrees in artificial intelligence and got very excited and interested in neuroscience and so really shifted gears or, and started looking more at how the brain works and how you make models of the brain. And uh, which led all the way to a PhD and then by the time I got my PhD and started trying to think of what my research would do, would be, um, I was thinking like a about the computer models of the brain, but also tying it back to some of my real time embedded system working in the software engineering industry and that, that got me thinking like, well, what if you put these brain models on our robots and have, uh, the robot control by these artificial Brains and that. That’s led to a very long career in that area of research, which we now call neurorobotics, but it’s gone through several, several name changes over the years.
Tom: Yeah, I’m sure. So some people listening may not kind of see the connection between how the mind works and needing robots to demonstrate this. So would you be able to just enlighten us on that area a little bit.
Jeffrey: Yeah. Um, so we have a phrase that we use in our lab. It’s called the “brain is embodied and the body is embedded in the environment”. Um, and what that means is brains don’t work without a body. Um, and there’s a close coupling between what the brain is doing, what the body is doing. A lot of people think that the body is actually telling the brain what to do and not the other way around. And then your actions in the world, uh, make a huge difference. And it changes the world in that interaction or reaction, uh, can lead to emerging of some really interesting behaviors. So, um, this was really started to study, you know, a better model for studying how the brain works. Because as a modeler and roboticists I have control over what the body is doing and I have the ability in this artificial brain, which our artificial brains have had anywhere from thousands to millions of neurons and connections. But we have access to every single neuron in connection as the robot is doing its behavior and its learning and so that gives us a really powerful tool for studying the brain, body and behavior, which you can’t do with animal models.
Tom: You get, uh, an inside look almost at everything that’s happening.
Jeffrey: Exactly.
Tom: Yeah.
Jeffrey: And then there’s a flip side, which it’s more of the artificial intelligence that if you look at any organism that has a nervous system in biology, they’re so far ahead of what we can do right now in artificial intelligence. So this might be a model or a framework to make more intelligent systems.
Tom: Yeah. Um, so you’re saying you’re building the body for this mind, you’re building the robots and all the senses and everything that goes on that when you’re thinking about building this artificial brain as it were, is it, is there any like physical components to that? Is it all software? Is it a mix or how do you kind of come to that?
Jeffrey: Yeah, the brain itself. So you’re right. So the robots, we either make our own custom made, uh, or we, uh, use off the shelf robots that have a really good rich sensor and mobility. Um, the brain models are done primarily in software. So, uh, it turns out the equations to capture the dynamics of a nerve cell or neuron are not that bad as far as a, you know, a differential equation is, and the same goes for the, the change that the connection or synapse where a lot of the learning and memory takes place, the differential equations for what’s going on there are not that bad to model mathematically and in software. What, what becomes more of a challenge technically is, um, you know, you’ve got let’s say 100,000 neurons in the model and several million connections and it’s on a robot. So it has to operate in real time. So then it becomes from a computer science point, a interesting and difficult, uh, parallel processing a problem.
Tom: Yeah. So when you’re, you’re modeling these minds, obviously as you’ve just mentioned, there’s a lot of computing power that goes into that. Are you find, obviously there must be some heat generation with the such a powerful processing. Do you find you’re limited in how much you can model?
Jeffrey: Yeah that’s a very good point. Um, there is, there’s a limit. It’s the heat, but even more so the energy consumption. So your robots are on batteries if their autonomous. So having on the onboard computation and limits your time that you can do this. I’ve worked on a couple projects, uh, in a field called neuromorphic engineering and they’re making hardware that mimics the brain. It’s very parallel. It’s event driven. Uh, it uses a representation like the brain does, uh, neurons that spike. Uh, and that leads to a very efficient, um, both in memory ending and energy consumption, a way of representing information. And so those things operate on low power and they’re, they’re just coming of age. Uh, we’ve done a couple of experiments putting them on one of our robots. Um, and you know, a small hobby grade battery can control the whole neural network operating the robot, uh, and, and also power the robot itself.
