Introduction
Total Time Active during a fear conditioning experiment refers to the cumulative period an animal is considered “active” within the testing environment. This is defined as any movement that surpasses a predefined threshold of significance, excluding minor adjustments or minimal movements like twitching, grooming, or head bobbing.
In fear conditioning, freezing refers to when the animal remains motionless apart from respiratory movements and is a key sign of fear. ConductVision tracks both movement and freezing, giving a clear view of the animal’s activity during the experiment. It’s important to note that Total Time Active is essentially the complement of Freezing Duration, and these two metrics together provide a full picture of the animal’s movement behavior during the experiment.
Fear Conditioning Experiment
In a typical fear conditioning experiment, animals are exposed to a neutral stimulus (e.g., a sound tone or light) followed by an aversive event (such as a mild foot shock). Through repeated pairings, animals learn to connect the stimulus with the aversive event, leading to freezing responses. As previously mentioned, Freezing demonstrates a subject’s fear, while Total Time Active measures movement, often indicating exploratory behaviors and lower fear or anxiety levels.
ConductVision uses advanced algorithms and high-precision video tracking to detect and quantify these movements in real-time. The following illustrates how Total Time Active is captured and processed within ConductVision:
- Activity Detection: The software is programmed to detect meaningful animal movement based on movement thresholds, velocity, and displacement within the arena. Activity is only logged when these movements surpass the threshold, ensuring that small, involuntary movements aren’t misclassified as active behavior. This threshold can be adjusted by researchers to fine-tune the sensitivity of detection for specific experiments.
- Movement Threshold Settings: Researchers can set a threshold for what counts as “active movement,” such as speed (e.g., more than 1 cm/sec) or how far the animal moves. ConductVision tracks movements above this threshold as activity, while anything below is considered minimal or freezing behavior.
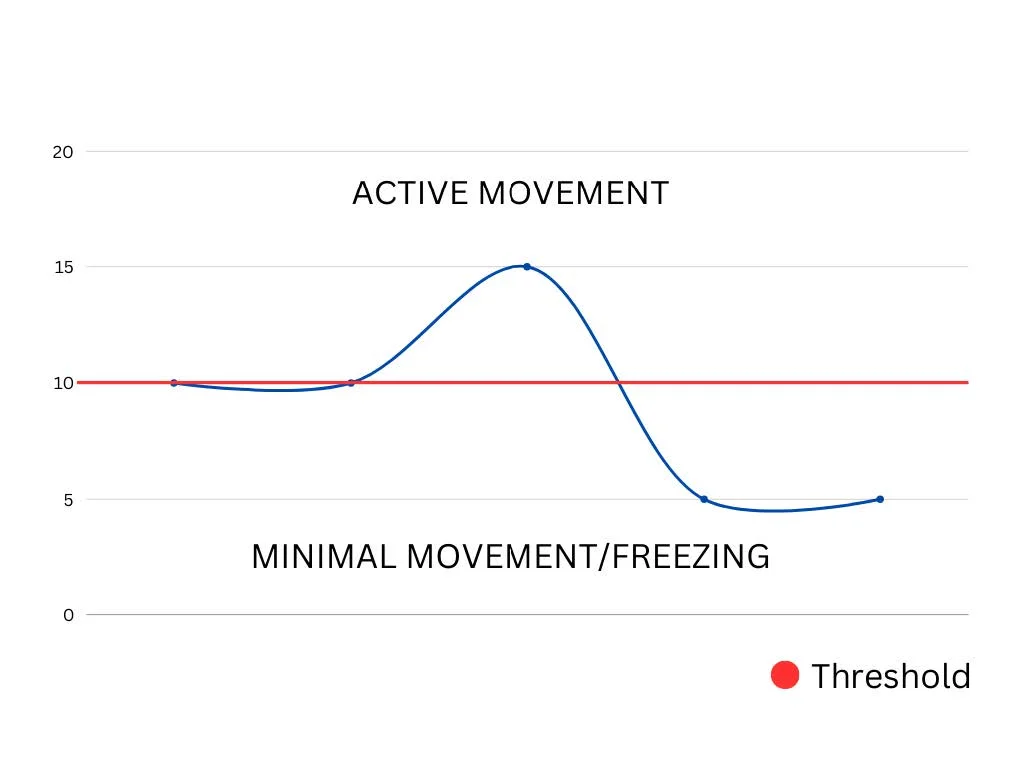
Figure: Researchers can set a threshold to define what qualifies as active movement. Any movement above this threshold (red line) is recorded as active behavior, while anything below is categorized as minimal movement or freezing. Created by Vanja Antonijevic at Conduct Science.
