Choosing the Outcome Measurements: Planning Is the Core of Research
Conducting a medical study is a challenging process. From choosing a study design to computing a statistical analysis, medical research is complicated. On top of that, health-related studies must follow safety and ethical regulations, which adds a burden to researchers. To avoid any possible complications, planning is paramount.
Choosing the right measurements is an essential part of each study. Outcome measurements should be well-defined and accurate in order to help researchers understand the connection between variables, assess the benefits of a new intervention, and improve patients’ well-being (Peat, 2011).
Defining the Outcome and Explanatory Variables
When it comes to choosing the right measurements, explanatory and outcome variables also need to be clear and easy to measure in order to test the main hypothesis of the study. Note that the explanatory variable is a type of independent variable. While independent variables are completely autonomous and unaffected by any other factors, explanatory variables are not entirely independent (“Explanatory Variable & Response Variable: Simple Definition and Uses,” 2015). Still, explanatory variables are vital as they can explain any possible changes and can affect the dependent variable. In fact, most phenomena are interconnected. Let’s say one wants to measure the effects of fast food and soda drinks on weight: these variables are not completely independent as food corners often offer menus that contain both options. Thus, independent and explanatory variables are two terms that are often used interchangeably. However, in precise clinical studies with multiple outcomes, all measured factors should be well-defined.
On the other hand, dependent variables, also called outcome and response variables, are the factors that are expected to change during an experiment. The outcome variable is the focus of any study, including clinical trials. For instance, experts may be interested in treatments that prolong the life of cancer patients. The type of treatment (chemotherapy, for example) will be the explanatory variable, while the survival time will be the outcome variable (“Explanatory Variable & Response Variable: Simple Definition and Uses,” 2015). Let’s not forget, though, that today’s medicine and healthcare technology focus not only on mortality rates but patients’ overall well-being and quality of life (especially in severe and chronic conditions).
Subjective Vs. Objective Outcome Measurements
Choosing the outcome measures can be a tricky task. All outcome measurements are vital for research and experts can decide on either subjective or objective outcome measurements. Both subjective and objective outcome measurements have their benefits and challenges in practice, and it’s a fact that there’s not a one-model-fits-all approach.
Subjective outcome measurements, for instance, are defined as any measurements that are open to interpretation. They can be self-administered, administered by an observer or a medical professional. One of the advantages of subjective measurements is that they are easy to administer, cost-effective, and rapid. As such, they are an effective method in clinical trials, which can assess if there’s an improvement in people’s self-reported status (Peat, 2011). Examples of subjective outcomes are questionnaires about the frequency of symptoms or illness severity. However, as the name suggests, subjective outcome measurements are based on subjective judgment, and as such, they can be prone to errors and bias. Therefore, when it comes to clinician-reported outcomes, for example, training of staff is crucial in order to avoid observer bias.
On the other hand, objective measurements gather medical information collected by standard instruments or professional equipment, which usually reduces bias. For instance, lab results and biochemistry data are examples of objective measurements (Peat, 2011). However, one of the disadvantages is that these measurements collect short-term data that changes quickly, such as blood pressure. Nevertheless, objective measurements are precise, and therefore, highly implemented in research.
Multiple Outcome Measurements
Multiple outcomes are also paramount in research, and clinical trials and experts need to consider them when choosing the outcome measurements. Since any new treatment affects various factors in one’s life, often one single measure is not enough to reflect all the physical, emotional, and social changes in patients (Tyler et al., 2011). In fact, in cases when the most important outcome is unclear, and effectiveness and efficacy must be checked across various domains, multiple measures are needed (Peat, 2011). However, when designing a study with multiple outcome measures, there must be a clear differentiation between primary and secondary outcomes in order to overcome all statistical challenges in the analysis. In fact, often researchers have a small set of measuring tools as primary outcomes and a broader one as secondary.
Note that outcomes that are significant from a medical point of view might be perceived as less important by subjects. For instance, experts may aim to reduce hospitalization rates, while patients may aim further, as returning to work.