Jeffrey: So that’s a huge, huge, right. I think for especially, you know, the edge computing and the in the embedded system computing. Um, as you know, more AI applications go, you know, away from power sources. I think that’s a huge thing and that’s a good point you bring out. These models when we run them on a GPU suck up a lot of power and a, and a lot of heat. And usually if you’re, if we’re doing a large scale neural network on a server, um, you have all these fans running and in dedicate your conditioning. We can’t do that if you’re going to be a robot out in the field somewhere.
Tom: I guess it is the crux of science in a way is waiting for the, uh, the technology often to catch up with what your trying to do.
Jeffrey: It’s catching up, which is exciting. Yeah.
Tom: Yeah. That’s good. It’s very exciting. I noted in the Darwin x, Darwin 10 project, um, this was incredibly important. Not only for you and your team, but neuroscience has a, as a whole, I think you managed to trace the backward processes of the hippocampus quite impressively I might add with 100,000 neurons and 1.5 million digital synapses. So, I mean that’s just sounds impressive in itself and I can kind of imagine what that would look like. Software or hardware wise. So, can you tell us a bit about the process, like the, the methodology you went through to pull this together to make this come to be?
Jeffrey: Well thank you. Yeah, that was, that was an exciting project. Uh, we, um, yeah, there was, there was just trying to pull it off. To have a network that large new, and given the time, I think the paper came out in 2005, but, uh, you know, the work started a couple of years prior to that. So, you know, we have limited computing power in those days. So somehow we were able to pull it off. And like you said, I said before, had to run it realtime. Um, that was when I was at the neuroscience institute. And, uh, this model, ofthe hippocampus was a dream of the institute director Gerald Lieberman. And there are several people on the, in the group that worked on that. Um, one part of the process was working with Doug Nitz who is also at the Institute at the time. And He, uh, was one, he’s one of those that studies the rat hippocampus, uh, and surrounding areas.
Jeffrey: And he had a vast knowledge of what the neurons should look like and what the anatomy and connectivity should look like. Uh, there was a lot of data out there, but, but we worked very closely, Doug and I to make sure the model had all the, the right aspects that, that are in the real circuit. And so there’s, so there’s, that was, that project was driven by, we want a model of this particular brain area. So then you think about all the inputs and outputs within that brain area, and then also the inputs and outputs between that brain area and other areas. Uh, so that was important. And then the, the question was, well, what should this do? But, uh, at that time, one of the gold standards for understanding spatial memory, especially in the rat, was something called a Morris water maze.
Jeffrey: So, so you don’t know what that is? They’re usually, they put the rat in a tank of water, the, there’s some milkiness in the water, so it’s opaque. So they can’t see through the water. They’re swimming along and somewhere just below the surface, they can’t see it. But there’s a, uh, a platform, so they’re very good swimmers, but they don’t like to be in the water. So they’re very, you know, pleased when they find this platform, and it triggers their, their rewards system. And so they’re quite motivated the next few days when they get put back in this tank to understand the spatial layout so they can swim directly there and get out of the water fast. So, you know, we, we didn’t want to make a submarine robot, so we, we made a, a drive variation of this where we had a black floor. Uh, but one part of the floor had a different reflectivity and we had a downward facing sensor that the robot could literally feel, couldn’t see, but it could feel when it actually was on that spot.
Jeffrey: And yeah, it was pretty, it was, we’re pretty excited when it worked. You know, you start seeing the robot showing this goal directed behavior when you started seeing in the hippocampus itself, the emergence of these place cells, which had been shown in the rat. And then like you said, that that ability then to use the whole, since we had the whole brain, the body and the behavior to trace back how, how a neuron that was saying, I’m, I am here actually, what, what other neurons led to it, you know, knowing its place in the world, that, that was the backwards trace that you referred to. Yeah.
Tom: That must be such a rewarding feeling after two years of work, at least to see something you physically built and put together behaving as something almost biologically. Would.
Jeffrey: Yeah. No, that was, that was exciting. That was one of my favorite projects. Uh, just because of the details that went into it, the process of putting it together. And then I still, when I give talks, show the, the video that goes with that. Cause it’s, I think it’s really just you see the behavior and it looks like the robot really has a goal in mind. Yeah.