- Positional Tracking: ConductVision uses high-resolution video and smart tracking to monitor the animal’s position in the arena. It calculates the animal’s path, speed, and movement. When the animal moves, the software records it as “active time.” This data helps researchers understand the animal’s exploration and reactions to stimuli.
- Freezing Detection: ConductVision has a dedicated freezing detection algorithm that identifies when the animal is motionless. If movement falls below a specific threshold (for example, near-zero motion for a sustained period of 0.5 seconds or longer), this is categorized as freezing. This allows researchers to assess conditioned fear responses with precision.
- Summing Active Time: Throughout the session, the software sums all periods when the animal is considered “active.” This enables an understanding of how much the animal moved during the experiment, providing insights into its behavior in response to the fear-conditioning stimuli.
- Sensitivity Calibration: ConductVision also allows researchers to calibrate the sensitivity of movement detection, enabling the system to capture either broad or fine-scale movements. This flexibility is critical for various experimental designs, whether the goal is to detect subtle changes in posture or large-scale exploratory behavior. Adjusting the sensitivity helps define what counts as “activity” versus freezing, tailoring the analysis to the specific needs of the study.
See more of Our Posts
Video tracking and animal behavior
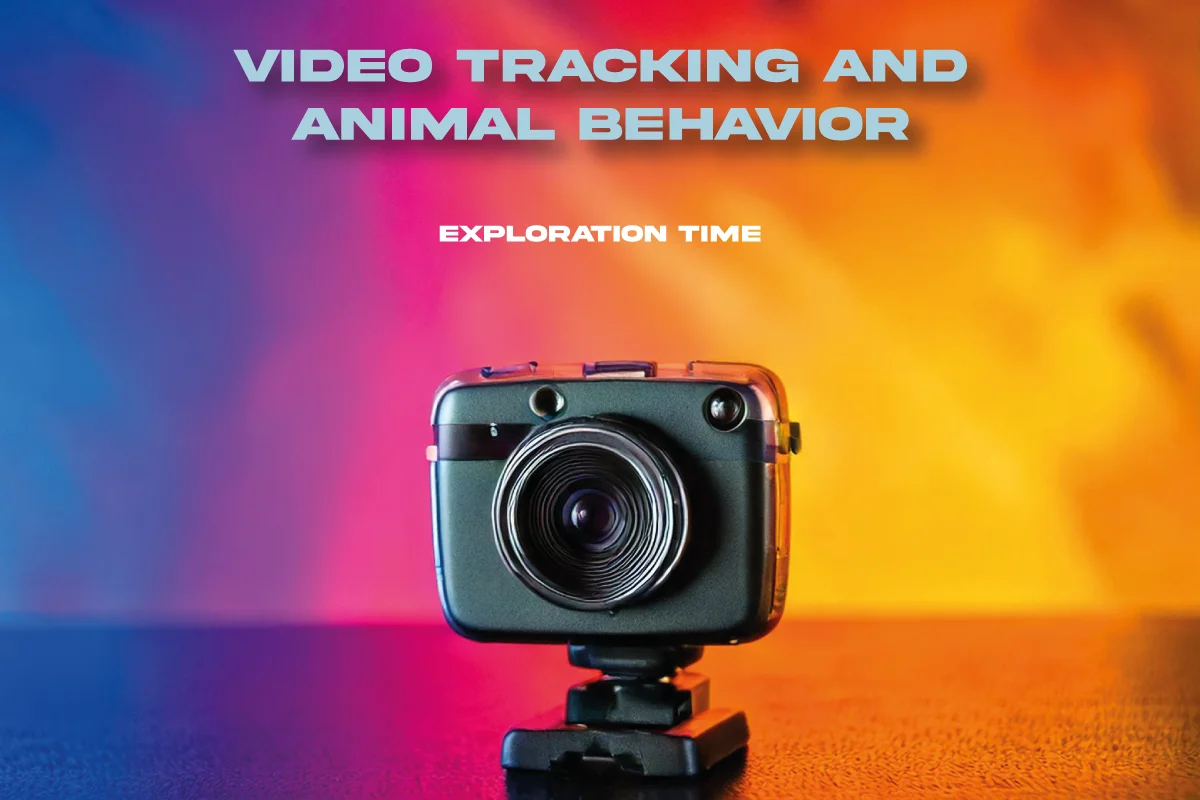
Exploration time
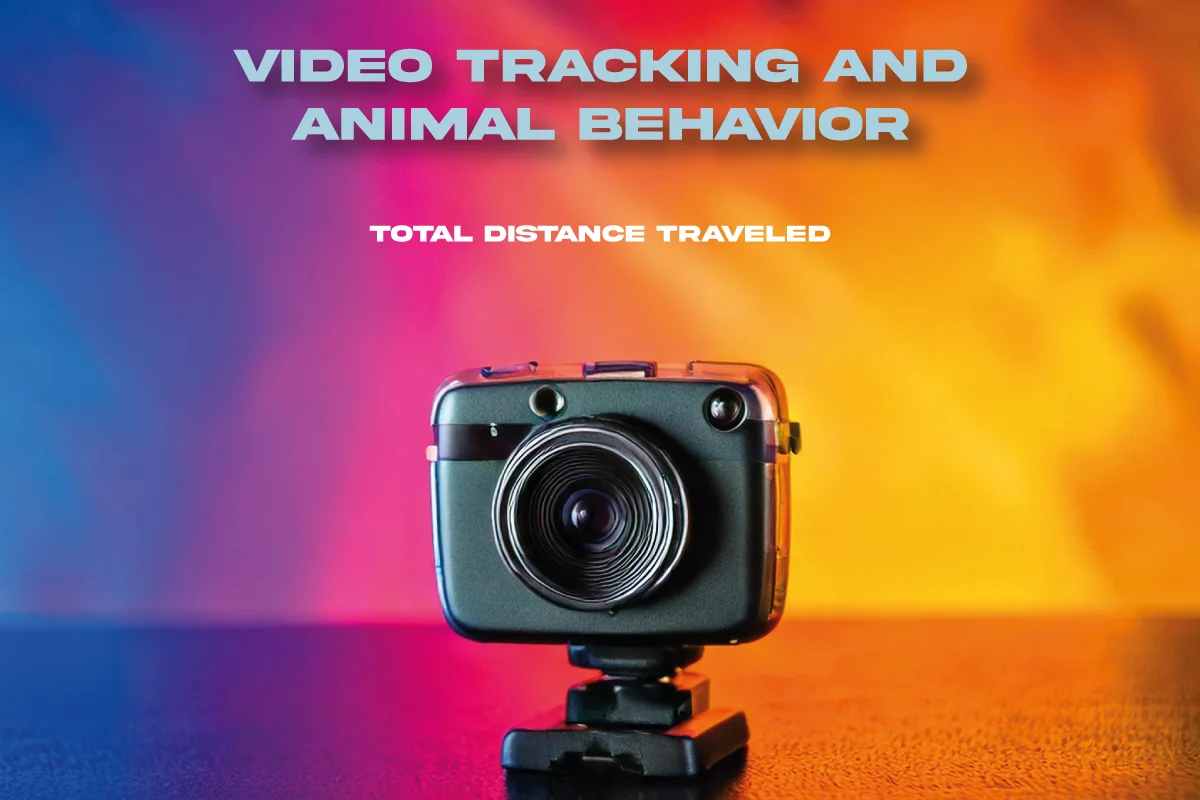
Total Distance Traveled
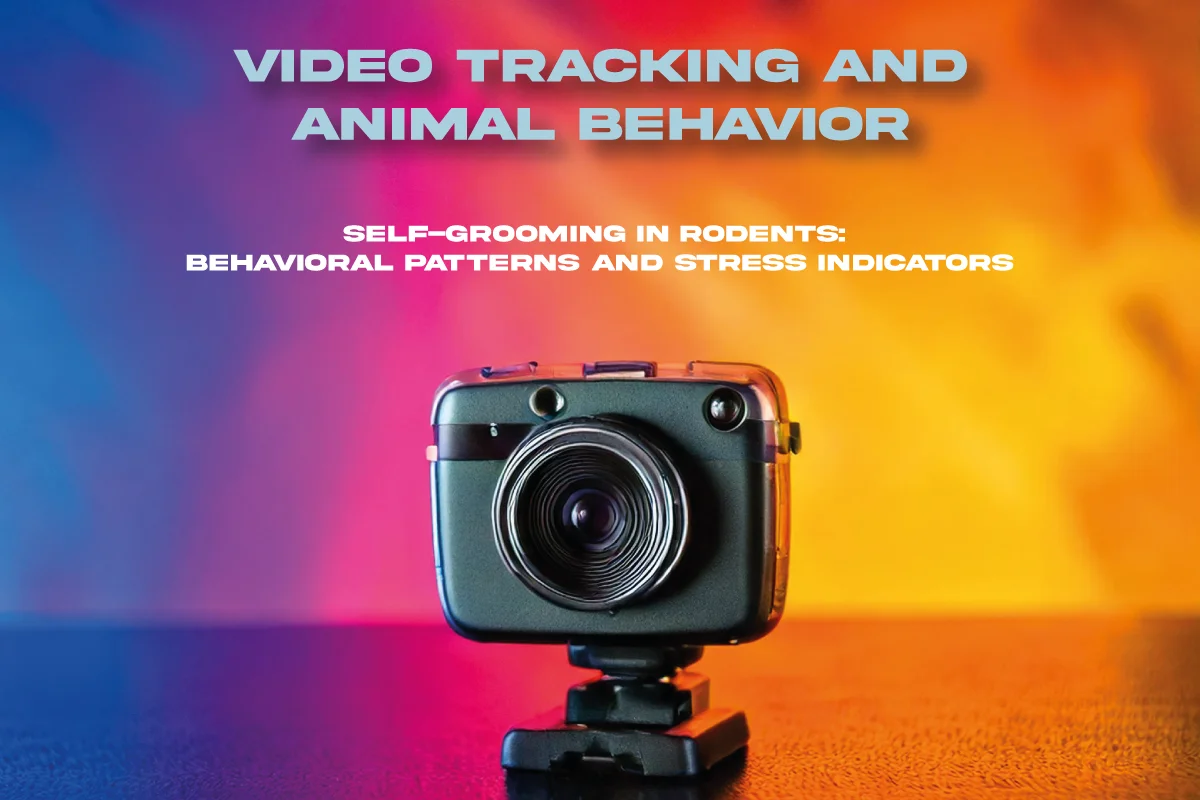
