Data Is the Core of Research
Big data is the core of medical research. Since clinical studies face strict protocols, unpredictable delays, and pressure for low costs, sponsors and contract research organizations (CRO) need robust data to succeed. Synthesized and abundant medical information can help researchers deal with the complexity and demands of today’s medical research.
Clinical trials rely on multifaceted datasets and clinical trial performance analytics to improve drug development, health outcomes, and revenue. Clinical trial performance metrics provide information across systems to track execution, manage logistics, and detect risks across multiple sites and regions. To be more precise, performance metrics can be defined as discrete units of research information, which can be employed to benefit internal and operational performance, as well as interoperability. Performance analytics can reshape the future of research.
Usage and Benefits of Clinical Trial Performance Analytics
With leveraging health technologies, clinical trials need robust data and sophisticated analytics to flourish (Simpao et al., 2014). It’s not surprising that researchers have started to adopt analytics and performance indicators to analyze and visualize clinical trial data. Note that in research, the employment of analytics is defined as the systematic use of medical information, as well as its quantitative and qualitative analysis. Analytics can boost quality performance, risk assessment, decision-making, resource allocation, and relationships with sponsors. Visual analytics, for instance, is an essential part of clinical research and site selection.
Interestingly, experts state that today’s performance analytics differ from traditional operational metrics and standard key performance indicators. While standard indicators show what factors need to be assessed (e.g., low enrollment), performance analytics reveal the actual cause of a problem (e.g., strict inclusion criteria). Valuable clinical trial performance metrics, in particular, are data points which incorporate quality assessment and predictive modeling techniques. They provide insights into operational performance and quality. Note that other essential factors that boost internal operations and relationships with sponsors include benchmarking, high-quality management, and competitor research. It’s no surprise that sophisticated clinical trial management systems (CTMS) platforms and heat maps have started to adopt clinical trial performance metrics to improve medical research and site performance.
Types of Clinical Trial Performance Metrics
Clinical trial performance metrics, as explained earlier, reveal numerous benefits over traditional indicators. Performance metrics are vital in quality assessment, site selection, and risk performance. Such metrics can be used not only to assess a clinical trial but also to improve outcomes and support action plans. Therefore, sponsors must decide on a strict set of metrics and goals of assessment. Note that generally speaking, there are two types of metrics that can help researchers measure risks and performance: standardized metrics (e.g., site quality performance) and study-specific indicators. Also, clinical trial performance metrics can be categorized into three groups: start-up, maintenance, and close-out metrics.
Start-up metrics: Start-up metrics may include investigator recruitment, regulatory protocols, the cycle time for received and finalized budget, vendor selection, first patient first visit (FPFV), cycle time to site activated, and approval rate.
Maintenance metrics: During the actual trial, vital maintenance metrics involve site quality, consent forms, timelines/deadlines for reports, meeting criteria, randomization methods, privacy, and trial master file/documentation.
Close-out metrics: The close-out metrics tackle aspects, such as last patient last visit (LPLV), analysis and errors, and time to closure of sites.
Although each study has its peculiarities, usually short cycle times across different metrics (e.g., budget, protocols) indicate site and staff professionalism and effectiveness. To set an example, since institutional review board (IRB) approval is vital, a site which approves new trials and has good records for this data point may be preferred by sponsors and external organizations. After all, clinical trial performance metrics should support good clinical practice, internal performance, and relationships with sponsors.
High-Quality Clinical Sites and Performance Metrics
Prior to the commencement of a clinical trial, sponsors and researchers must consider multiple factors, possibilities, and obstacles. Site selection is one of the most crucial aspects of digital health research. Sites vary from universities to independent medical institutions. Note that clinical trials with multiple sites and across various regions are more likely to face errors and bias. Site performance is fundamental (Whitham et al., 2018). Sites that reveal short cycle times, enroll subjects on a regular basis, and document high compliance in accordance to protocols usually lead to high-quality data and success.