Surrogate End-points & Clinical Trials
Note that in long-term clinical trials choosing the right outcome measurements is a delicate process. In fact, the primary outcome variable is called a surrogate end-point or an alternative short-term outcome. Surrogate end-points are defined as biomarkers “intended to substitute for a clinical endpoint” (Aronson, 2005). Surrogate end-points facilitate research as they are easy to implement and more cost-effective. On top of that, there are some ethical issues that allow the use of surrogate end-points only. For instance, in laboratory settings and physiological markers, blood pressure indicators may be used as a surrogate for stroke. Still, when the primary outcome is mortality, end-points cannot substitute the true end-points in the long term and more research is needed to confirm findings and benefits of treatment.
In general, the clinical trial is a complicated process, which is often marked by low recruitment rates, financial demands, and ethical regulations. Research is needed as new drugs, and alternative treatments can improve people’s well-being and save lives.
Outcome Measurements: Qualities to Consider
No matter what outcome measurements researchers choose, there are some essential qualities that all measures need to cover:
Validity:
All measurements need to reveal good validity. Validity can be explained as the degree to which a measurement is valid and strong in what it claims to measure. For instance, intelligence tests need to measure intelligence, not memory, in order to be valid.
- Face validity is one of the essential types of validity. As the name suggests, face validity describes if a measurement is at face value and assesses what appears to be measured. In clinical settings, face validity shows if the outcome measures identify important symptoms and changes.
- Content validity or logical/rational validity shows if a measurement manages to measure every facet of a theoretical construct. In medicine, content validity guarantees that measurement is relevant to the study and the illness in general.
- Criterion validity reveals how well a measurement can predict a health-related outcome and correlates with other research measures.
- Construct validity can be defined as the extent to which a test measures the construct it’s supposed to test.
Reliability & Repeatability:
Reliability or the consistency of measurement is also crucial. The repeatability of measurement is essential because good test-retest reliability can avoid variability. In other words, the same test given to the same people in a short period should show the same results. The between-observer agreement should also be sought. Note that between-observer agreement or inter-rater reliability reveals the consensus between different raters.
Errors:
Errors should be diminished by good outcome measurements. There are three types of systematic errors (Peat, 2011):
- Subject errors are systematic errors related to subjects’ bias.
- Observer errors are due to differences in observers and the administering and interpreting of a test.
- Instrument errors are caused by the instrument itself. Thus, tools should be accurate and calibrated according to a standard (certain temperature, for example).
Responsiveness:
Responsiveness is another crucial quality (Tarrant et al., 2014). It measures the efficacy and effectiveness of an intervention and the extent to which the service quality meets clients’ needs. Measurements should be responsive to within-subject changes. Some tools cannot detect small changes, and therefore, they cannot be used as primary outcomes. For example, measurements, such as a 5-point scale in which the symptom frequency is categorized as ‘constant,’ ‘frequent,’ ‘occasional,’ ‘rare’ or ‘never,’ are not responsive; such tools cannot detect subtle changes in symptoms. On top of that, experts should consider not only physiological changes but the quality of life.
Sample Size:
The statistical power of a test is another factor to consider when choosing outcome measurements (Peat, 2011). In general, the sample size needs to be adequate in order to show clinical and statistical differences between groups. Often, studies focus only on primary outcomes, but in practice, a broad range of outcomes should be implemented in research.
Sample size and types of variables go together. Here we should mention that categorical values, for instance, need bigger samples. A categorical or nominal variable has two or more categories (without any order). Gender is a categorical variable with two categories (male and female), and there is no intrinsic ordering of the categories. Ordinal values, on the other hand, are similar but there’s order in the categories. Note that the interval of the categories and their order is inconsistent, though. Economic status – with low, medium, and high categories – is an example of ordinal values. On the other hand, interval values have also categories placed in order but with equal spacing in between. Income of $5,000, $10,000, and $15,000 is a good example, as the size of that interval is the same ($5,000). Last but not the least, continuously distributed measurements, such as blood pressure, are vital (“What is the difference between categorical, ordinal and interval variables?” 2017).
To sum up, choosing the outcome measurements is a serious step that lies in the path to success of any health-related study. Because outcome measurements matter!
References
- Aronson, J. (2005). Biomarkers and surrogate endpoints. British Journal of Clinical Pharmacology, 59(5), p.491-494.
- Explanatory Variable & Response Variable: Simple Definition and Uses (2015, February 16). Retrieved from http://www.statisticshowto.com/explanatory-variable/
- Peat, J. (2011). Choosing the Measurements. Health Science Research, SAGE Publications, Ltd.