Self-Grooming in Rodents: Behavioral Patterns and Stress Indicators
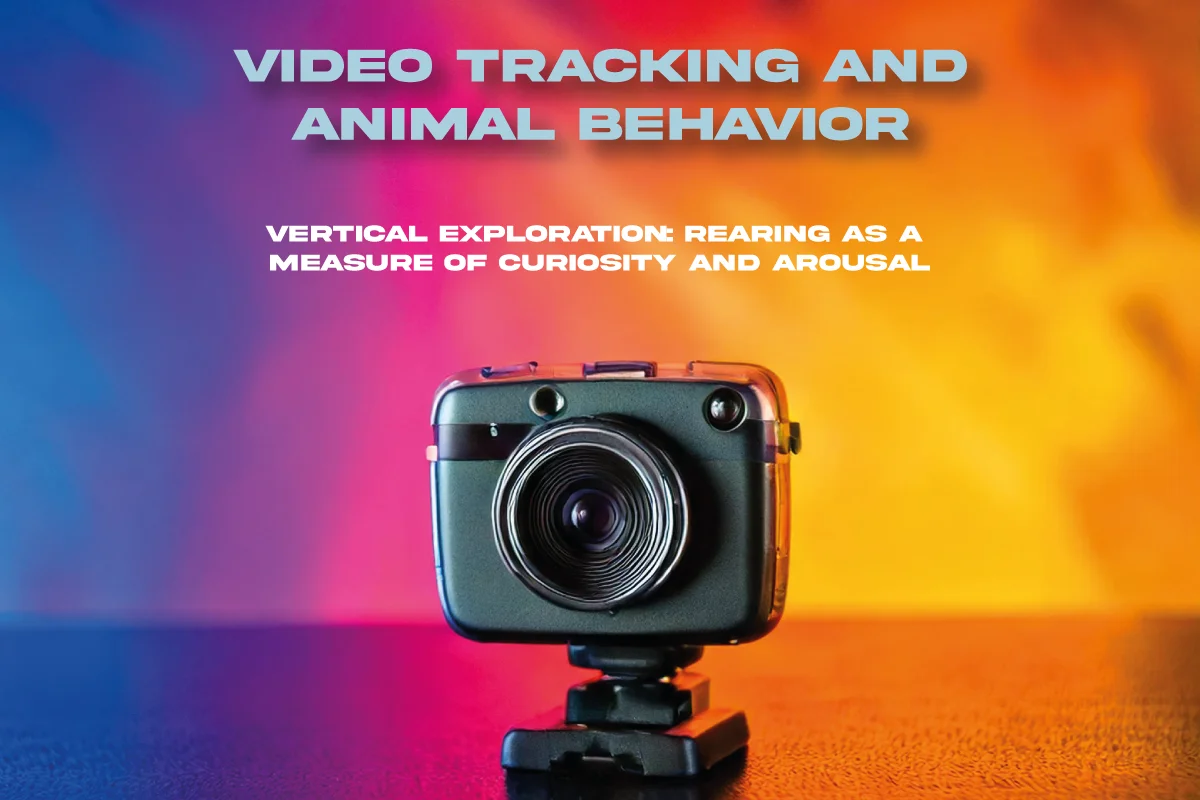
Vertical Exploration: Rearing as a Measure of Curiosity and Arousal

Short- and Long-Term Locomotor Activity Analysis Using Video Tracking Software: A Guide for Neuroscientists

Unlocking the Complexity of Leptin-Deficiency Behavioral Studies Using Computer Vision Tracking
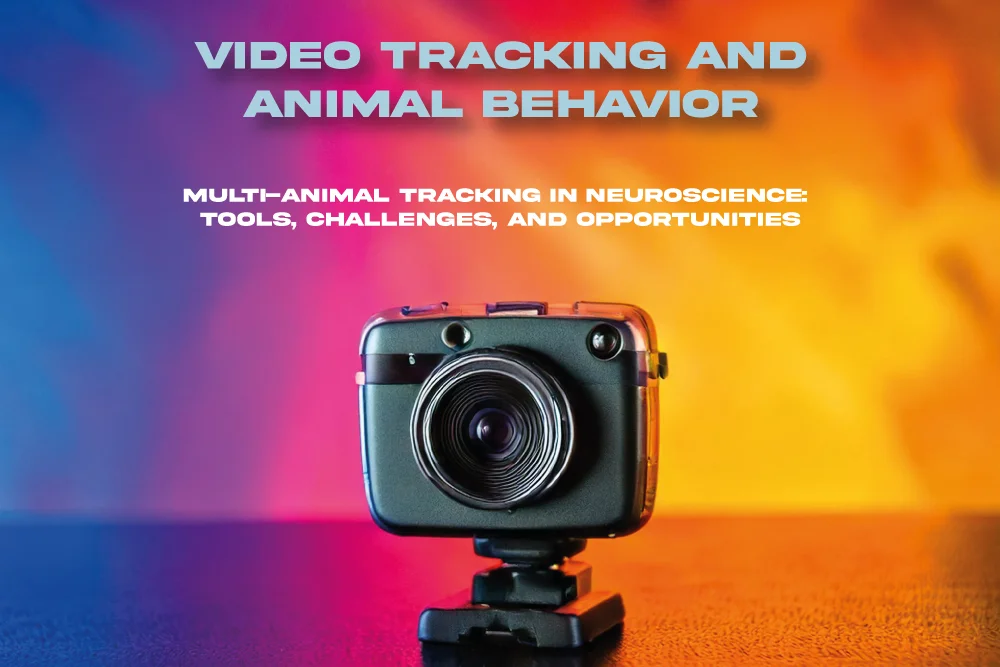