Interestingly, after a systematic literature review, a Delphi study identified 117 performance metrics across 21 studies (Whitham et al., 2018). 30 metrics were excluded due to unclarity or specific requirements. 32 experts in three focus groups identified 28 meaningful metrics and categorized them into four domains (recruitment and retention, data quality, protocol compliance, and staff). These metrics were used to create an online Delphi survey; 211 respondents were invited. The final round of the study consisted of a consensus meeting, during which the research team concluded the following eight metrics divided into three domains:
Recruitment and retention: Recruitment of participants is one of the most challenging aspects of research. Therefore, recruitment and retention metrics are vital. Actual recruitment versus target recruitment is an important indicator. If the number of participants recruited into the study by the site of interest is high, the site is effective. Another vital metric is the percentage of participants who have consented. The third indicator in this category is the number of randomized subjects who have withdrawn their consent (to any further participation or follow-up procedures).
Data quality: Multiple aspects, such as sample size and study design, affect data quality. Hence, data quality is essential for patient well-being and marketing success. A vital metric is the percentage of randomized subjects (at the site of interest) with a query which concerns primary outcome data. Randomization is essential, so the percentage of randomized participants with complete data for primary and important secondary outcomes should be analyzed as well. The third metric in this group is the number of adverse events (at least one) per number of randomized subjects (at the particular research site).
Protocol compliance: Regulations and ethical consideration are vital in research, with patients being the core of digital health. Therefore, a crucial metric related to protocol compliance is the percentage of participants with at least one protocol violation. Last but not least, researchers must assess the percentage of randomized participants who started allocated intervention as defined in the protocol.
It’s interesting to mention that in research, it’s accepted that sites with a good historical track record will reveal future high-quality performance. Sites that enroll and maintain subjects are considered reliable. Nevertheless, metrics, such as protocol complexity and excessive lab tests, can also affect interpretations and future results. Interpretations, predictions, and generalizations should be based on robust data. For instance, if research teams report positive outcomes in their cohorts, but not in the general population, sponsors should not make conclusions about site quality.
We should mention that a recent study showed that operational data from central lab services could be a powerful indicator of site performance. Such data can be used to improve operational planning and quality (Yang et al., 2018). Yang and colleagues used metadata about laboratory kit shipments to clinical sites (e.g., shipment dates) to reconstruct past performance and provide insights into the operational performance of other sites. As a result, the research team created a set of metrics from over 14,000 protocols, 1400 indications, 230,000 investigators, and 23 million patient visits. Note that Yang and colleagues (2018) concluded that country and regional trends are vital, particularly in the study of Alzheimer’s disease.
Clinical Trial Performance Analytics and Interactive Data Visualization Methods
Performance analytics and visualization methods go hand in hand. Visualization techniques can help experts understand performance indicators and optimize site networks. Visualizations that represent historical enrollment data may include tree-maps, color-coded charts, and enrollment targets. One of the main benefits of data visualization is the fact that findings are clear and easy to analyze. Thus, medical specialists and personnel without IT and data mining knowledge can easily find patterns and mitigate risks.
What’s more, visuals improve communication and interoperability. Complex ideas can be communicated in real time and with precision. Simulation techniques, dimensional modeling, and interactive maps help researchers present large numbers of data – something that standard tabular models cannot accomplish. Visual analytics in health care can enable data exploration, generation of hypotheses, recruitment, data quality, and study management. Such methods can apply not only to clinical trials, but a wide range of fields, such as genomics, diseases surveillance, and clinical decision support. In addition, user dynamic interfaces can present data in detail and allow comparisons of different designs and protocols. Interactive dashboards and single plots can allow analysis of historical data, predictions of future performance, and trends (Yang et al., 2018).
Performance Analytics in Practice: The Future of Research
In medical research, planning is the key to success. From literature reviews to protocols, planning can benefit the employment of big data and performance metrics. For instance, one of the main goals of digital health research and drug development is to cut costs and accelerate drug authorization. While well-defined, standardized performance metrics can benefit clinical trials, each study imposes certain peculiarities and complexity, so experts should set meaningful data points and goals. To assess the progress of a clinical trial, meaningful metrics must measure variance, bring new insights, and lead to change.