Tom: Yeah. That’s amazing. So you talked about the technology where we are now and maybe the technology where you are, were with that project in 2005, how has the, the technology changed in your career? Has the, has that influenced the work or as the work influenced the progress of technology?
Jeffrey: Well, we’ll start with the technology has influenced the work, uh, computation and much better now. So back in the, the early days of doing this, we had the, you know, the computer’s running the robot. We’re on the second floor of the building and the Robo was on the third floor and then we had to set up Wifi links. So it seemed like a robot’s brain was on it. Yeah. We do a lot more now on board, which means we can do a lot more interesting, not just in the labs. So we taken our robots, Yo in parks and to mountain trails. Uh, we have a new robot that we’re using in classrooms. Um, so that really gets the robot out into the environment instead of lab work. Uh, another amazing technological, um, advance has been cell phones or smart phones. So, we’re getting tired. Every time we did a robot project, we would custom make a robot. It would take several years, big expense.
Jeffrey: You do your, your experiment. And then, you know, I have a kind of a robot hall of Fam or Grave Yard, depending on how you look at it, where I have all these robots sitting there, they’re down there, go down there and they’re, you know, they’re just sitting there. Um, so I had a postdoc Nicholas Oros, a few years back that looked at android smartphones and found a, a little interface board that the smart could talk to. And so then we started using the smart phone as the compute engine. Cause the computers on the smart phones are getting so good. And the smart phone had a really high definition camera, uh, had a GPS system.
Tom: So everything you need for the core of a robot.
Jeffrey: Yeah. And then there was a Bluetooth link that you can link to this board which could then control motors, could you could add extra sensors so we can do some very cheap rapid prototyping with these android base robots. That’s made a big difference. That’s made us much more mobile and do interesting things.
Tom: Oh it was really speed up the process of even testing as well. Cause if they’re that adaptable you can just put them into another robot or something or change something slightly and you’ve got a whole new test or repeatability is easy.
Jeffrey: Yup. Exactly. And then also coupled with that, with 3D printing and the rapid prototyping there, uh, I have some engineering students that like to work with us cause they are interesting designing robots so they can use it like on campus are our 3D printing or rapid prototyping. So we can make new body designs fairly quickly too.
Tom: Okay. Say that that’s come about in recent years and that must be a huge advancement in. Everything you do really
Jeffrey: Yeah, and not just our lab, see all these other labs. They are more a lot of the prototype robots or are using 3D printing. And it makes for great way to do. Yeah. Now has our work influenced technology. I think so. And it’s a little tougher sell. Um, we’re, we’re bringing making these brainy robots, but a lot of the ideas that have come from our lab and a lot of other labs that did this. Have influenced, you know, computer, computer vision, you see a lot of neuroscience ideas, they’re learning, uh, learning, how you reinforce things that go well, reinforcement learning, uh, navigation. You know, a lot of ideas from navigation, like the Darwin Ten and some other people you know, who’ve made slam models based on the rat, like a, um, a colleague that I know in a couple of colleagues I know from Australia, Janet Wiles and Michael Milford made a very popular slam algorithm based on the rats neural network. So yeah. So I think, I think that this is going to happen more as AI is sort of doing amazing things, but it’s reaching certain, you know, crossroads or limits that they need new ideas or talk to a bunch of them there. They’re actually turning to neuroscientists to come up with new ideas to advance what AI is already done.
Tom: Well it makes sense. I think we always look towards nature in a way to figure things out, especially in science. You know, we look to what’s, what’s there, what, how can we get to the standard? So why not come to neuroscientists who know, who know that?
Jeffrey: Yup, exactly.
Tom: So I’ve seen some in your earlier work with Carl 1, and you were saying about learning, you were using in that system more of an operant learning methods. So there was a good action. You’d give the robot a good signal that was a bad action. Uh, you give the robot a bad signal. So do you still do this and make every robot go through the same process? Or is there a way you can kind of skip this new learning process? Can you just like upload, where you’ve got to with the previous robot maybe?