- Tarrant, C., Angell, E., Baker, R., Boulton, M., Freeman, G., Wilkie, P., Kackson, P., Wobi, F., & Ketley, D. (2014). Responsiveness of primary care services: development of a patient-report measure – qualitative study and initial quantitative pilot testing. Health Services and Delivery Research, No. 2, 46.
- Tyler, K., Normand, S., & Horton, N. (2011). The Use and Abuse of Multiple Outcomes in Randomized Controlled Depression Trials. Contemporary Clinical Trials, 32(2), p. 299-304.
- What is the difference between categorical, ordinal, and interval variables? (2017). Retrieved from https://stats.idre.ucla.edu/other/mult-pkg/whatstat/what-is-the-difference-between-categorical-ordinal-and-interval-variables/
See more of Our Posts
DIGITAL HEALTH CATEGORY
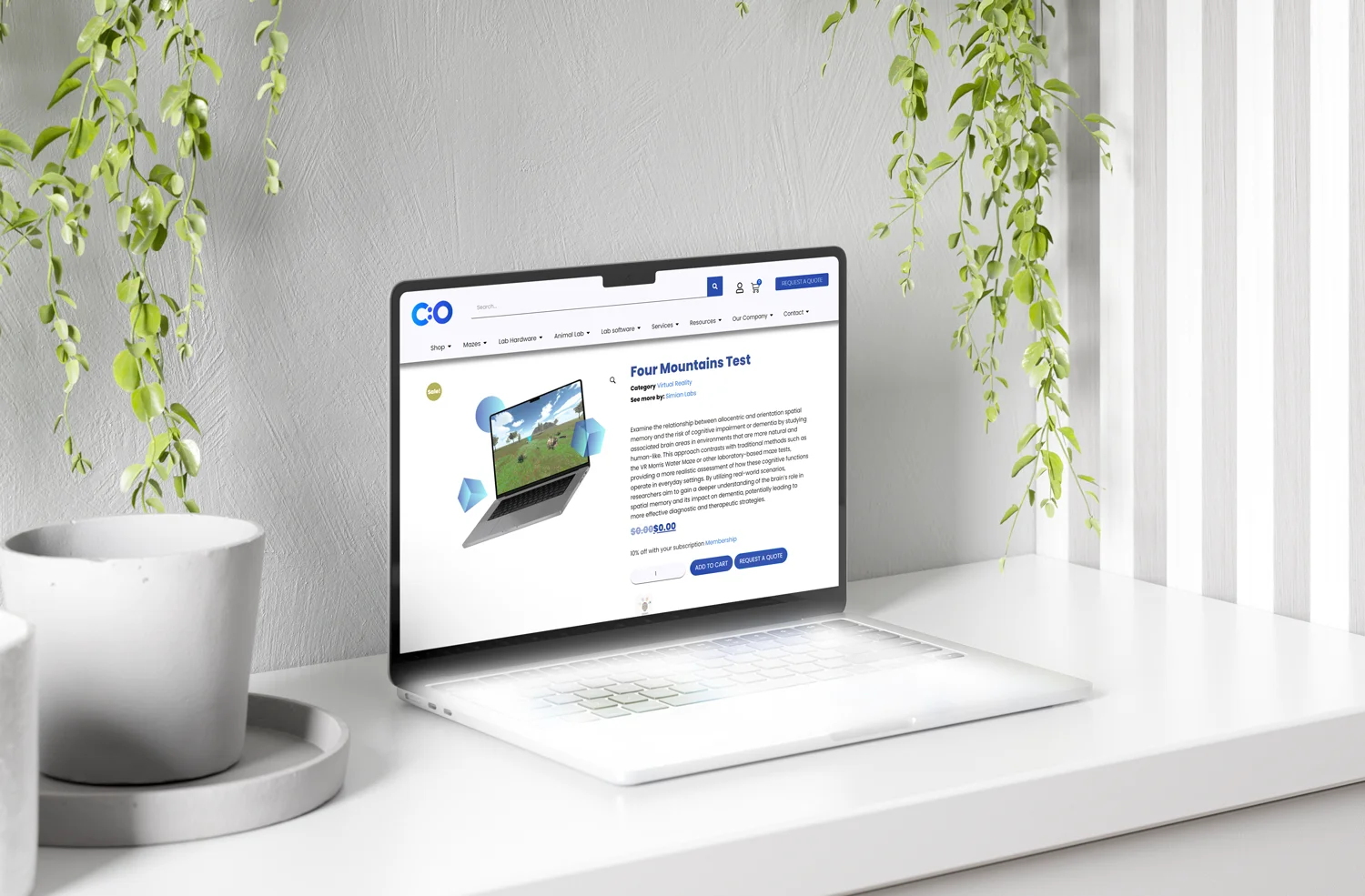
History and Early Tests Leading to the Four Mountains Test
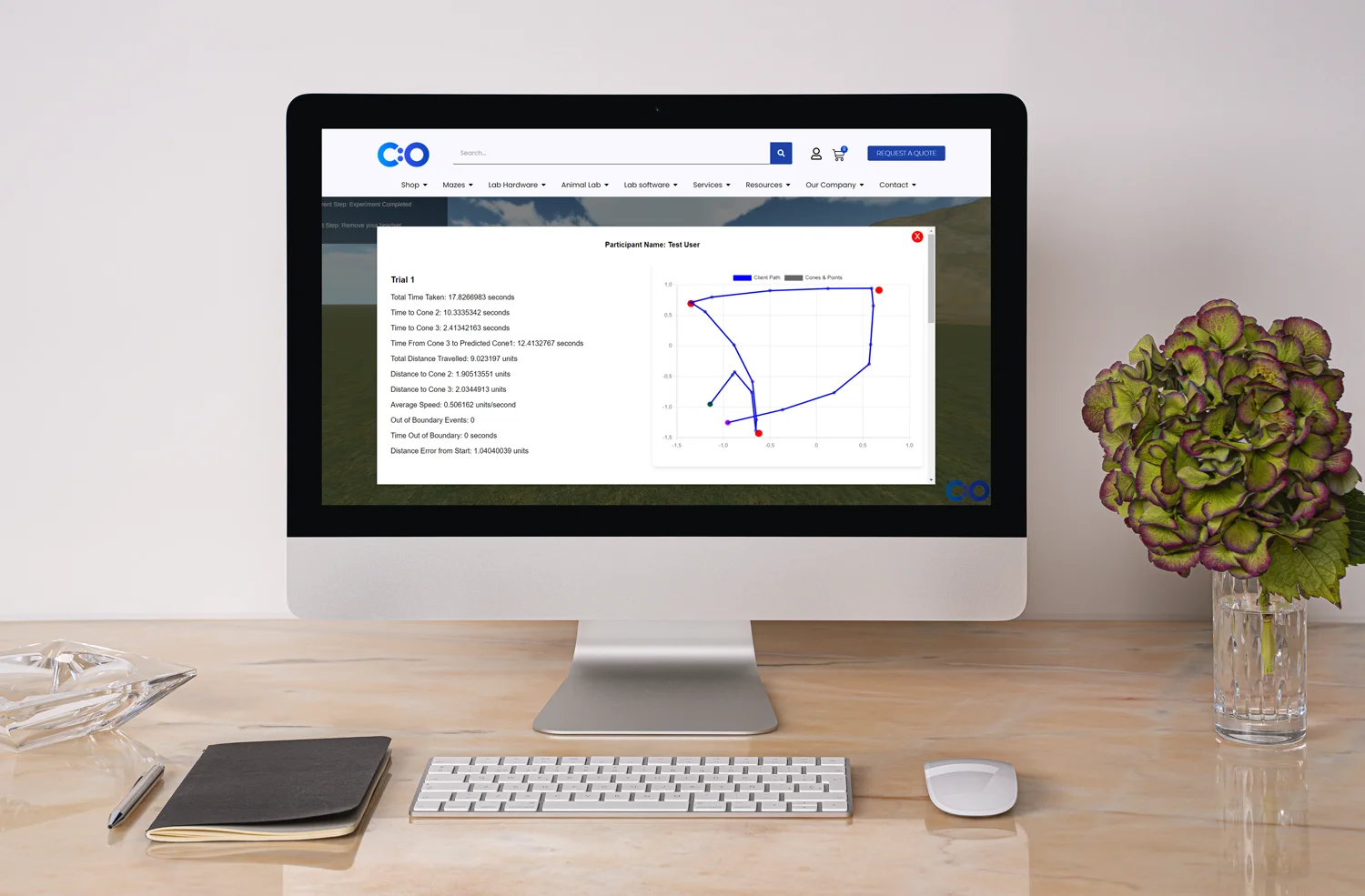
Analyzing Data from the Four Mountains Test