Most of all, performance analytics and analysis should lead to action plans. As explained above, data varies between sites, clinical trials, and over time. If a metric shows that a given action has not been beneficial, experts should reassess their methods and change them. Reassessment of metrics is also crucial to identify weaknesses. Such reviews should be done on a regular basis. Note that another factor which affects performance analytics is reproducibility. Reproducibility can help experts verify results for different operations and across different time periods. At the same time, clinical trial performance metrics should compare universal benchmarks in order to assess areas of competitiveness and marketing advantages. Since drug development is a costly business, with a huge percentage of drugs failing to reach the market, planning and performance analytics becomes crucial. Most of all, performance analytics must be employed to improve patient outcomes. As patients are the focus of digital health research, sponsors, researchers, and regulatory bodies must find a balance between costs and benefits – with the sole purpose of improving drug development and patient well-being.
Conclusion
Clinical research is a complex topic, with health technologies (e.g., BYOD approach) influencing clinical trials and business strategies. Performance analytics have the potential to improve clinical trials, internal and operational performance, and relationships with sponsors. Analytics can be used to track operations, boost outcomes, and mitigate risks. Clinical trial performance metrics, in particular, are vital data points which can provide information about research sites and performance. With standardized and site-specific metrics, researchers can assess historical site records and predict future outcomes. By implementing quality assessment, predictive modeling, and visualization methods, researchers can reduce costs and speed up market authorization. In fact, visuals can provide valuable insights into the connection between sites, regions, and hypotheses. Perhaps one of the most valuable aspects of clinical trials performance analytics is their potential to trigger real changes and improve health outcomes.
By planning and establishing useful metrics, sponsors can evaluate sites and improve research. In the end, big data, performance analytics methods, and health technologies are reshaping the future of drug development and clinical trials. Medical data can bring clinical research a step closer to success.
References
- Simpao, A., Ahumada, L., & Galvez, J. (2014). A Review of Analytics and Clinical Informatics in Health Care. A Review of Analytics and Clinical Informatics in Health Care. Journal of Medical Systems, p. 38-45.