Jeffrey: Oh, okay. Oh, there’s a couple of questions in there. Um, I’ve been interested in for years in what’s known as value systems. Um, and in the brain they’re called neuromodulatory systems. So there’s, without going all the, unless you really want the neuro-biological details there, there are chemicals in the brain that carry important value signatures. So, um, one is, is good is, is reward. Um, there’s, there’s another signal or several signals for I would be, it’s a little more nuanced than just bad. It’s more things that could be harmful and risky and in what your, what’s your level of risk taking should be. Um, and that seems to find our way in most robots that we design that, that there’s some modulatory signals that are signaling value. We’ve, we’ve gotten more flavors of this cause there’s, there’s value signals for your curiosity. There’s value signals for how you deal with the uncertainties in the world. Believe it or not that we, we sometimes filter these things out and sometimes we are quite responsive to these and there’s different levels that the brain sets based on these chemicals. So, so we’ve kind of moved from just good and bad to having all these interesting nuance signals and also in the brain. Um, the more we looked at it, all of these signals seem to interact with each other. And can compound each other. Uh, and that gets very interesting and complicated as you can imagine. So
Tom: yeah, I was going to say, that must be.
Jeffrey: Yeah. So that was supposed to be when I first came to moved to Irvine and in 2008 that was supposed to be like a one or two year project and we’re still, yeah, so we’re 10 years later still working on models of that because there’s just, it’s so rich and it makes a huge difference. It can set the behavioral state, the context, it can really rapidly shift, uh, what the organism can do. And it has big implications, implications for artificial intelligence because if you get one of these signals, it might tell you, oh, I’ve got a, at least what I was doing now, and adapt to the kind of the new signals coming in that that’s hard for the AI system right now. But that’s what we do. We, you know, something changes. We don’t just fall apart or, or need hours or days of retraining, we would just deal with it right away. These systems seem to be very important for that.
Tom: So we’re kind of moving away. So where we think of, or I personally think of computers and such as been quite binary systems. You know, it’s either this or that, this or that. And you’re trying to just break that down in artificial intelligence where they have this mesh of incoming signals that interact with each other in a more natural sense.
Jeffrey: Yeah. Oh well said. Yeah, that’s exactly right.
Tom: Yeah. That’s really cool. That’s really interesting.
Jeffrey: Yeah. Carl 1 was pretty much a binary signal if it’s good or bad, but yeah, but they did lay down the groundwork because we could see how, how rapidly the signals could change Carl’s behavior. And then also, um, also how, how, how, how real behavioral effect cause when, when it happened, if you are watching Carl in our lab, it, it making a very rapid change, uh, in where we looked. And so it looked like it really suddenly realized, oh, there’s something very important I have to attend to. So if you anthropomorphize it you go wow, everyone will come in and are like, wow, that thing really looks like is you know all of a suddenly really interested in this green color, this red color, you know. Um, and I think that was another clue that these, these signals can cause a rapid, rapid shift of attention that the animals get all the time.
Tom: So leading on from that, and there’s also the question of when you’re developing these rules, these good and bad signals, how do you deter that they don’t cause a bias, uh, one doesn’t overly affect the others or one slightly unwanted signal is causing too much of a bias compared to others?
Jeffrey: Yeah, no, that’s a good point. Um, there’s a, there’s a couple things in that point. Uh, bias is definitely an issue with, um, with any researcher. One Nice thing about…
Tom: Yeah definitely.
Jeffrey: …One nice thing about the robots is it keeps you honest because you can’t, you aren’t biased by simulation or virtual world, uh, because you have the real world. Um, another way we try and get around the bias is we try and match the behavior of the robot. And the signals the robot responds to, to what we have seen in animal models, animal behaviors. But it’s still an issue. The bias is still an issue. And the other thing that I haven’t successfully solved, and I don’t think anyone else has, is, you know, the, the, these values we talk about for an animal are real, you know, it effects their body physically.
Tom: Oh okay, yeah.
Jeffrey: There’s needs, if they, the reason, the reason an animal is hungry as is, it needs food energy. You know from food to metabolise it, but you know, you stay the same in a robot value system. Uh, you know, that that’s not, it’s, it’s not real to the robot. That’s something I’m trying to struggle with. I mean you could say if the battery is low that it’s hungry, but there’s a bunch of other things that are real cues to our body and to what drives us. You know, these low level drives that have a real effect on our physical wellbeing. Um, so that’s something I think if we could do that in the robot would also give us a better model. But I think that would cut down on the bias as well.