Multi-Animal Tracking in Neuroscience: Tools, Challenges, and Opportunities
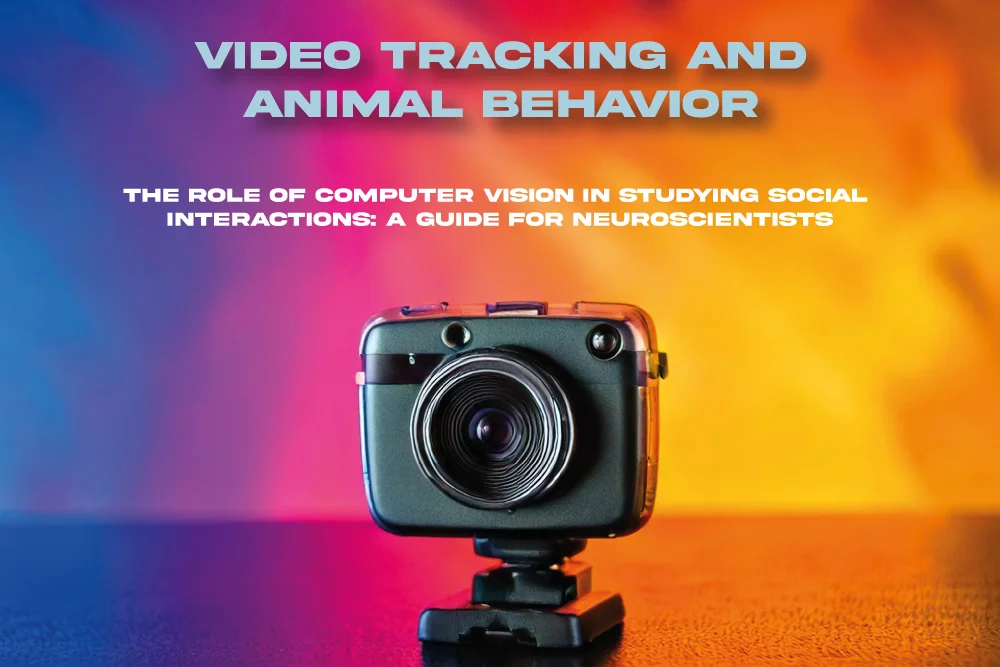
The Role of Computer Vision in Studying Social Interactions: A Guide for Neuroscientists
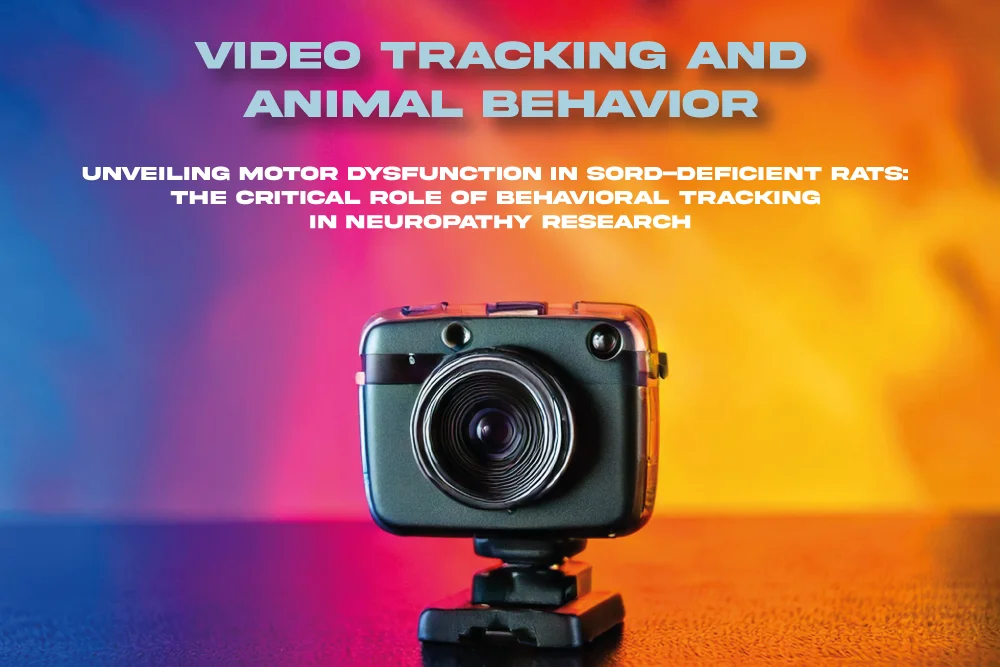
Unveiling Motor Dysfunction in SORD-Deficient Rats: The Critical Role of Behavioral Tracking in Neuropathy Research
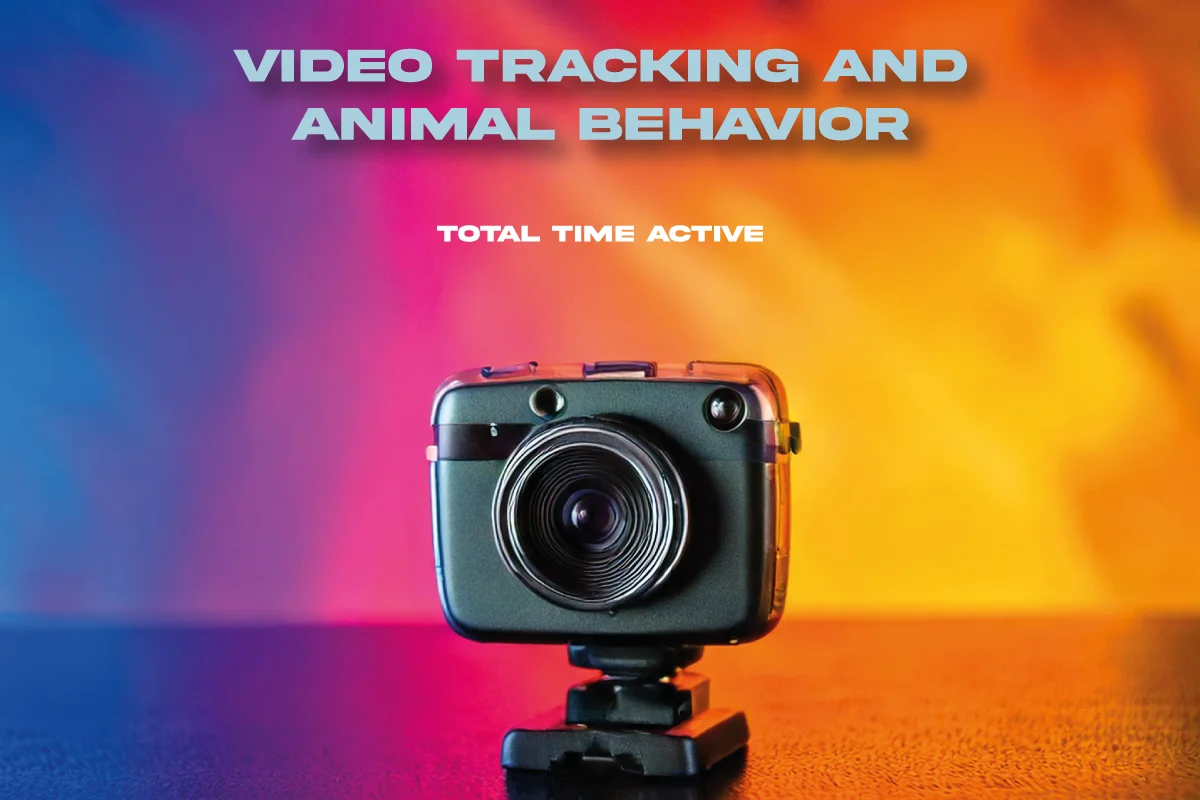
Total Time Active
All Resources
Never miss our posts!