- Whitham, D., Turzanski, J., Bradshaw, L., Clarke, M., Culliford, L., Duley, L., Shaw, L., Skea, Z., Treweek, S., Walker, K., Williamson, P., & Montgomery, A. (2018). Development of a standardised set of metrics for monitoring site performance in multicentre randomised trials: a Delphi study. Trials.
- Yang, E., O’Donovan, C., Phillips, J., Atkinson, L., Ghosh, K., & Agrafiotis, D. (2018). Quantifying and visualizing site performance in clinical trials. Contemporary Clinical Trials Communications, 9, p. 108-114.
See more of Our Posts
DIGITAL HEALTH CATEGORY
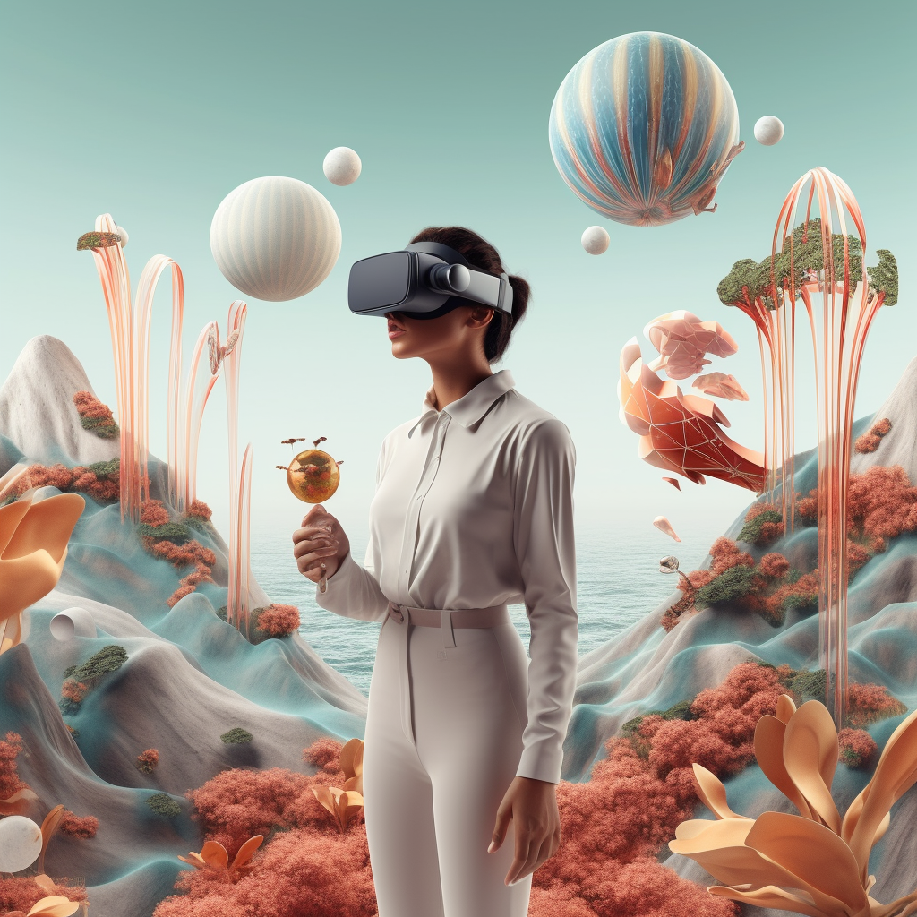
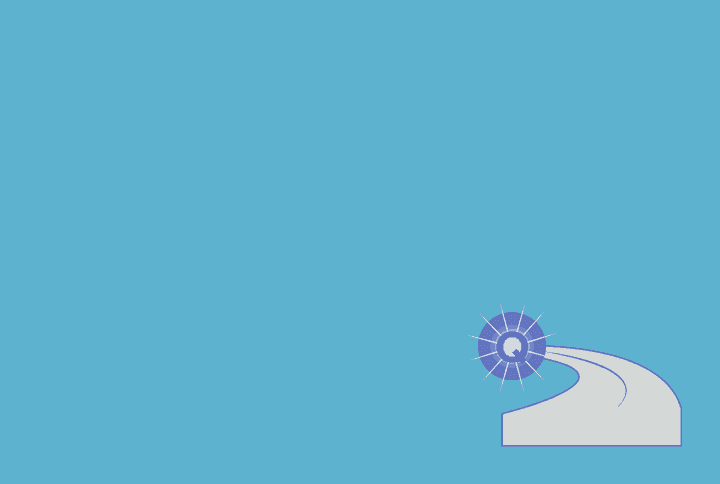
FHIR Claim Response
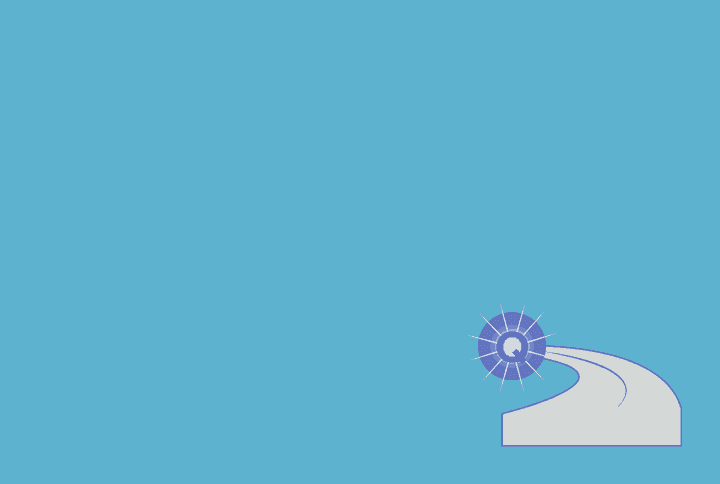
Corsi Block Test
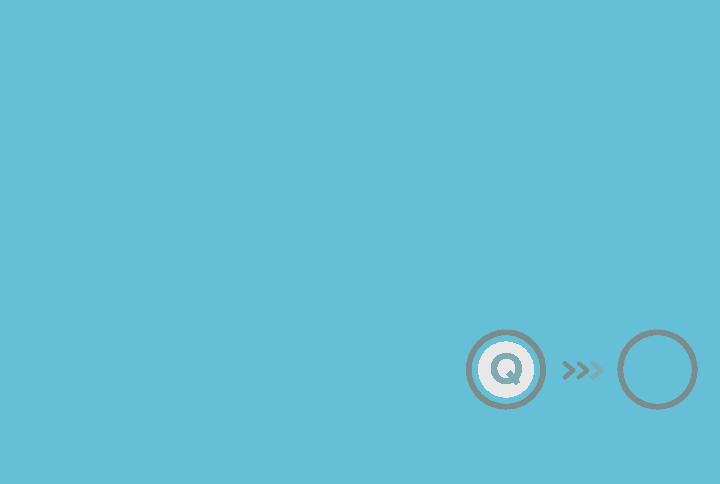
9-Hole Peg


Digital Health Research: A Guide
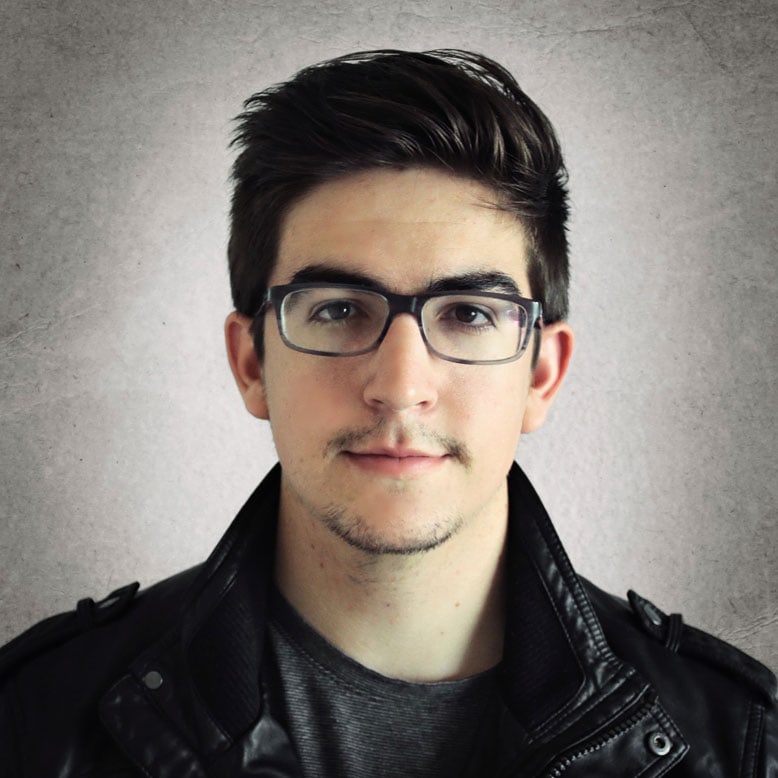
Alex Wormuth: Data Saves Lives
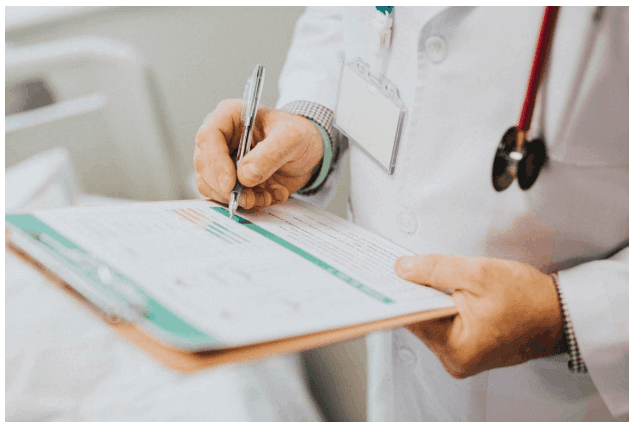
Digital Transformation in the Healthcare Industry: 5 Key Trends

mHealth App Development: Current Practices and Future Perspectives

Schizophrenia in mHealth
All Resources
Never miss our posts!
Author:

Louise Corscadden, PhD
Dr Louise Corscadden acts as Conduct Science’s Director of Science and Development and Academic Technology Transfer. Her background is in genetics, microbiology, neuroscience, and climate chemistry.