Tom: Yeah, I think that’s, that’s part of science though isn’t it? It’s just getting rid of as much bias as you can to perform a standard experiment in whichever field it is. Yeah. Um, so this is kind of where you are now, uh, with the current things you’re doing, what do you reckon lies in the, in the future? Do you reckon we’re, how far away are we from modelling, say the whole brain? Cause maybe, are you kind of restricted at the moment to modelling certain parts at a time.
Jeffrey: Yeah. I mean the models are getting bigger. Um, it’s still there. There’s still a lot of issues of how you put all the pieces together and it’s not just computation because our computers have gotten bigger and better. Um, it really has a lot to do with, uh, once you have multiple, even with, uh, AI and deep learning neural networks, once you have multiple networks of these and the brain has many different sub networks. Yeah. They all are interacting once they interact and it really changes the dynamics. Um, in a big way. Um, and I’m sure that’s important for real brains, but it’s difficult and in all of our artificial neural networks to actually keep these things under control and working well. Um, so that I think is going to be something in the future that a lot of us are, are dealing with. I know we personally are dealing with that and in one of our new projects where we have multiple brain networks, we’re getting recordings from, we have to somehow link them together.
Jeffrey: And I know the community as a whole is now thinking, well, I’ve got this one neural network to recognize these things and another neural network to recognize some other things and how I have to put them together in a whole system. So that system integration is going to be a big issue to moving forward, I think. And also there’s a lot of work still in the robotics community, robotics has come along way, but the robots are still mainly hard bodied. Brittle. Nothing like the flexibility and mobility of a, of real biological organisms, humans or any other, uh, biological organisms. So, so we need a lot of work there that almost becomes a materials problem. And then I also mentioned the issue that a lot of this work to really make more advances is going to move out of labs and into the real world and a lot. And also, you know, exploration like, uh, in space or on the deep sea floor. Um, and we’re going to need low power computer processing at the edge because they’re going to be away from anywhere that they can get a power, a power source. So there’ll be far away from a power source and another issue is they’ll probably be breaking down far away from it where anyone can fix them. So they going to need some health monitoring and maybe some self repairs. A lot of, a lot of interesting.
Tom: Yeah, exactly. A lot of places to go.
Jeffrey: Yeah. And the future.
Tom: Yeah. Um, so on a bit of a lighter note. There’s a, I wanted to ask, how far away are we from the Kurzweil technological singularity? Um, for those who are listening who don’t really understand what this is so as a prediction may from a man called Ray Kurzweil and he predicts that at some point artificial intelligence will transcend the intelligence of man, as close as 2045, I think is his current estimate. He goes far as to say they’ll get bored and leave planet earth and leave us all behind. What do you think of this? Is the sci-fi or is this a future we can expect?
Jeffrey: Um, I’m not a believer in that idea. I know there are some components of it. Yeah, there’s some things. I think one thing is the original idea was that uh, the singularity is based on the amount of computing we had, which could, uh, at the singularity point, the amount of computing, uh, would be as much as the computing and the, the human brain. Uh, but I, I that’s I think flawed because you’re comparing apples and oranges. The human brain works very differently. Human brain also, like I keep saying, you know, brain has embodied and the body is embedded in the environment and it is linked closely, closely to how our body works. Um, so just having pure computation, I don’t think you’re gonna I don’t agree with the singularity idea. I also just knowing where we’re at, we have a long way to go. There’s a lot of issues that we’ve talked about in these last few minutes of that that need to be resolved, going to take many years to resolve.
Jeffrey: Um, I think the robots, the systems, will get more and more intelligent. I think that the people designing these, uh, for the most part do not want to make something that’s, uh, yeah, the, the, that’s sort of why would you, why would you even want to make an artificial system that’s smarter than humans that gets bored with humans? I, and we, I think we are as engineers in control of what we’re making. Um, I also, you know, always when I give talks, a lot of people, they asked me about Skynet. Again, I know the idea is that we’re, we as engineers, it’s our duty to, to make these safe. I wouldn’t want to work, uh, around or operate a machine that I don’t have full control over. So we, we always put lots of safety mechanisms and these, and we are designing them to work within certain constraints.