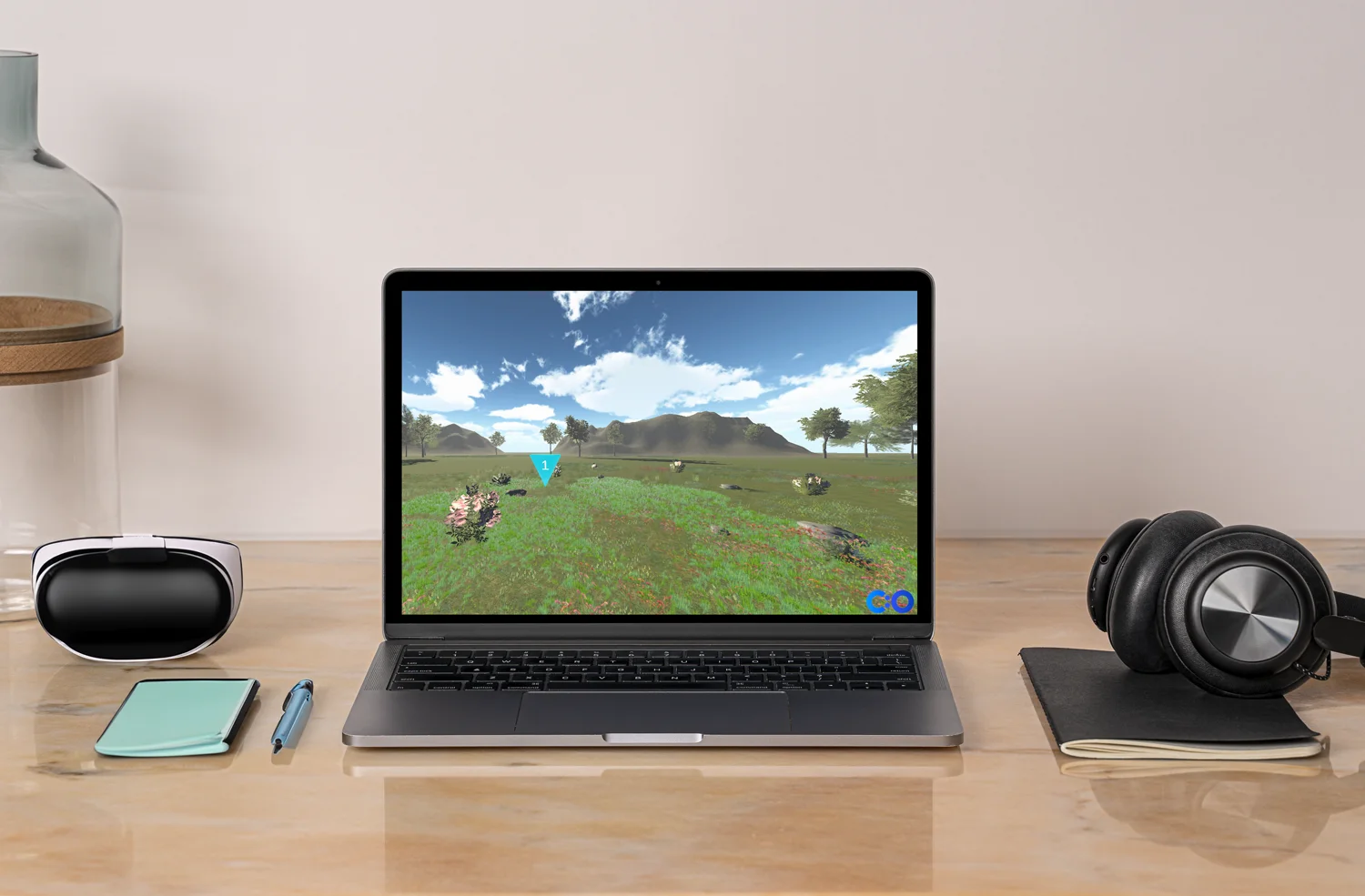
Understanding the Four Mountains Test: A Scientific Exploration
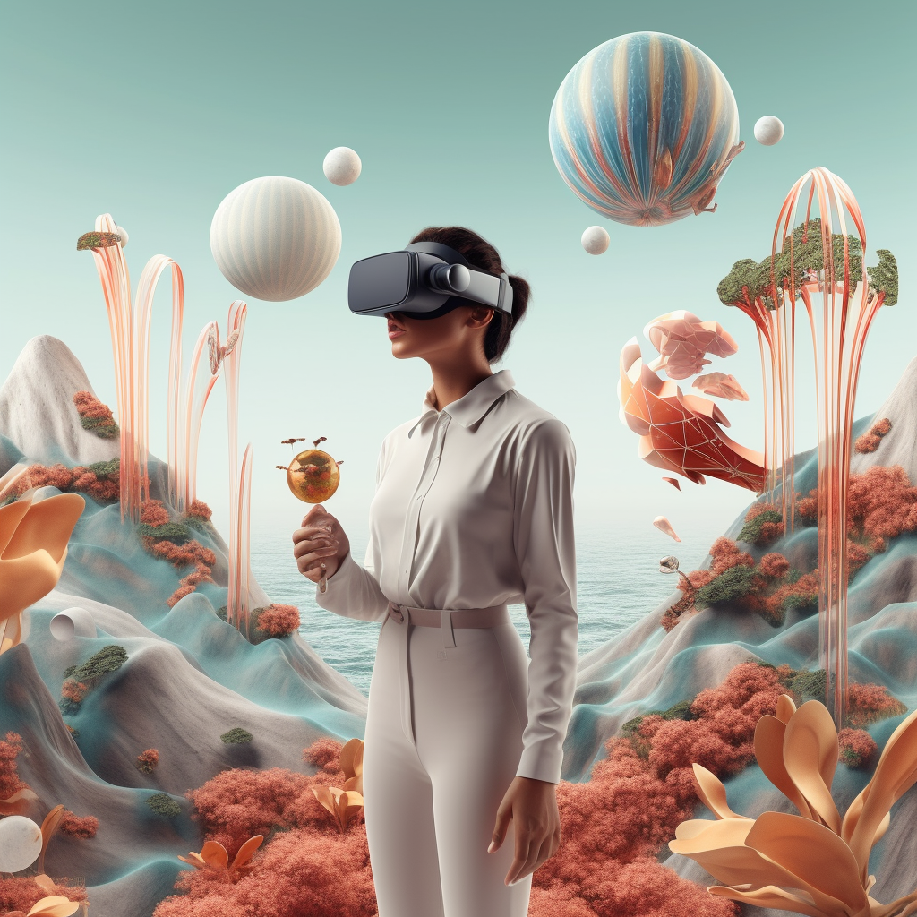
Virtual Reality Science: Exploring the Boundaries of Scientific Discovery
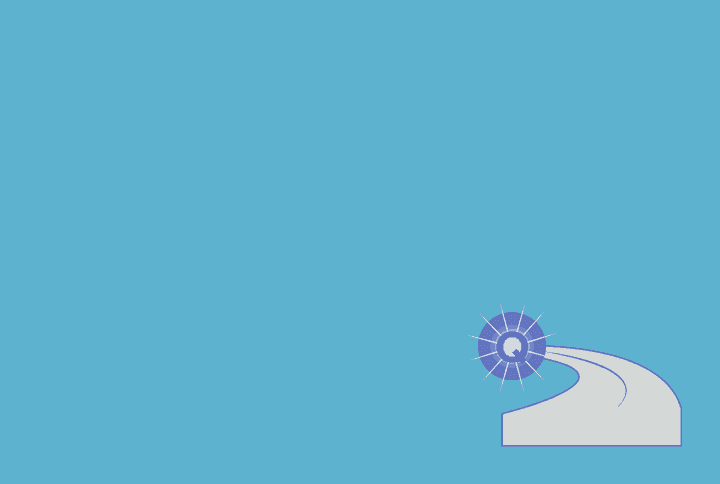
FHIR Claim Response