Author:
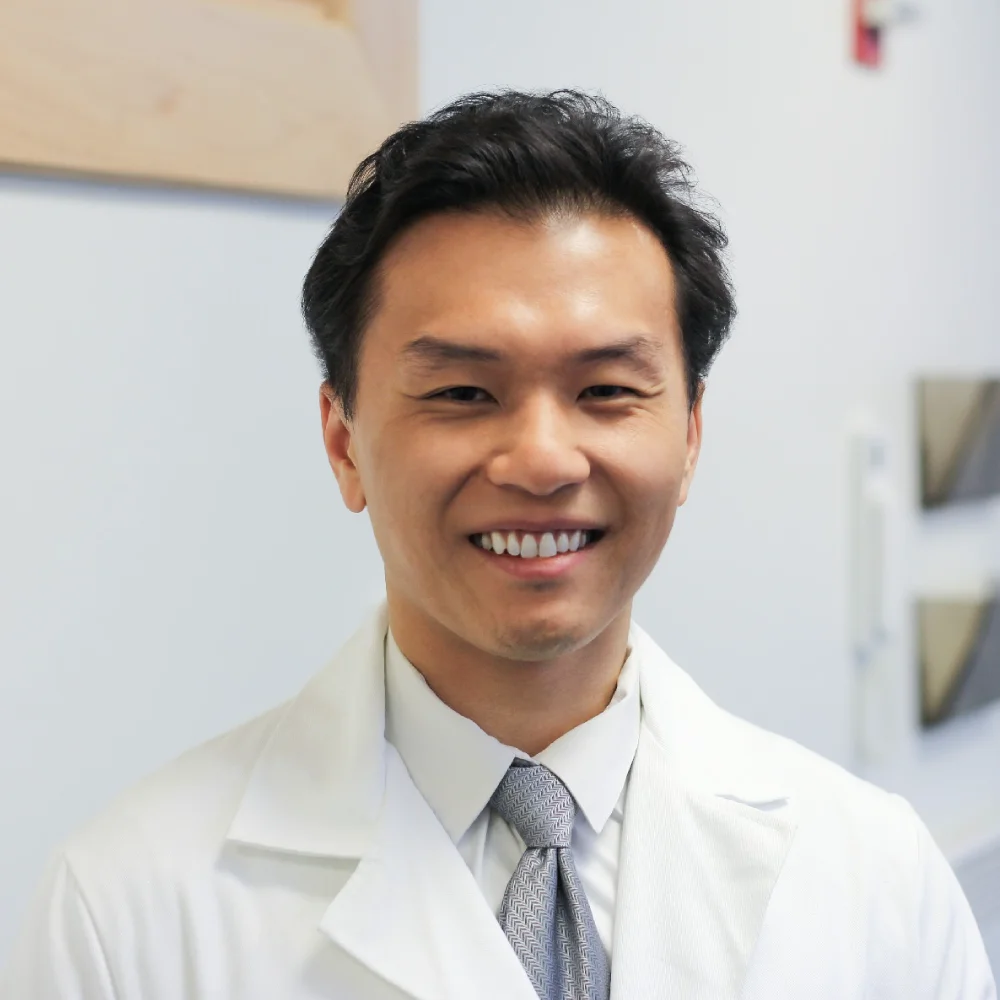
Shuhan He, MD
Shuhan He, MD is a dual-board certified physician with expertise in Emergency Medicine and Clinical Informatics. Dr. He works at the Laboratory of Computer Science, clinically in the Department of Emergency Medicine and Instructor of Medicine at Harvard Medical School. He serves as the Program Director of Healthcare Data Analytics at MGHIHP. Dr. He has interests at the intersection of acute care and computer science, utilizing algorithmic approaches to systems with a focus on large actionable data and Bayesian interpretation. Committed to making a positive impact in the field of healthcare through the use of cutting-edge technology and data analytics.