Jeffrey: And I don’t think that’s going to change. I do think though, I don’t know what you said, 2045, I think by 2050, we’re going to see, and we’re already seeing a lot of these intelligent systems being tools that we use in our lives. And the more intelligent we make them more natural, make them, the easier it’ll be to interface with them, the easier it’ll be for them to, to help us out. Um, for sure. So I see a big push in, in the next few decades and in health care, um, assistive technology. Uh, and like that we also talked a little bit before like exploring places that are hard to reach for, uh, for people, um, you know, in kind of pushing the frontiers in that way. I see like huge, um, benefits coming from, from this current of technology in those fields.
Tom: Yeah. Uh, so I’ve, final question for you. This may be a bit of a tough one. Um, so you’re, you’re trying to model something that the, the brain, the mind consciousness, even maybe if you stretch that far, it’s something we still don’t fully understand. And there are, you know, hundreds of definitions floating around with, from everyone. So what would be your favorite definition of the mind? The consciousness. The, the brain.
Jeffrey: Oh, ooh, that’s a tough question. I would, like you said, there’s no good definition for consciousness. We argue about this forever. But, um, I do think what, what we can see or what, what I would like to see, um, is systems that are really intelligent, adaptive, and fluid. And I think that’s what cognition is. That we can not only react to what’s going on now, uh, but then we can also use this knowledge, knowledge about what to do in the future. Uh, and that when something happens that we either respond to it with something we remember from the past or we realize this is a whole new situation, I’m not going to fall apart. I’m going to figure out what to do now and learn from my mistake. Uh, and I think that goes to the heart of what cognition is. And maybe as we, as, as this cognition gets better and as you are able to, you know, think about past, present and future, um, maybe that’s what consciousness is about.
Jeffrey: Yeah. But that’s about as bad as far as I’ll go because. It’s very hard as the problem, we just had a little panel discussion. It was interesting. But the, the real hard thing, you know, it let’s say in my field is, how would I convince you that my robot is conscious? You know, there’s more problem. I mean you and I, I’m assuming you’re conscious because I can put myself in your shoes and we can have this conversation. You’re acting like I would expect another person to act and you’re maybe the same with me and so, but you know, if it’s an artificial system is ways to sort of fool that cause we know it’s not the same as you will need. So that, that’s why I try and stay out of this even though it’s really, really interesting. Yeah, no that’s fair. I suppose it is for the field of neurorobotics, or cognitive robotics. It is kind of like the ultimate, you know, goal tp get a system that someone said that’s a conscious system and then you know, you really like achieved like some sort of pinnacle or trans, you know, they got discovered.
Tom: But I guess since no one knows what that looks like from since you have the inside look to what the brain is doing in your robots, no knows what consciousness may even look like. So yeah, that’s the holy grail.
Jeffrey: It is the holy grail. Um, but you know, as we develop this, hopefully as systems get more and more brain power, literally, I mean, you know, using the, using the ideas of that we know that are all the great discoveries coming on neuroscience and, and maybe a, the system becomes more and more intelligent and that really maybe said is able to tell us something about how conscious works.
Tom: Amazing.
Jeffrey: That’d be wonderful.
Tom: Yeah. All right, well I think that’s it for me. So thank you very much for joining me today it has being incredibly interesting conversation and I can’t wait to find out more about what you guys end up doing and what you discover next. So I’m excited for you.
Jeffrey: All right, well thank you very much. It’s been a pleasure.
Tom: Once again, that was doctor Jeffery Krichmar from the University of California Irvine. And if you guys enjoyed that, we have got lots more interviews lined up for you from scientists from lots of different fields. If you want to be able to find these, you can head over to conduct science.com or alternatively you can find them integrated into some of our conduct science podcast episodes, which you can find on all of the latest podcast directories such as Itunes, Spotify, stitcher, and pod bean. Just type in the conduct science podcast. But that’s all from me today. So I will see you guys… Next time.