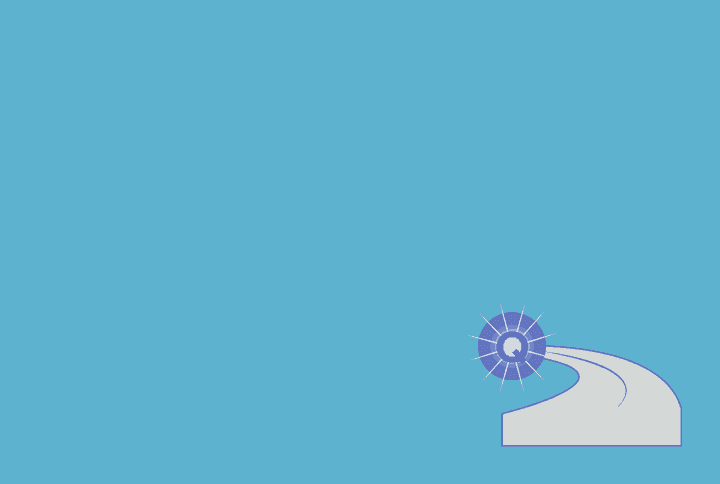
Corsi Block Test
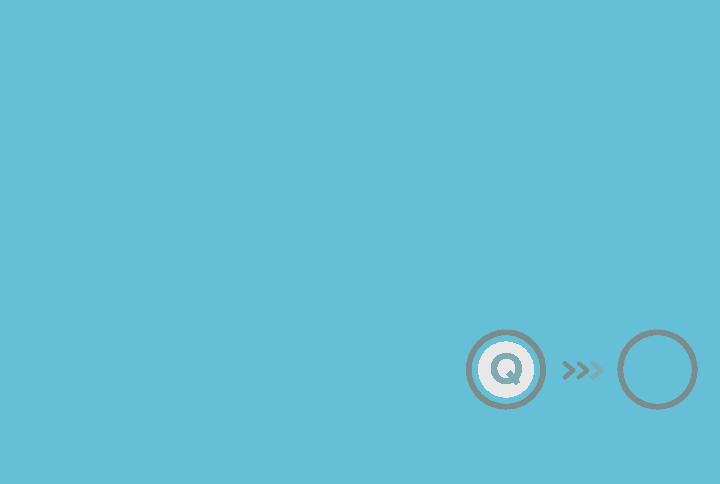
9-Hole Peg

What Adoption of Remote Patient Monitoring will mean for Healthcare Delivery

Digital Health Research: A Guide
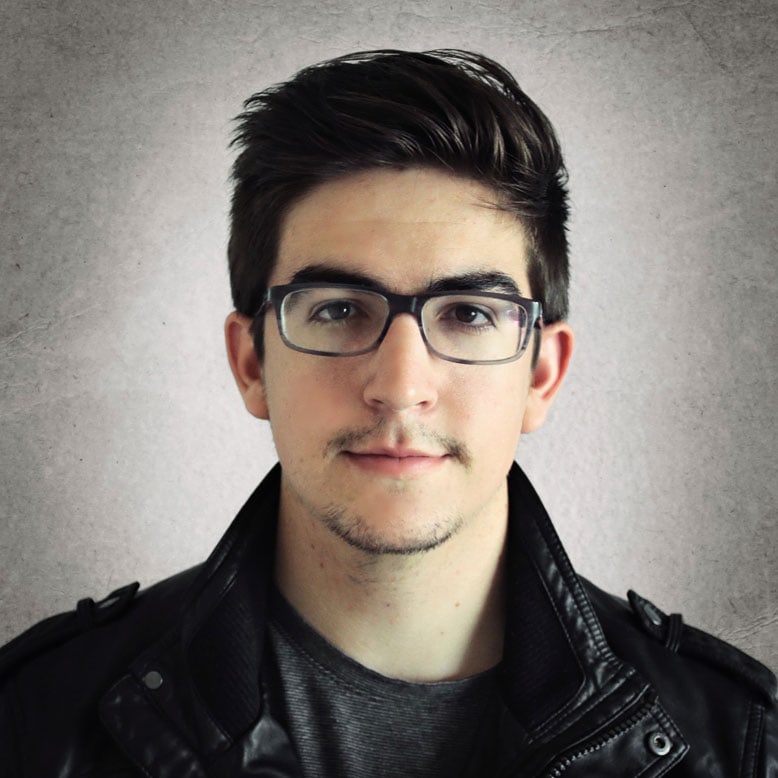
Alex Wormuth: Data Saves Lives
Never miss our posts!
Author:
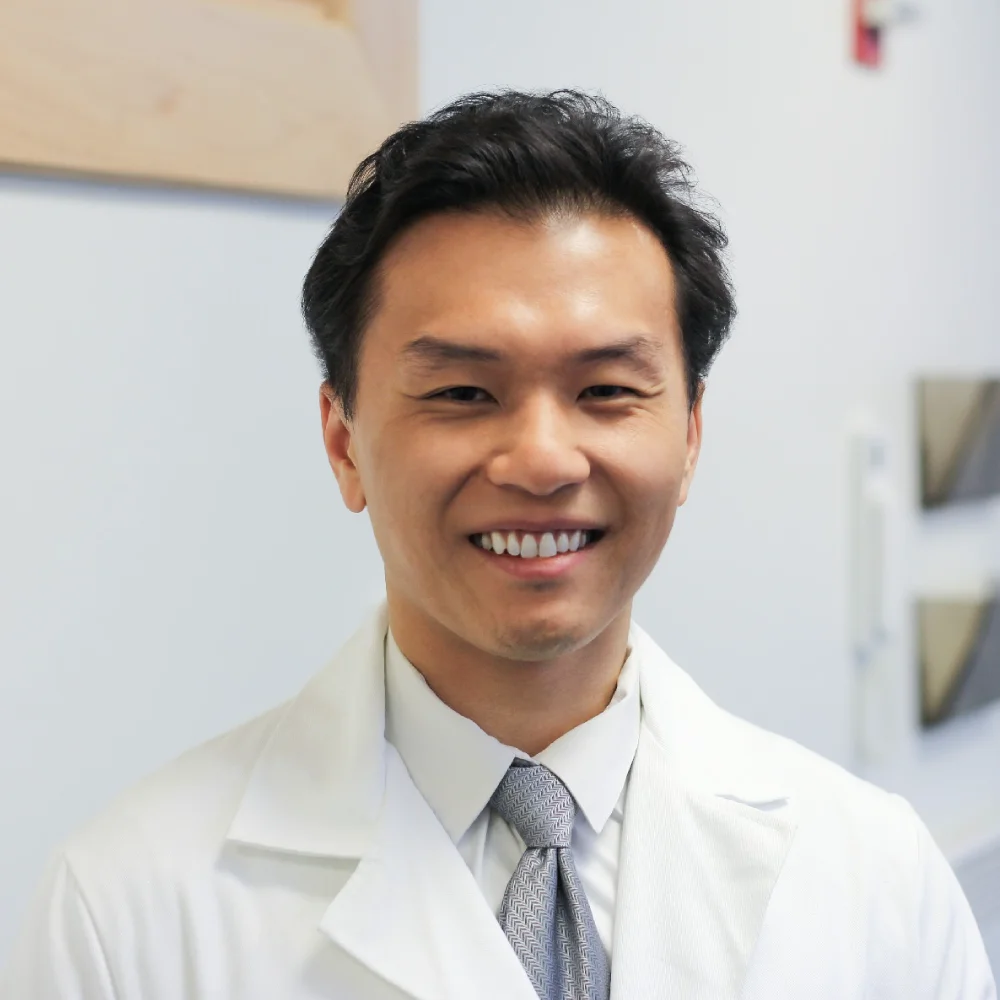
Shuhan He. MD
Shuhan He, MD is a dual-board certified physician with expertise in Emergency Medicine and Clinical Informatics. Dr. He works at the Laboratory of Computer Science, clinically in the Department of Emergency Medicine and Instructor of Medicine at Harvard Medical School. He serves as the Program Director of Healthcare Data Analytics at MGHIHP. Dr. He has interests at the intersection of acute care and computer science, utilizing algorithmic approaches to systems with a focus on large actionable data and Bayesian interpretation. Committed to making a positive impact in the field of healthcare through the use of cutting-edge technology and data analytics.